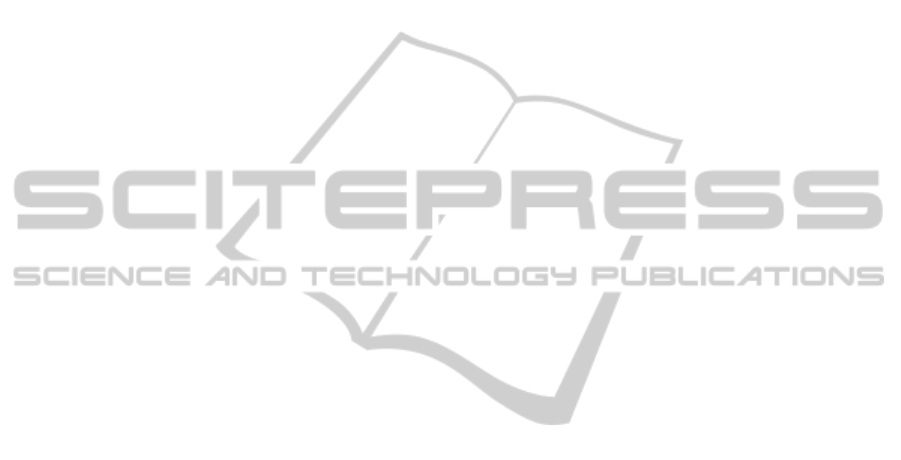
tion and, to a lesser extent, the error rate of the re-
sulting classifier on microarray data and artificial data
of similar dimension. We focused on the aggrega-
tion method used in the ensemble procedure, because
that is an important aspect of the ensemble construc-
tion procedure which, to our knowledge, had scarcely
been investigated in such a setting before. Similarly
to (Haury et al., 2011), we found that average rank ag-
gregation usually performed worse than the other ag-
gregation methods. We also found that average score
aggregation usually resulted, with a few exception, in
the best stability, while stability selection aggregation
was in-between.
We observed, in some cases, a trade-off between
stability and error rate. Previous studies such as
(Saeys et al., 2008) already suggested such a dataset-
dependent trade-off between robustness and classifi-
cation performance. Here, however, we find that the
aggregation method can also play a role in this trade-
off, since in some cases error rate and stability were
differently affected by the different ensemble aggre-
gation methods. This trade-off did not seem to apply
to our artificial data, though: on them, a better er-
ror rate was systematically paired with a better stabil-
ity. This difference could be due to structural differ-
ences with the real data (the latter probably present-
ing much more complex and numerous interactions),
or to a lack of diversity in the artificial data, since the
trade-off is not observed in all datasets. Nonetheless,
we observed that in all cases, the most stable method
without ensemble could be rendered more stable via
ensemble, with or without a trade-off on the classifi-
cation error rate.
As future work, we think it would be interesting
to study or develop more aggregation methods, such
as average weighted rank or score (giving a higher
weight to higher scores or lower ranks). Weighted
(exponential) rank reportedly performed better than
average rank (Haury et al., 2011), so maybe such an
improvement could be obtained by using the same
method on scores. Hybridizationof different base fea-
ture selection methods also seems to be an interesting
area to explore, which will also require some specific
work on the aggregation strategies.
REFERENCES
Abeel, T., Helleputte, T., Van de Peer, Y., Dupont, P., and
Saeys, Y. (2010). Robust biomarker identification
for cancer diagnosis with ensemble feature selection
methods. Bioinformatics, 26(3):392–398.
Dernoncourt, D., Hanczar, B., and Zucker, J.-D. (2014).
Analysis of feature selection stability on high dimen-
sion and small sample data. Computational Statistics
and Data Analysis, 71(0):681 – 693.
Ein-Dor, L., Zuk, O., and Domany, E. (2006). Thousands
of samples are needed to generate a robust gene list
for predicting outcome in cancer. Proceedings of the
National Academy of Sciences, 103(15):5923–5928.
Han, Y., Yang, Y., and Zhou, X. (2013). Co-regularized
ensemble for feature selection. In Proceedings of the
Twenty-Third International Joint Conference on Arti-
ficial Intelligence, IJCAI’13, pages 1380–1386. AAAI
Press.
Han, Y. and Yu, L. (2012). A variance reduction framework
for stable feature selection. Statistical Analysis and
Data Mining, 5(5):428–445.
Haury, A.-C., Gestraud, P., and Vert, J.-P. (2011). The influ-
ence of feature selection methods on accuracy, stabil-
ity and interpretability of molecular signatures. PLoS
ONE, 6(12):e28210.
Jain, A. K. and Chandrasekaran, B. (1982). 39 dimension-
ality and sample size considerations in pattern recog-
nition practice. Handbook of Statistics, 2:835–855.
Kuncheva, L. I. (2007). A stability index for feature selec-
tion. In Devedzic, V., editor, Artificial Intelligence and
Applications, pages 421–427. IASTED/ACTA Press.
Liu, H., Liu, L., and Zhang, H. (2010). Ensemble gene se-
lection by grouping for microarray data classification.
Journal of biomedical informatics, 43:81–87.
Meinshausen, N. and Bhlmann, P. (2010). Stability selec-
tion. Journal of the Royal Statistical Society: Series B
(Statistical Methodology), 72(4):417–473.
Rokach, L. (2010). Ensemble-based classifiers. Artificial
Intelligence Review, 33(1-2):1–39.
Saeys, Y., Abeel, T., and Peer, Y. (2008). Robust feature
selection using ensemble feature selection techniques.
In Daelemans, W., Goethals, B., and Morik, K., edi-
tors, Machine Learning and Knowledge Discovery in
Databases, volume 5212 of Lecture Notes in Com-
puter Science, pages 313–325. Springer Berlin Hei-
delberg.
Simon, R. (2003). Supervised analysis when the number of
candidate features (p) greatly exceeds the number of
cases (n). SIGKDD Explor. Newsl., 5(2):31–36.
Somol, P., Grim, J., and Pudil, P. (2009). Criteria ensembles
in feature selection. In Benediktsson, J. A., Kittler, J.,
and Roli, F., editors, MCS, volume 5519 of Lecture
Notes in Computer Science, pages 304–313. Springer.
Somol, P. and Novoviˇcov´a, J. (2010). Evaluating stability
and comparing output of feature selectors that opti-
mize feature subset cardinality. IEEE Trans. Pattern
Anal. Mach. Intell., 32(11):1921–1939.
Surowiecki, J. (2004). The Wisdom of Crowds: Why the
Many are Smarter Than the Few and how Collective
Wisdom Shapes Business, Economies, Societies, and
Nations. Doubleday.
Wald, R., Khoshgoftaar, T. M., and Dittman, D. J. (2013).
Ensemble gene selection versus single gene selec-
tion: Which is better? In Boonthum-Denecke, C.
and Youngblood, G. M., editors, FLAIRS Conference.
AAAI Press.
Yang, P., Hwa Yang, Y., B. Zhou, B., and Y. Zomaya, A.
(2010). A review of ensemble methods in bioinfor-
matics. Current Bioinformatics, 5(4):296–308.
ICPRAM2014-InternationalConferenceonPatternRecognitionApplicationsandMethods
330