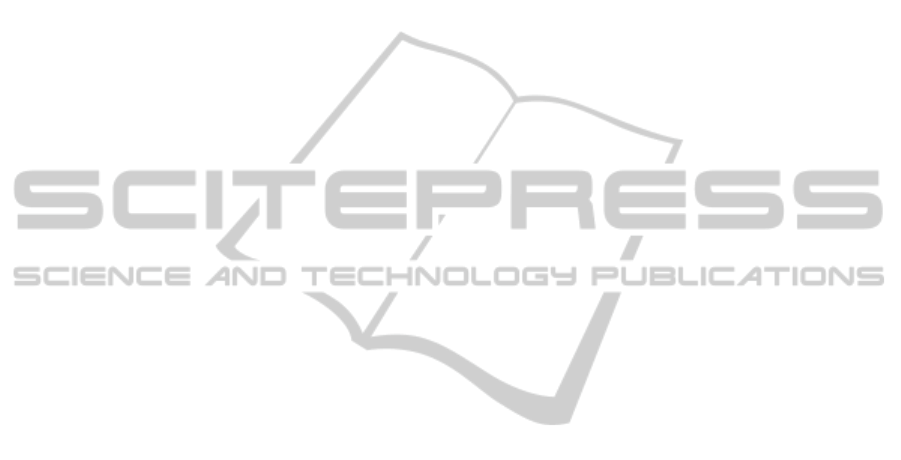
5 DISCUSSION
Vein images from a near-infrared camera may result
in the low quality because of the worse S/N ratio.
Therefore, the efficient filtering process will be
necessary for quantifying venous changes. The
proposed filtering method based on the GA-EM
algorithm sufficiently modified the low-contrast vein
images during a blood pressure stimulus, under an
unknown correct image answer. However, the ability
of the EM algorithm is influenced by the method of
selection of the initial parameters. Furthermore, the
design of a novel filter with the EM algorithm must
avoid singularity or note identifiability (Casella and
Berger, 2002).
The same filtering procedure is not optimal for
all situations because the accuracy of image
processing depends on inter- or intra- individual
variability of vein shapes. The typical image
processing will need the adjustment of the camera or
filtering parameter settings every measurement
environment or an acquired image, by trial and error.
The proposed filtering method will sufficiently
improve the images obtained from such situations.
6 CONCLUSIONS
The image filter made by the GA-EM algorithm was
able to efficiently detect vein shapes recorded by an
infrared camera. The filtered images were quantified
with the EM algorithm to assess venous changes
during the blood pressure measurement. The
proposed imaging filter was able to modify its kernel
array, adapting the feature of target images. In future
studies, the optimal range of parameters for the GA-
EM algorithm should be investigated to catch
abnormal veins at an early stage. If the proposed
filtering method is incorporated into the e-healthcare
application, it could be widely distributed through
smart phones or tablets.
ACKNOWLEDGEMENTS
This study was partially funded by a Grant-in-Aid
for Scientific Research (C) from Japan Society for
the Promotion of Science (KAKENHI, 25330171).
REFERENCES
Feltracco, P., Barbieri, S., Bertamini, F., Michieletto, E.,
Ori, C., 2007. Economy class syndrome: still a
recurrent complication of long journeys. Eur. J.
Emerg. Med., vol. 14, pp. 100–103.
Callam, M. J., 1994. Epidemiology of varicose veins. Br.
J. Surg., vol. 81, pp. 167–173.
Bergqvist, D., Jaroszewski, H., 1986. Deep vein
thrombosis in patients with superficial
thrombophlebitis of the leg. Br. Med. J. (Clin. Res.
Ed.), vol. 292, pp. 658–659.
Kashihara, K., Ito, M., Fukumi, M., 2012. Estimation of
venous shapes acquired from CMOS camera images.
Proc. of the Eighteenth Korea-Japan Joint Workshop
on Frontiers of Computer Vision (FCV2012), pp. 47–
52.
Zharov, V. P., Ferguson, S., Eidt, J. F., Howard, P. C.,
Fink, L. M., Waner, M., 2004. Infrared imaging of
subcutaneous veins. Lasers Surg. Med., vol. 34, pp.
56–61.
Soni, M., Gupta, S., Rao, M. S., Gupta, P., 2010. A new
vein pattern-based verification system. International
Journal of Computer Science and Information Security,
vol. 8, pp. 99–105.
Yakno, M., Saleh, J. M., Rosdi, B. A., 2011. Low contrast
hand vein image enhancement. Proc. of 2011 IEEE
International Conference on Signal and Image
Processing Applications (ICSIPA), pp. 390–392.
Bilmes, J. A., 1998. A gentle tutorial of the EM algorithm
and its application to parameter estimation for
Gaussian mixed and hidden Markov models. U.C.
Berkely, TR–97–021.
Eshelman, L. J., Schaffer, J. D., 1993. Real-coded genetic
algorithms and interval-schemata. Foundations of
genetic algorithms, vol. 2, pp. 187–202.
Casella, G., Berger, R. L., 2002. Statistical Inference, 2nd
ed., Pacific Grove, CA: Duxbury.
HEALTHINF2014-InternationalConferenceonHealthInformatics
510