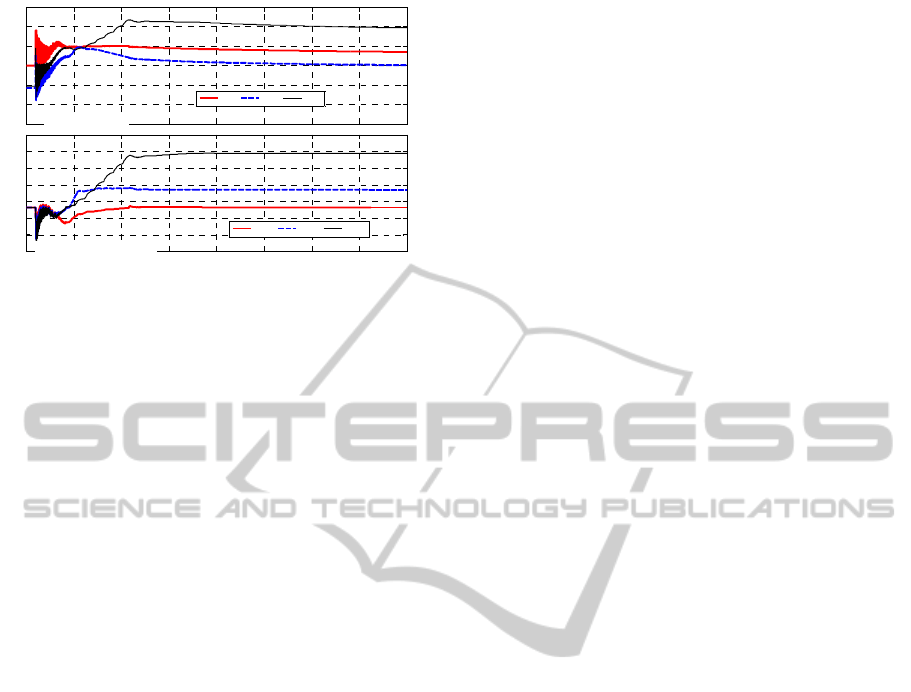
Figure 8: The system stator current and stator voltage
profiles under the MACS control.
The local automation reduces the AVR setting. GAs
at busses 202 and 203 begin to increase reactive
power output until the generator excitation currents
reach their near-critical values. Collaborative actions
of GAs and LAs are allowed to unload G301-303
(Fig. 9).
As a result, the system voltage profile improves,
as can be seen in Fig. 7, and the Kohonen network
does not detect any deterioration at t=130.63 s. From
t=206 s, the Kohonen network identifies the normal
state, however, the alarm 3 state is also still activated
because the system is still in the normalization of
post-emergency state.
Thus, as a result of the MACS control actions,
the subsystem can maintain its stability without load
shedding via coordinating available sources of
reactive power.
5 CONCLUSIONS
This paper proposes a neural multi-agent-based
approach to the system monitoring and control with
the goal of identifying potential voltage instability
problems before they lead to major blackouts. The
proposed MACS structure is hierarchical; it consists
of the top-level agent, middle-level agents and low-
level agents. Under normal operating conditions, the
MACS operates in a competitive mode; low-level
agents exchange information with other agents to
maintain their local conditions within specified
limits and to maximize profits of their respective
companies. An alarm state triggers a collaborative
mode in which the agents coordinate their actions to
prevent a system blackout.
REFERENCES
IEEE PES PSDP Task Force on Blackout experience,
mitigation, and role of new technologies, blackout
experiences and lessons, Best practices for system
dynamic performance, and the role of new
technologies, IEEE Special Publication 07TP190, July
2007.
Wang X., Shao W., Vittal V., 2005. Adaptive corrective
control strategies for preventing power system
blackouts, in Proc. of 15
th
PSCC, Liege.
Lehnhoff S., Häger U., Zimmermann T., Rehtanz C.,
2011. Autonomous distributed coordination of fast
power flow controllers in transmission networks, in
IEEE PES ISGT 2011 Europe, Manchester, UK.
Häger U., 2012. Agent-based real-time Coordination of
Power Flow Controllers, Dissertation, TU-Dortmund
University.
Panasetsky D.A., Voropai N.I., 2009. A multi-agent
approach to coordination of different emergency
control devices against voltage collapse. IEEE
Bucharest PowerTech.
Negnevitsky M., 2008. Computational Intelligence
Approach to Crisis Management in Power Systems,
Int. Journal of Aut. and Control, 2 (2/3), 247-273.
Kalyani S., Shanti Swarup K., 2012. Design of pattern
recognition system for static security assessment and
classification, Pattern Analysis & Applications, vol.
15, 299-311.
Voropai N., Kurbatsky V., Tomin N., Panasetsky D.
“Preventive and emergency control of intelligent
power systems,” In Pros. of PES ISGT Europe, Berlin.
Niebur D. 1994. Kohonen self-organizing neural network
for power system security assessment, Thesis #1244,
Lausanne, EPFL.
Negnevitsky M., Tomin N., Panasetsky D., Kurbatsky V.,
2013. Intelligent Approach for Preventing Large-Scale
Emergencies in Electric Power Systems, IEEE
PowerTech 2013, Grenoble, France.
Negnevitsky M., Voropai N., Kurbatsky V., Tomin N.,
Panasetsky D., 2013. Development of an Intelligent
System for Preventing Large-Scale Emergencies in
Power Systems, IEEE/PES GM, Vancouver, BC,
Canada.
IEEE PES CAMS Task Force on Understanding,
Prediction, Mitigation and Restoration of Cascading
Failures “Initial Review of Methods for Cascading
Failure Analysis in Elect. Power Trans. Syst.,” In
Proc. IEEE PES GM, Pittsburgh, PA USA July 2008.
0 50 100 150 200 250 300 350 400
1
1.02
1.04
1.06
1.08
1.1
1.12
1.14
t,сек.
U,отн.ед.
Напряжение Статора, отн.ед.
U
G
303 U
G
306 U
G
307
0 50 100 150 200 250 300 350 400
0.7
0.8
0.9
1
1.1
1.2
I,отн.ед.
Ток Статора, отн.ед.
I
G
303 I
G
306 I
G
307
ICAART2014-InternationalConferenceonAgentsandArtificialIntelligence
570