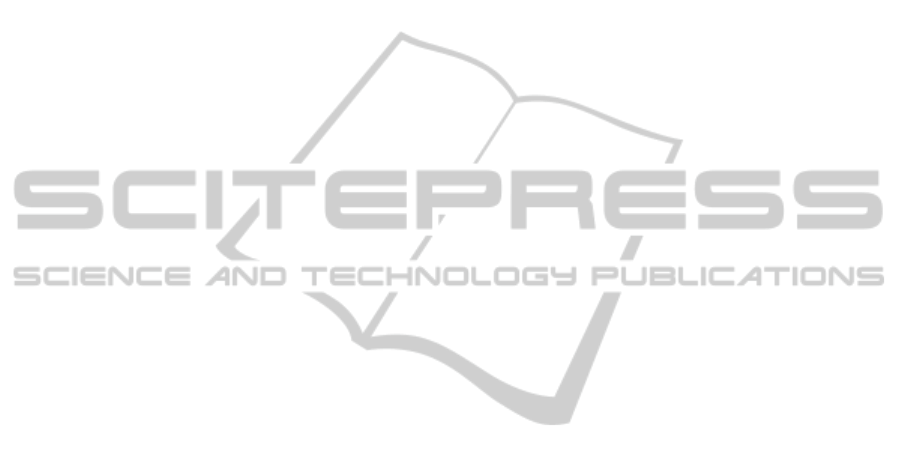
In this position paper, we discussed how computer
vision and video analysis can be employed to evalu-
ate video data from sensorimotor assessment, a diag-
nostic protocol for screening pervasive developmental
disorders such as autism.
Our next steps aim at concluding the analysis of
the “grab the stick test” as discussed hereby. This will
allow us to measure the times of reaction for all sticks
and estimate their variability for each patient. Then
a global study of these results should help sustain or
reject both task understanding and learning hypothe-
ses. We shall also verify the relation between sensori-
motor impairment and mouth gestures throughout the
test. A set of common mouth gestures must be de-
fined in order to distinguish them from unusual ones.
One solution to this problem is to model mouth ges-
tures through the Facial Action Coding System, an
approach adopted in successful recent works (Mahoor
et al., 2009; Senechal et al., 2013).
Future research shall also deal with video editing
and other behavior analysis from video. The first cat-
egory concerns the automatic detection of zooming
and video passages that provide insufficient content
for an analysis by either a human expert or computer
vision tools, as it is the case when sticks or hands are
not visible in a scene. The automatic segmentation of
the assessment videos into passages corresponding to
distinct sensorimotor activities shall also be exploited.
The second category covers the study of signs of
fatigue and loss of attention during the assessment.
This research shall evaluate the videos thoroughly in
order to look for remarkable signs, such as yawning or
torso relaxation and bending. The clinical motivation
is to perceive common signs related to these manifes-
tations, as well as to understand which stimuli might
help the child to regain attention.
ACKNOWLEDGEMENTS
The videos used in this study were acquired with the
informed consent of the parents, who agreed to the
use of the data for educational and research purposes.
Ana B. G. Fouquier was the recipient of a Post
Doctoral Fellowship provided by the Brazilian Na-
tional Council for Scientific and Technological De-
velopment (CNPq).
REFERENCES
Albiol, A., Torres, L., and Delp, E. (2001). Optimum color
spaces for skin detection. In Proc. IEEE Int. Conf.
Image Process., volume 1, pages 122–124.
Bernard, J. et al. (2006). Evolution de la fr´equence des
gestes chez des garc¸ons avec autisme ˆag´es de 1 `a 3 ans
par analyse de vid´eos familiales. Devenir, 18(3):245–
261.
Bullinger, A. (2006). Approche sensorimotrice des trou-
bles envahissants du d´eveloppement. Contraste,
2(25):125–139.
Hashemi, J. et al. (2012). A computer vision approach for
the assessment of autism-related behavioral markers.
In Proc. IEEE Int. Conf. Development and Learning
and Epigenetic Robotics (ICDL), pages 1–7.
Jones, M. J. and Rehg, J. M. (2002). Statistical color mod-
els with application to skin detection. Int. J. Comput.
Vision, 46(1):81–96.
Jutard, C., Kloeckner, A., P´erisse, D., and Cohen, D.
(2009). Int´erˆet de l’abord sensorimoteur dans
les pathologies autistiques s´ev`eres II : illustration
clinique. Neuropsychiatrie de l’Enfance et de
l’Adolescence, 57(2):160 – 165.
Kloeckner, A. et al. (2009). Int´erˆet de l’abord sensorimoteur
dans les pathologies autistiques s´ev`eres I : introduc-
tion aux travaux d’Andr´e Bullinger. Neuropsychiatrie
de l’Enfance et de l’Adolescence, 57(2):154 – 159.
Mahoor, M. et al. (2009). A framework for automated mea-
surement of the intensity of non-posed facial action
units. In IEEE Comput. Soc. Conf. Comput. Vision
and Pattern Recognition Workshops, pages 74–80.
Matas, J., Galambos, C., and Kittler, J. (2000). Robust
detection of lines using the progressive probabilistic
hough transform. Comput. Vision and Image Under-
standing, 78(1):119–137.
P´erez, P., Hue, C., Vermaak, J., and Gangnet, M. (2002).
Color-based probabilistic tracking. In Proc. European
Conf. Comput. Vision, pages 661–675.
Porayska-Pomsta, K. et al. (2012). Developing technology
for autism: an interdisciplinary approach. Personal
Ubiquitous Comput., 16(2):117–127.
Rehg, J. M. (2011). Behavior imaging: Using computer
vision to study autism. In IAPR Conf. Mach. Vision
Appl., pages 14–19.
Rehg, J. M. et al. (2013). Decoding children’s social behav-
ior. In Proc. IEEE Comp. Soc. Conf. Comput. Vision
and Pattern Recognition, pages 3414–3421.
Senechal, T. et al. (2013). Smile or smirk? automatic detec-
tion of spontaneous asymmetric smiles to understand
viewer experience. In Int. Conf. Automatic Face and
Gesture Recognition, pages 1–8.
Wendland, J. et al. (2010). Retrait relationnel et signes
pr´ecoces d’autisme : ´etude pr´eliminaire `a partir de
films familiaux. Devenir, 22(1):51–72.
Zakian, A. et al. (2000). Early signs of autism: A new study
of family home movies. L’Enc´ephale, 26:38–44.
ICPRAM2014-InternationalConferenceonPatternRecognitionApplicationsandMethods
740