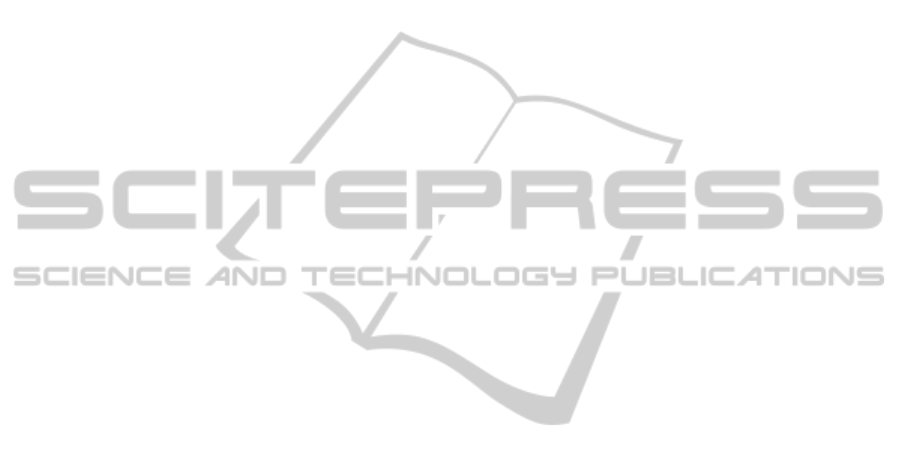
6 CONCLUSIONS
This paper presents new approaches to face
templates creation. Proposed methods were designed
for real-world face recognition systems with large
number of users in order to enhance face recognition
system performance and to accelerate the
classification process. The proposed methods
produce one, two or three templates per person
which reduces template database access time,
classification time and redundancy among person's
templates. This results in a more efficient
recognition process. The impact of different face
template creation methods has been examined on
two databases, the PubFig database and the IFaViD
database.
Firstly the tested face recognition system and its
key algorithms have been described in detail.
Subsequently the examined methods for face
template creation have been described. Face
template examination and optimization is a novel
step in face recognition research. Remarkable results
have been obtained despite the fact that the proposed
approaches to face template creation are only the
first step in our research.
The next section has described the IFaViD and
the PubFig testing and training databases and briefly
summarizes their properties.
Finally the test results have been presented in the
last section. The most remarkable finding is that
different methods for face template creation have a
significant influence on system recognition
performance. The next finding is that there may be
differences in recognition performance when
different one-face template methods are used. The
centroid approach outperforms the rest of one-face
template methods. Moreover it does not require any
extra setup, just like the medoid template method.
The multiple template methods have outperformed
all other methods and seem to offer a promising
approach as they enable complex cluster
representation.
ACKNOWLEDGEMENTS
Research described in the paper is financially
supported by the Ministry of industry and trade of
Czech Republic under grant IVECS, No.
FR-TI3/170 and by Ministry of Education, Youth
and Sports and European Structural Funds under
grant SIX, No CZ.1.05/2.1.00/03.0072.
REFERENCES
Ahonen, A., Hadid, A. & Pietikäinen, M. (2006) Face
Description with Local Binary Patterns: Application
to Face Recognition. In Pattern Analysis and Machine
Intelligence, IEEE Transactions on, vol.28, no.12,
pp 2037-2041.
Bambuch, P., Malach, T., & Malach, J. (2012) Video
database for face recognition. In Technical Computing
Bratislava 2012. Bratislava, 7
th
November 2012.
Bratislava: RT Systems, s.r.o & Systémy Priemyslovej
Informatiky s.r.o. pp 12.
Kumar, N., Berg, A. C., Belhumeur, P. N. & Nayar, S. K.
(2009) Attribute and Simile Classifiers for Face
Verification. In 12
th
International Conference on
Computer Vision 2009. Kyoto, 29
th
September – 2
nd
October 2009. pp. 365-372.
Malach, T., Bambuch, P. & Malach, J. (2012). Face
Detection in video sequences. In Radioelektronika
2012. Brno, 17
th
-18
th
April 2012. Brno: Department
of Radio Electronics, Brno University of Technology.
pp 228-292.
Muja, M. & Lowe, G. D. (2009) Fast approximate nearest
neighbors with automatic algorithm configuration. In
Conference on Computer Vision Theory and
Applications. Lisboa, 5
th
to 8
th
February 2009. Lisboa:
INSTICC Press. pp. 331-340.
Prinosil, J. (2013) Local Descriptors Based Face
Recognition Engine for Video Surveillance Systems.
In 36th International conference on
telecommunications and signal processing. Rome, 2
nd
to 4
th
July 2013.
Stallkamp, J., Ekenel, H. K. & Stiefelhagen, R. (2007)
Video-based Face Recognition on Real-World Data. In
IEEE 11th International Conference on Computer
Vision. Rio de Janeiro, 14
th
to 21
st
October 2007. pp:
1-8.
Viola, P. & Jones, M. (2001) Robust Real-Time Object
Detection. International Journal of Computer Vision.
Zhao, W. & Chellappa R. (2006) Face Processing:
advanced modeling and methods. Academic Press.
FaceTemplatesCreationforSurveillanceFaceRecognitionSystem
729