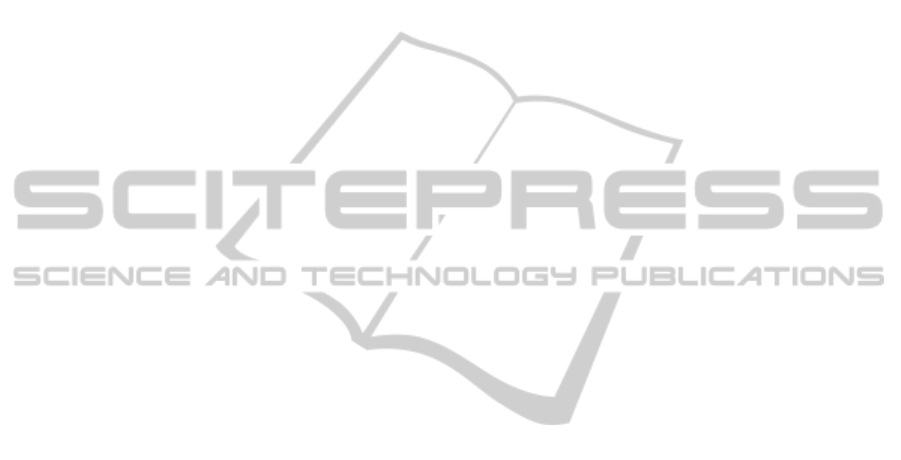
In the particular sector of garment and apparel dis-
tribution, (Bahng and Kincade, 2012) provide strong
evidence that fluctuation in temperature can impact
sales of seasonal garments on a daily basis. However,
no evidence has been found to substantiate an impact
on sales of a whole season. (Rowley, 1999) prove
what retailers had long suspected, ie, that precipita-
tion affected substantially actual clothing purchases.
The classic example can be found in the northern
hemisphere: the placement of Easter in the calendar
affects seasonal retail sales pattern depending upon
whether it is in early March or late April. Generaliz-
ing, (Starr-McCluer, 2000) estimated that the effect of
weather on retail sales in the United States has a small
but statistically significant role in explaining monthly
retail sales.
3 DATA AND METHODS
3.1 Data
The analysis of this relationship relies on choosing
the most representative set of economic output, rele-
vant weather data, and appropriate quantitative tech-
niques. The objective is to prove the existence of a
statistically significant relationship and establish the
weather-sensitivity relationship in the case of textile
and clothing.
3.1.1 Defining Weather Sensitivity
Weather exposure is the amount of revenues or costs
at risk, which results from changes in weather con-
ditions (Brockett et al., 2005). Many businesses ex-
perience some form of seasonal pattern in their activ-
ity. For example, electricity consumption is higher in
winter than in summer, ice cream sales are stronger
in summer than in winter, and so on. Business man-
agers can execute their plans as long as the weather
patterns remain typical. Potential gains or losses arise
when weather conditions unexpectedly deviate from
their normal values. Meteorologists refer to these
deviations as weather anomalies. Because they are
unexpected, weather anomalies, or weather surprises
(Roll, 1984), can potentially change the economic
performance of a firm or a sector. Normal weather
conditions are calculated as the average weather over
30 years (Baede, 2001; Dischel, 2002).
The average weather is the climate. Climate vari-
ability is the extent to which actual weather differs
from this climate. Climate variability causes potential
disruption in economic conditions (Dischel, 2002).
How much less will the ice-cream producer sell if
the temperature is cooler than normal? How much
more will the motorway operator spend to clear roads
if snowfall is higher than in a typical winter? An
economic sector is considered to be weather sensi-
tive if weather anomalies can explain a percentage of
the performance of the sector. In other words, a sec-
tor is weather sensitive if it is possible to establish a
statistically significant relationship between weather
anomalies and change in revenues: The stronger the
relationship, the higher the sensitivity to weather.
3.1.2 Time Series from the Institut Franc¸ais de
la Mode
The first weather-sensitivity research papers focused
on U.S. economic output. Gross Domestic Product
(GDP) or gross state product data for the 11 non-
governmental sectors were used as a measure of eco-
nomic activity principally because time-series were
sufficiently long to apply statistical analysis (Dutton,
2002; Larsen, 2006; Lazo et al., 2011).
Similarly, one important data source which could
have been used are monthly turnover indices pub-
lished by the French National Bureau of Statistics
(INSEE). These indices aggregate turnover figures of
firms falling within the scope of value-added tax pay-
ment. They are available at the most detailed level of
the European classification of activities. More impor-
tant, turnover indices are volume-based, which make
them particularly relevant as a base for testing the im-
pact of weather anomalies, as weather risk is a volume
risk (Brockett et al., 2005; Barrieu, 2003; Dischel,
2002). Though data starts in January 1995, only two
sectors could have been used for our analysis (tex-
tile retail sales in specialized stores and retail sale via
stalls and markets of textiles, clothing and footwear).
Moreover, the level of detail is insufficient.
We used data provided by IFM, the French Insti-
tute of Fashion. Researchers from the Economic Ob-
servatory of IFM have been gathering sales figures
in volume from a panel of thousands of textile and
clothing retailers across France since January 2000.
Panel members range from independent multi-brand
clothing stores to specialized single brand chain stores
and department stores. Data is available by garment
type for women, men and children. Sales figures are
compiled and analyzed by IFM in a survey (Distribi-
lan) to highlight major trends and changes by product
category and distribution channel year on year on a
monthly basis (see table 1).
ICORES2014-InternationalConferenceonOperationsResearchandEnterpriseSystems
278