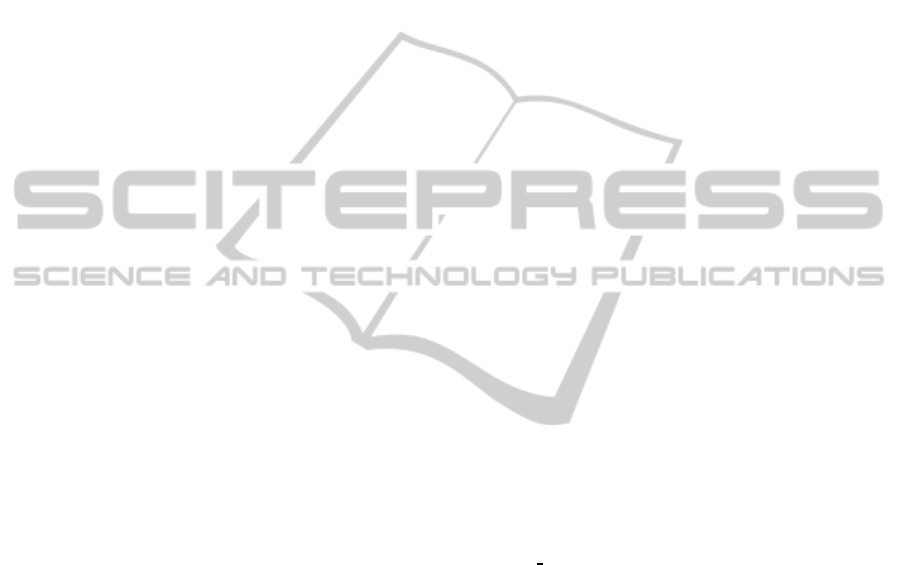
over the entire sequence. In this way we are sure that
when the sliding window is centered around the ges-
ture the corresponding NN will provide the maximum
answers, while when the window overlaps the ending
or the beginning parts of the gestures some false pos-
itive answers can be provided. The obtained results
are very encouraging as the number of false positives
is always smaller than the true positives. Furthermore
by filtering on the number of consecutive concordant
answers a correct final decision can be taken. Tests
executed on persons different from those used in the
training set have demonstrated that the proposed sys-
tem can be trained off line and used for the gesture
recognition by any other user with the only constraint
of repeating the same gesture more times.
In future work we will face the problem of the
length of the gestures. In this paper we have imposed
that the gestures are all executed in 2 seconds corre-
sponding to 60 frames. When the gestures are exe-
cuted with different velocities the correct association
is not guaranteed. Current researches focus on the
automatic detection of the gesture length and on the
normalization of all the executions by interpolating
the missing values.
ACKNOWLEDGEMENTS
This research has been developed under grant PON
01-00980 BAITAH.
REFERENCES
Almetwally, I. and Mallem, M. (2013). Real-time tele-
operation and tele-walking of humanoid robot nao us-
ing kinect depth camera. 10th IEEE International
Conference on Networking, Sensing and Control (IC-
NSC), page 463466.
Bhattacharya, S., Czejdo, B., and Perez, N. (2012). Gesture
classification with machine learning using kinect sen-
sor data. Third International Conference on Emerging
Applications of Information Technology (EAIT), pages
348 – 351.
Biswas, K. and Basu, S. (2011). Gesture recognition using
microsoft kinect. 5th International Conference on Au-
tomation, Robotics and Applications (ICARA), pages
100–103.
Castiello, C., D’Orazio, T., Fanelli, A., Spagnolo, P., and
Torsello, M. (2005). A model free approach for pos-
ture classificatin. IEEE Conf. on Advances Video and
Signal Based Surveillance, AVSS.
Cheng, L., Sun, Q., Cong, Y., and Zhao, S. (2012). De-
sign and implementation of human-robot interactive
demonstration system based on kinect. 24th Chi-
nese Control and Decision Conference (CCDC), page
971975.
Cruz, L., Lucio, F., and Velho, L. (2012). Kinect and rgbd
images: Challenges and applications. XXV SIBGRAPI
IEEE Confernce and Graphics, Patterns and Image
Tutorials, page 3649.
den Bergh, M. V., Carton, D., de Nijs, R., Mitsou, N., Land-
siedel, C., Kuehnlenz, K., Wollherr, D., Gool, L. V.,
and Buss, M. (2011). Real-time 3d hand gesture inter-
action with a robot for understanding directions from
humans. 20th IEEE international symposium on robot
and human interactive communication, pages 357 –
362.
Gu, Y., andY. Ou, H. D., and Sheng, W. (2012). Human
gesture recognition through a kinect sensor. IEEE In-
ternational Conference on Robotics and Biomimetics
(ROBIO), pages 1379 – 1384.
Hachaj, T. and Ogiela, M. (2013). Rule-based approach
to recognizing human body poses and gestures in real
time. Multimedia Systems.
J.Oh, Kim, T., and Hong, H. (2013). Using binary decision
tree and multiclass svm for human gesture recogni-
tion. International Conference on Information Science
and Applications (ICISA), pages 1 – 4.
Lai, K., Konrad, J., and Ishwar, P. (2012). A gesture-
driven computer interface using kinect. IEEE South-
west Symposium on Image Analysis and Interpretation
(SSIAI), pages 185 – 188.
Leo, M., P.Spagnolo, D’Orazio, T., and Distante, A. (2005).
Human activity recognition in archaeological sites by
hidden markov models. Advances in Multimedia In-
formation Procesing - PCM 2004.
Miranda, L., Vieira, T., Martinez, D., Lewiner, T., Vieira,
A., and Campos, M. (2012). Real-time gesture recog-
nition from depth data through key poses learning
and decision forests. 25th SIBGRAPI Conference on
Graphics, Patterns and Images (SIBGRAPI), pages
268 – 275.
SpecialOPeration (2013). Arm-and-hand signals for ground
forces. www.specialoperations.com/Focus/Tactics/
Hand
Signals/default.htm.
ICPRAM2014-InternationalConferenceonPatternRecognitionApplicationsandMethods
746