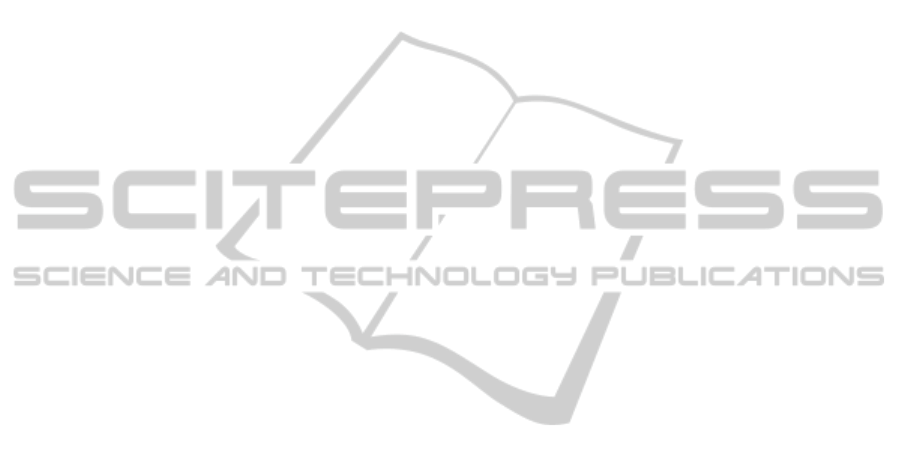
compliance with social conventions, awareness to
the inner state of emotion or motives, or the ability
to act and interact in a consistent and transparent
way (Aarts and de Ruyter, 2009). The analysis
process must therefore imply several levels of
abstraction, from the local level of the data to a more
global level of norms, functional requirements and
goals (Weber and Glynn, 2006). Although some
contextual situations are fairly stable, discernible,
and predictable, there are many others that are not:
for (Greenberg, 2001), context must be seen as a
dynamic construct evolving with time, episodes of
use, social interaction, internal goals, and local
influences. Following (Klein et al., 2006), we will
approach interpretation not as a state of knowledge,
but rather as “a process of fitting data into a frame
that is continuously replaced and adapted to fit the
data”. As a consequence, we may not reduce the
understanding process to the description and
handling of contextual elements, nor to the mere
application of data-driven or goal-driven methods.
On the contrary, this process must be seen as the
constant perception-action loop, which consists in
focus, perception, interpretation, context modelling
and anticipation. The paradigm of Multi-Agent
Systems allows for multiple, heterogeneous entities
to be handled through a unified communication /
cooperation frame (Isern et al., 2010). The
heterogeneity of agents is considered as a
requirement to encompass the variety of states a
person can find oneself in; a large knowledge base
of interconnected interpretation models can thus be
explored, as a dynamic population of multiple
hypotheses on several levels of abstraction. A law
enforcement approach has been proposed (Carvalho
et al., 2005) to support dependable open software
development in the context of ambient intelligence
environments. Following these lines of approach, we
propose using the normative agent paradigm as a
way to design flexible context-aware norm-driven
systems. Normative MAS are a class of Multi-Agent
Systems in which agent behaviour is not only guided
by their mere individual objectives but also
regulated by norms specifying in a declarative way
which actions are considered as legal or not by the
group (Castelfranchi, 2006). Agents acting in such a
system may be seen as “socially autonomous": they
do not only pursue their own goals but are also able
to adopt goals from the outside, and act in the best
interest of the society. An additional control over the
adoption of goals is therefore needed, in the form of
norms, which operate as external incentives for
action (Dignum et al., 2000). These norms are
designed as condition-action rules, triggered by a
dedicated engine, and result in agent notifications.
Agents subscribe to norms and may adopt them or
not, which may result in penalties. Norms may
finally be adapted to cope with the evolution of
context (Boissier et al., 2011). The system dynamics
therefore depends not only on the agent dynamics
but also on the dynamic of the norms.
3 PROPOSED ARCHITECTURE
3.1 Proposed View
According to (Hébert, 2002), we propose to
distinguish between intrinsic and extrinsic
perspectives to interpretation. Intrinsic interpretation
is domain-dependent: it is based on attributes and
classes that are inherent to the activity domain.
Intrinsic interpretation drives the construction of
hypotheses, which may be concurrent and
contradictory, as to whether a person is running,
walking, or staying quiet. Extrinsic interpretation is
observer-dependent: it relies on attributes and
classes that are inherent to the observer domain.
Extrinsic interpretation drives the construction of an
evaluative view over human activity, regarding
whether this activity is normal or alarming. It may
further apply to the way intrinsic interpretation is
conducted and provides a view as to whether this
process is efficient, informative, or for example open
or restricted to few hypotheses. These two universes
of discourse are mutually dependent. Indeed, the
evolution of intrinsic activity properties may yield
the evolution of extrinsic evaluation models, e.g. a
skiing or walking activity calls for different evalua-
tive models. Conversely, the observer’s extrinsic
evaluation provides a perspective view that drives
what is looked at, e.g. focus on speed if there is a
risk of fall for a skiing activity, focus on location if
there is a risk of getting lost for a walking activity.
Interpretation is modelled as a dynamic
exploration process driven in a context-sensitive
way. As proposed in a previous paper (Vettier and
Garbay, 2014), it is abductive in nature and
modelled as a perception-action loop combining
prediction and verification stances. This process is
situated with respect to a set of intrinsic and
extrinsic require-ments. Intrinsic requirements are
domain-dependent: interpretation hypotheses are to
be grounded into the evidence of data and the realm
of the activity at hand. Their confidence has to be
high enough for the hypothesis to be maintained:
otherwise other hypotheses must be evaluated.
ICAART2014-InternationalConferenceonAgentsandArtificialIntelligence
674