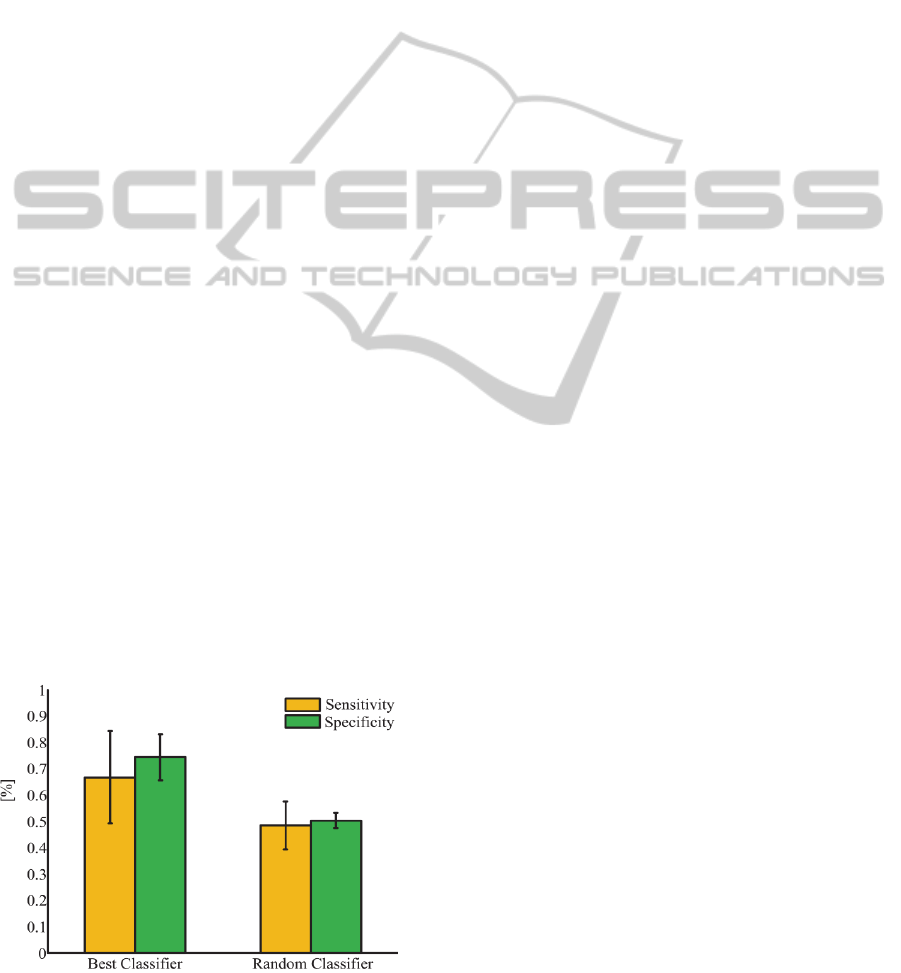
sampling of the majority class (No-Event) was
performed in the training phase of the classifier. This
was done in order to have the same number of Pre-
FOG and No Event windows to train the classifier
with. If no under-sampling had been performed, the
classifier would have “learned” mostly No-Event
patterns thus leading to high specificity but very
poor sensitivity.
In order to test the significance of results, a
random classifier was made, which randomly
assigned “No-Event” or “pre-FOG” classes based on
the proportion of classes in the under-sampled
training set. One would expect such a classifier to
perform with sensitivity and specificity around 50%.
3 RESULTS AND DISCUSSION
Results are reported in Fig. 2.
The best obtained result, in terms of trade-off
between sensitivity and specificity (arithmetic
mean), was
- Sensitivity: 66.5 %
- Specificity: 73.9 %,
In the following, the details of all the parameters of
the data analysis corresponding to this result are
listed:
- Thigh sensor
- Norm of the signal
- Symbolic Frequency of 16 Hz
- Duration of the windows of 2 seconds
- Alphabet size of 10
Both sensitivity and specificity of this combination
resulted significantly better than the ones of the
random classifier (Fig. 3), which performed, as
expected, with sensitivity and specificity around
50%.
Figure 3: Comparison between the performance of the best
classifier and of the random classifier.
From results in Fig. 2 it can be noted that
different combinations of sensors/signals/ frequency
can lead to higher specificity or higher sensitivity
(but not to both).
From results in Fig. 2 it can also be noted that
thigh sensor seems to perform generally better than
ankle and trunk sensors in sensitivity, and
comparably in specificity.
Also, sensitivity estimates tend to be less
consistent (higher variability of performance across
subjects) than specificity estimates.
Although the best result is obtained with a 2-
seconds window, it seems that there is not a clear
difference or pattern in considering windows of
different durations.
Finally, considering different symbolic
frequencies leads to different combinations of
sensitivity and specificity but no consistent pattern
can be observed (e.g. higher symbolic frequency
always leads to better sensitivity/specificity).
Interestingly, the sensitivity in discriminating
between pre-FOG patterns and normal activity is
comparable to the sensitivity in discriminating
between FOG patterns and normal activities
obtained by previous studies (73.1% in Bächlin
2010, 66.3% in Mazilu 2012, 68.5% in Mazilu
2013).
On the other hand, specificity is lower than the
ones obtained in those studies (81.6% in Bächlin
2010, 95.4% in Mazilu 2012, 86.8% in Mazilu
2013).
However, an overall lower performance was
expected because the task of discriminating the
patterns before the event occurs is generally more
complex than detecting the event after it has
happened.
These preliminary findings demonstrate that it is
possible to identify (some of) the motor patterns that
eventually lead to FOG events before they occur,
support the idea that the gait pattern changes prior to
freezing, and suggest that this pre-event period can
be automatically identified by using wearable
sensors.
As a limitation of this study, the algorithm
presented in this study was not optimized for speed;
in future work, a real-time implementation should be
done.
Moreover, the use of different classifiers and the
fusion of decisions made from different
combinations of sensors, time windows and
frequencies, could possibly permit to improve the
obtained results.
AutomaticIdentificationofMotorPatternsLeadingtoFreezingofGaitinParkinson'sDisease-AnExploratoryStudy
733