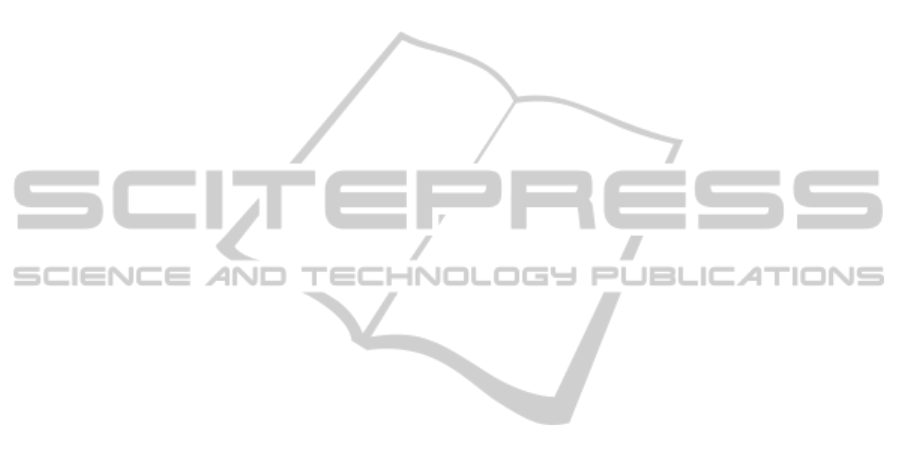
unpredictable; it depends on personnel turnover and
changes on medical guidelines. On the other hand, its
impact can be usually limited by exploiting EMs. The
comparison of classification techniques outlined that
ESA is able to achieve good performance in terms of
accuracy, this is probably due to the fact that it is able
to exploit the semantic hidden into syntactical struc-
tures, but it avoids to classify about the 30% of the
testing dataset; EMs have very good average accu-
racy, also on documents from the Radiotherapy De-
partments, which have very irregular structures. The
irregularity of documents is related to the lack of a
supporting environment that is able to provide “stan-
dard sentences” to physicians while they are generat-
ing clinical documents.
Future work include further improvements of
the ESA algorithm by exploiting automatic param-
eter configuration techniques (e.g., ParamILS (Hut-
ter et al., 2009)), since it uses several parameters
as thresholds, and a study of the impact of obsoles-
cence that helps engineers in designing the best pos-
sible training set giving a large set of clinical docu-
ments. Interesting literature is available about this is-
sue (Cano et al., 2006; Foody et al., 2006; S
´
anchez
et al., 2003) but a specific analysis considering the
peculiar features of the clinical domain is still miss-
ing. We are also interested in investigating a better ex-
ploitation of standard sentences techniques, in order
to improve the systems used by physicians for gener-
ating clinical reports, and for simplifying documents
classification.
REFERENCES
Breiman, L. and Breiman, L. (1996). Bagging predictors.
In Machine Learning, pages 123–140.
Cano, J. R., Herrera, F., and Lozano, M. (2006). On the
combination of evolutionary algorithms and stratified
strategies for training set selection in data mining.
Appl. Soft Comput., 6(3):323–332.
Chen, R. C. and Hsieh, C.-H. (2006). Web page classi-
fication based on a support vector machine using a
weighted vote schema. Expert Syst. Appl., 31(2):427–
435.
Dietterich, T. G. (2000). Ensemble methods in machine
learning. In Multiple classifier systems, LBCS-1857,
pages 1–15. Springer.
Enr
´
ıquez, F., Cruz, F. L., Ortega, F. J., Vallejo, C. G., and
Troyano, J. A. (2013). A comparative study of clas-
sifier combination applied to nlp tasks. Information
Fusion, 14(3):255–267.
Foody, G., Mathur, A., Sanchez-Hernandez, C., and Boyd,
D. (2006). Training set size requirements for the clas-
sification of a specific class. Remote Sensing of Envi-
ronment, 104(1):1–14.
Frank, E. and Bouckaert, R. R. (2006). Naive bayes for text
classification with unbalanced classes. In In Proc 10th
European Conference on Principles and Practice of
Knowledge Discovery in Databases, pages 503–510.
Freund, Y. and Schapire, R. E. (1997). A decision-theoretic
generalization of on-line learning and an application
to boosting. Journal of Computer and System Sci-
ences, 55(1):119–139.
Guo, G., Wang, H., Bell, D. A., Bi, Y., and Greer, K. (2004).
An knn model-based approach and its application in
text categorization. In In Proc 5th International Con-
ference on Computational Linguistics and Intelligent
Text Processing, pages 559–570.
Huang, Y. and Suen, C. Y. (1993). The behavior-knowledge
space method for combination of multiple classifiers.
In Computer Vision and Pattern Recognition, 1993.
Proceedings CVPR ’93., 1993 IEEE Computer Soci-
ety Conference on, pages 347–352.
Hutter, F., Hoos, H. H., and St
¨
utzle, K. L.-B. T.
(2009). ParamILS: an automatic algorithm configu-
ration framework. Journal of Artificial Intelligence
Research, 36:267–306.
Jordan, M. I. and Jacobs, R. A. (1993). Hierarchical mix-
tures of experts and the EM algorithm. Technical Re-
port AIM-1440.
Kittler, J., Hatef, M., Duin, R. P. W., and Matas, J. (1998).
On combining classifiers. IEEE transactions on pat-
tern analysis and machine, 20:226–239.
Kuncheva, L. I. (2004). Combining Pattern Classifiers:
Methods and Algorithms. Wiley-Interscience.
Kuncheva, L. I., Bezdek, J. C., and Duin, R. P. W.
(2001). Decision templates for multiple classifier fu-
sion: an experimental comparison. Pattern Recogni-
tion, 34:299–314.
Rocchio, J. J. (1971). Relevance feedback in information re-
trieval. In The Smart retrieval system - experiments in
automatic document processing, pages 313–323. En-
glewood Cliffs, NJ: Prentice-Hall.
Ruiz, M. E. and Srinivasan, P. (2002). Hierarchical text cat-
egorization using neural networks. Information Re-
trieval, 5:87–118.
Salton, G. and Buckley, C. (1988). Term-weighting ap-
proaches in automatic text retrieval. In Information
processing and management, pages 513–523.
Salton, G., Wong, A., and Yang, C. S. (1975). A vector
space model for automatic indexing. Commun. ACM,
18(11):613–620.
S
´
anchez, J. S., Barandela, R., Marqu
´
es, A. I., Alejo, R.,
and Badenas, J. (2003). Analysis of new techniques
to obtain quality training sets. Pattern Recognition
Letters, 24(7):1015–1022.
Wolpert, D. H. (1992). Stacked generalization. Neural Net-
works, 5:241–259.
HEALTHINF2014-InternationalConferenceonHealthInformatics
452