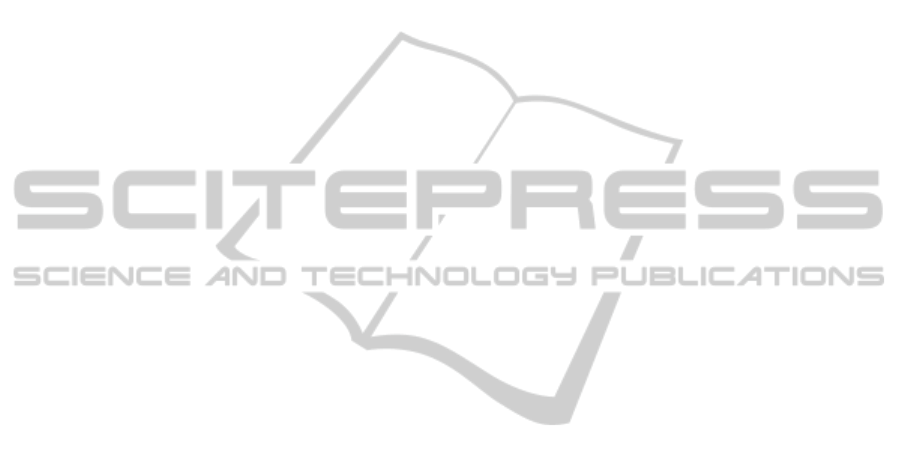
5 CONCLUSIONS
Proposed model extends and improves the model for
simulating the dynamics of information
dissemination within the social network presented in
the earlier work of the author. The system of
messages is now used, which is crucial for future
work. The model can be used to study the dynamics
in social networks of varying size and orientation
created for the purpose of information exchange
(such as corporate networks, on-line services, local
structures aimed to solving everyday situations,
etc.), not limited to on-line services and electronic
transmission of information.
The presented results are only the beginning of
the exploring and simulating of the social networks
with this model, but experiments shown that model
provides interesting outputs comparable with the
behavior of individuals in the real world.
The model is continuously developing and
modifying. We plan to do much more experiments to
examine the influence of all parameters on the
network dynamics. We are constantly searching for
a suitable user interface, too. Stress will also be laid
on model of the quality verification and setting
model parameters using data from real social
networks. The mid-term goal is also to implement in
more detail the emotional states of agents.
ACKNOWLEDGEMENTS
This work was supported by internal grant of the
Institute of Technology and Business in České
Budějovice, grant No. 1/2013.
REFERENCES
Ahmad, M. A., Teredesai, A., 2006. Modeling spread of
ideas in online social networks. In: Proceedings of the
Fifth Australasian Conference on Data Mining and
Analystics - Volume 61 (Sydney, Australia, November
29 - 30, 2006). Australian Computer Society,
Darlinghurst, Australia.
Balint, M., Posea, V., Dimitriu, A., Iosup, A., 2011. An
analysis of social gaming networks in online and face
to face bridge communities. In Proceedings of the
third international workshop on Large-scale system
and application performance (LSAP '11). ACM, New
York, NY, USA.
Federico, P., Aigner, W., Miksch, S., Windhager, F.,
Zenk, L., 2011. A visual analytics approach to
dynamic social networks. In Proceedings of the 11th
International Conference on Knowledge Management
and Knowledge Technologies (i-KNOW '11), Stefanie
Lindstaedt and Michael Granitzer (Eds.). ACM, New
York, NY, USA.
Jelínek, J., 2011. Modeling of information flow in social
networks (in Czech) In: CEUR Workshop
Proceedings, Vol-802, http://ceur-ws.org/Vol-802/
(online), ISBN: 978-80-248-2369-0.
Ma, H., Huang, J., 2013. CUT: community update and
tracking in dynamic social networks. In Proceedings
of the 7th Workshop on Social Network Mining and
Analysis (SNAKDD '13). ACM, New York, NY,
USA.
Menges, F., Mishra, B., and Narzisi, G., 2008. Modeling
and simulation of e-mail social networks: a new
stochastic agent-based approach. In: Proceedings of
the 40th Conference on Winter Simulation (Miami,
Florida, December 07 - 10, 2008).
Palazuelos, C., Zorrilla, M., 2012. Analysis of social
metrics in dynamic networks: measuring the influence
with FRINGE. In Proceedings of the 2012 Joint
EDBT/ICDT Workshops (EDBT-ICDT '12), Divesh
Srivastava and Ismail Ari (Eds.). ACM, New York,
NY, USA.
Siebers, P.O. and Aickelin, U., 2007. Introduction to
Multi-Agent Simulation. In: Encyclopedia of Decision
Making and Decision Support Technologies. IDEAS
Group.
Wasserman, S. and Faust, K., 1994. Social Network
Analysis. Cambridge University Press, ISBN
0521382696.
Wu, W., Zhang, Y., 2010. Stability analysis in dynamic
social networks. In Proceedings of the 2010 Spring
Simulation Multiconference (SpringSim '10). Society
for Computer Simulation International, San Diego,
CA, USA.
Zhao, X., Sala, A., Wilson, Ch., Wang, X., Gaito S.,
Zheng, H., Zhao, B., 2012. Multi-scale dynamics in a
massive online social network. In Proceedings of the
2012 ACM conference on Internet measurement
conference. ACM, New York, NY, USA.
InformationDisseminationinSocialNetworks
271