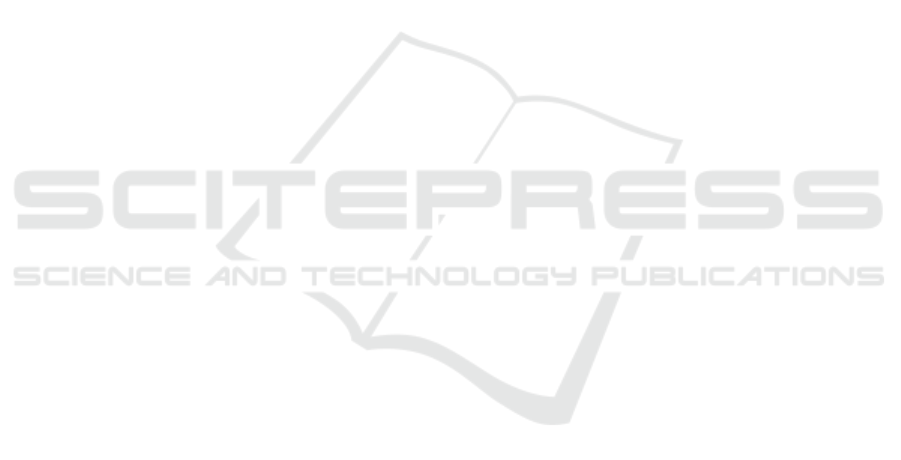
Neural Network for Fretting Wear Modeling
Laura Haviez
1,2,3
, Rosario Toscano
2
, Siegfried Fourvy
1
and Ghislain Yantio
3
1
LTDS, UMR 5513, Ecole Centrale de Lyon, Ecully, France
2
LTDS, UMR 5513, ENISE, Saint-Etienne, France
3
SAGEM, Boulogne-Billancourt Cedex, France
Keywords: Fretting Wear Modeling, Artificial Intelligence, Artificial Neural Networks.
Abstract: Materials wear is a very complex, only partially-formalized phenomenon involving numerous parameters
and damage mechanisms. The need to characterize wear in many industrial applications prompted the
present research. The study concerns an original strategy investigating the effect of contact conditions on
the wear behavior of carburized stainless steels under fretting and reciprocating sliding motion. A physical
model was constructed, and pre-treated experimental data were incorporated in a neural network to model
wear volume. Three models are proposed and compared, according to input.
1 INTRODUCTION
Wear is generally defined as loss of surface material
from contact surfaces subjected to relative motion.
Tribologic issue must therefore be taken into
consideration, and several models have been
developed in recent years (Kolodziejczyk, 2010;
Zhang, 2003). These models usually correlate wear
volume with physical and geometrical quantities
such as load, sliding distance, coefficient of friction,
hardness, materials (Anand Kumar, 2013; Genel,
2003; Sahraoui, 2004), and physical laws such as the
Archard wear criterion (Archard, 1953). Many
parameters influence wear. To identify one relevant
parameter, we chose a neural network to model
wear, creating an experimental database: the great
advantage of Artificial Neural Networks (ANNs) is
their ability to be used as an arbitrary function
approximation mechanism which ‘learns’ from
observed data. Fretting damage was used as a case
study. Small oscillatory movements may induce
interface fretting, shortening predicted lifetime. The
interface wear response was modeled and empirical
models were created based on data from fretting
tests. The Artificial Intelligence model was validated
against the physical description of fretting wear
behavior.
2 EXPERIMENTAL PROCEDURE
2.1 Material and Contact Type
Tests were performed on two chromium-
molybdenum stainless steels: one carburized
stainless steel (M1) and one stainless steel with mass
quenching (M2). The M1 specimen comprised 3
layers: the external layer was hard and decarburized
layer (white layer: WL); the second was the
carburized phase (CL), with hardness gradient
between 760 HV and 550HV (Figure 1a); the third
was the bulk, with 500 HV hardness. These
materials were studied to determine the wear
kinetics of a two cross-cylinder configuration.
According to Hertz, this configuration is equivalent
to a sphere/plane configuration where M1 is mobile
and M2 fixed. The two cylinders had the same
radius (7.5 mm) and the same length (20 mm). The
normal force was adjusted to reach 2,200 MPa
Hertzian maximum contact pressure. Surface
roughness was Ra=0.4µm for both materials.
2.2 Test System
Figure 1b shows a diagram of the fretting wear test.
An MTS hydraulic tension-compression machine
regulated displacement between cylinders (further
details of this setup and experimental method used
can be found in (Fouvry, 1996)). During the test,
normal force P was kept constant by a feedback
617
Haviez L., Toscano R., Fourvy S. and Yantio G..
Neural Network for Fretting Wear Modeling.
DOI: 10.5220/0004908506170621
In Proceedings of the 6th International Conference on Agents and Artificial Intelligence (ICAART-2014), pages 617-621
ISBN: 978-989-758-015-4
Copyright
c
2014 SCITEPRESS (Science and Technology Publications, Lda.)