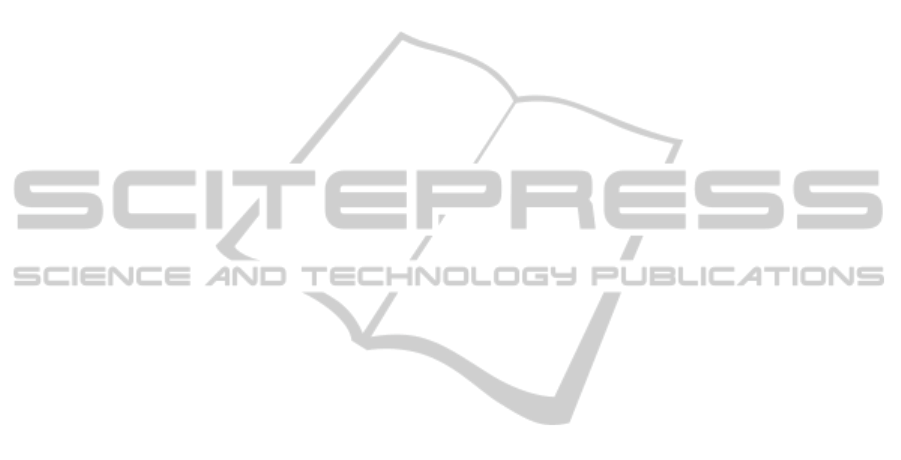
REFERENCES
Chin, K., DeVries, S., Fridlyand, J., Spellman, P.T.,
Roydasgupta, R., Kuo, W.-L., Lapuk, A., Neve, R.M.,
Qian, Z., Ryder, T., Chen, F., Feiler, H., Tokuyasu, T.,
Kingsley, C., Dairkee, S., Meng, Z., Chew, K., Pinkel,
D., Jain, A., Ljung, B. M., Esserman, L., Albertson, D.
G., Waldman, F. M., Gray, J. W., 2006. Genomic and
transcriptional aberrations linked to breast cancer
pathophysiologies. Cancer Cell 10, 529–541.
Cortes, C., Vapnik, V., 1995. Support-vector networks.
Machine learning 20, 273–297.
Desmedt, C., Piette, F., Loi, S., Wang, Y., Lallemand, F.,
Haibe-Kains, B., Viale, G., Delorenzi, M., Zhang, Y.,
D’ Assignies, M. S., Bergh, J., Lidereau, R., Ellis, P.,
Harris, A. L., Klijn, J. G. M., Foekens, J. A., Cardoso,
F., Piccart, M. J., Buyse, M., Sotiriou, C., On behalf of
the TRANSBIG Consortium, 2007. Strong time
dependence of the 76-gene prognostic signature for
node-negative breast cancer patients in the
TRANSBIG multicenter independent validation series.
Clinical Cancer Research 13, 3207–3214.
Efron, B., Tibshirani, R., 1995. Cross-validation and the
bootstrap: Estimating the error rate of a prediction
rule. Division of Biostatistics.
Galea, M. H., Balmey, R. W., Elston, C. E., Ellis, I. O.,
1992. The Nottingham Prognostic Index in primary
breast cancer. Breast cancer research and treatment
22, 207–219.
Guo, Z., Zhang, T., Li, X., Wang, Q., Xu, J., Yu, H., Zhu,
J., Wang, H., Wang, C., Topol, E., others, 2005.
Towards precise classification of cancers based on
robust gene functional expression profiles. BMC
Bioinformatics 6, 58.
Ibrahim, M., Jassim, S., Cawthorne, M.A., Langlands, K.,
2012. Integrating pathway enrichment and gene
network analysis provides accurate disease
classification. The International Conference on
Bioinformatics Models, Methods and Algorithms. 156–
163.
Khunlertgit, N., Yoon, B.-J., 2013. Identification of robust
pathway markers for cancer through rank-based
pathway activity inference. Advances in
Bioinformatics 2013, 1–8.
Loi, S., Haibe-Kains, B., Desmedt, C., Lallemand, F.,
Tutt, A. M., Gillet, C., Ellis, P., Harris, A., Bergh, J.,
Foekens, J. A., Klijn, J. G. M., Larsimont, D., Buyse,
M., Bontempi, G., Delorenzi, M., Piccart, M. J.,
Sotiriou, C., 2007. Definition of clinically distinct
molecular subtypes in estrogen receptor-positive
breast carcinomas through genomic grade. Journal of
Clinical Oncology 25, 1239–1246.
Nacu, Ş., Critchley-Thorne, R., Lee, P., Holmes, S., 2007.
Gene expression network analysis and applications to
immunology. Bioinformatics 23, 850–858.
Paik, S., Shak, S., Tang, G., Kim, C., Baker, J., Cronin,
M., Baehner, F. L., Walker, M. G., Watson, D., Park,
T., 2004. A multigene assay to predict recurrence of
tamoxifen-treated, node-negative breast cancer. New
England Journal of Medicine 351, 2817–2826.
Van Vliet, M. H., Reyal, F., Horlings, H. M., Van de
Vijver, M. J., Reinders, M. J., Wessels, L. F., 2008.
Pooling breast cancer datasets has a synergetic effect
on classification performance and improves signature
stability. BMC Genomics 9, 375.
Van’t Veer, L. J., Dai, H., Van De Vijver, M. J., He, Y.
D., Hart, A. A. M., Mao, M., Peterse, H. L., Van Der
Kooy, K., Marton, M. J., Witteveen, A. T., others,
2002. Gene expression profiling predicts clinical
outcome of breast cancer. Nature 415, 530–536.
Venet, D., Dumont, J. E., Detours, V., 2011. Most random
gene expression signatures are significantly associated
with breast cancer outcome. PLoS Computational
Biology 7, e1002240.
Wang, Y. C., Chen, B. S., 2011. A network-based
biomarker approach for molecular investigation and
diagnosis of lung cancer. BMC medical genomics 4, 2.
Wesolowski, R., 2011. Gene expression profiling:
changing face of breast cancer classification and
management. Gene expression 15, 105–115.
BIOINFORMATICS2014-InternationalConferenceonBioinformaticsModels,MethodsandAlgorithms
270