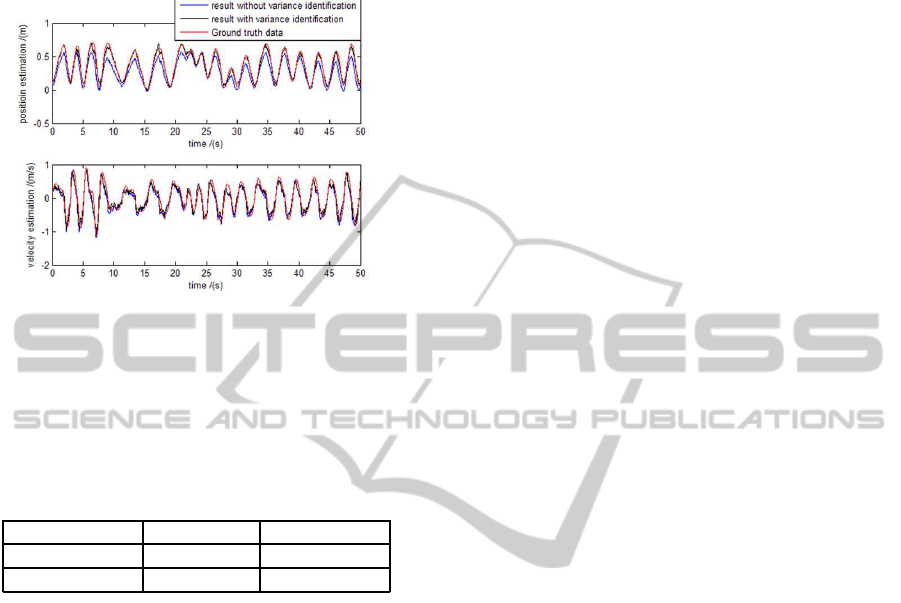
the motion estimation with a fixed empiric variance
value and with the ANVI method are recorded for
comparison.
Figure 6: Result of the motion estimation.
As shown in Figure 6, motion estimation includes
position estimation and velocity estimation, and the
accuracy of both improved with the ANVI method.
The root-mean-square error of the results compared
with the groudtruth data is shown in Table 1.
Table 1: Root-mean-square error of the results in Figure 7.
RMS error position(m) velocity(m/s)
without ANVI 0.110 0.151
with ANVI 0.037 0.105
5 CONCLUSIONS
A novel adaptive variance identification method is
proposed in this paper. Experiment shows that with
this method, variance of the noise could be identified
reliably. With the ANVI method, results of the motion
estimation will basically be optimal.
The method is especially suitable in the vision-
aided motion estimation of UAVs. Because firstly,
the noise of vision location results is changeable and
needs adaptive identification. Secondly, the validity
of the ANVI method is based on the special kinematic
properties of UAVs, as explained in the derivation.
However, since most kinds of robot share the same
kinematic properties that the kinematic acceleration
has a upper limit. Therefore, with certain adjustments
of the parameters, the proposed method could be used
in wide applications.
ACKNOWLEDGEMENTS
The work in this paper was supported by the Na-
tional Science and Technology Major Projects of the
Ministry of Science and Technology of China: ITER
(No.2012GB102007).
REFERENCES
Bosnak, M., Matko, D., and Blazic, S. (2012). Quadro-
copter hovering using position-estimation information
from inertial sensors and a high-delay video system.
Journal of Intelligent & Robotic Systems, 67(1):43–
60.
Chatila, R., Kelly, A., and Merlet, J, P. (2008). Hovering
flight and vertical landing control of a vtol unmanned
aerial vehicle using optical flow. In IEEE/RSJ IROS,
pages 801–806. IEEE.
de Marina, H, G., Pereda, F, J., Giron-Sierra, J, M., and
Espinosa, F. (2012). Uav attitude estimation using un-
scented kalman filter and triad. IEEE Transactions on
Industial Electronics, 59(11):4465–4474.
Edwan, E., Zhang, J, Y., and Zhou, J, C. (2011). Reduced
dcm based attitude estimation using low-cost imu and
magnetometer triad. In Proceedings of the 8th WPNC,
pages 1–6. IEEE.
Farhad, A., Marcin, K., and Galina, O. (2011). Fault-
tolerant position/attitude estimation of free-floating
space objects using a laser range sensor. IEEE Sen-
sors Journal, 11(1):176–185.
Mondrag´on, I., Olivares-M´endez, M., Campoy, P.,
Mart´ınez, C., and Mejias, L. (2010). Unmanned aerial
vehicles uavs attitude, height, motion estimation and
control using visual systems. Autonomous Robots,
29:17–34.
R.Szeliski (2010). Computer Vision: Algorithms and Appli-
cations. Springer, USA, 1nd edition.
Savage, P, G. (1998). Strapdown inertial navigation in-
tegration algorithm design part 1: attitude algo-
rithms. Journal of Guidance, Control, and Dynamics,
21(1):19–28.
Vasconcelos, J, F., Silvestre, C., and Oliveira, P. (2010).
Embedded uav model and laser aiding techniques
for inertial navigation systems. Control Engineering
Practice, 18(3):262–278.
Whitcomb, L., Yoerger, D., and Singh, H. (1999). Advances
in doppler-based navigation of underwater robotic ve-
hicles. In Proceedings of ICRA, volume 1, pages 399–
406. IEEE.
Yoo, C, S. and Ahn, I, K. (2003). Low cost gps/ins sensor
fusion system for uav navigation. In Proceedings of
the 22nd DASC, volume 2, pages 8.A.1–8.1–9. IEEE.
Zhao, H. and Wang, Z, Y. (2012). Motion measurement
using inertial sensors, ultrasonic sensors, and magne-
tometers with extended kalman filter for data fusion.
IEEE Sensors Journal, 12(5):943–953.
ICPRAM2014-InternationalConferenceonPatternRecognitionApplicationsandMethods
758