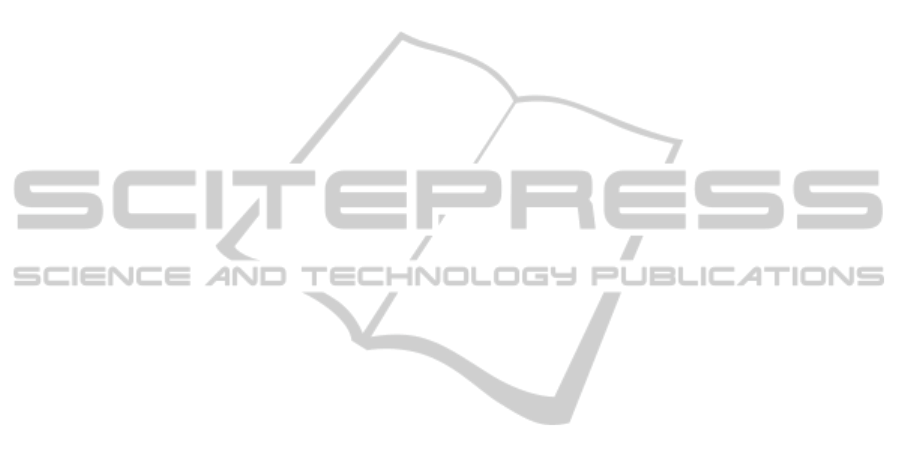
solve the optimizing problem with the GSMO algo-
rithm. Our method outperforms when compared with
other state-of-the art kernel clustering method. The
results are very encouraging and the proposed method
can be adapted to general clustering problems.
REFERENCES
Singh, K.K. and Singh, A. (2010). A Study of Image Seg-
mentation Algorithms for Different Types of Images.
In International Journal of Computer Science Issues,
vol. 7, no. 5.
Dehariya, V.K., Shrivastava, S.K. and Jain, R.C. (2010).
Clustering of Image Data Set Using K-Means and
Fuzzy K-Means Algorithms. In Computational Intel-
ligence and Communication Networks, pp. 386-391.
Filippone, M., Camastra, F., Masulli, F. and Rovetta, S.
(2008). A survey of kernel and spectral methods for
clustering. In Pattern Recognition, vol. 41, no. 1, pp.
176-190.
Schlkopf, B. and Smola, A.J. (2002). Learning with Ker-
nels. MIT Press Cambridge, MA, USA.
Mercer, J. (1909). Functions of positive and negative type
and their connection with the theory of integral equa-
tions. In Proceedings of the Royal Society of London,
vol. 83, no. 559, pp. 69-70.
Tzortzis, G.F. and Likas, A.C. (2009). The global kernel
k-means algorithm for clustering in feature space. In
IEEE Transactions on Neural Networks, vol. 20, no.
7, pp. 1181-1194.
Kannan, S.R., Ramathilagam, S., Devi, R. and Sathya, A.
(2011). Robust kernel FCM in segmentation of breast
medical images. In Expert Systems with Applications,
vol. 38, no. 4, pp. 4382-4389.
Tax, D.M.J. and Duin, R.P.W. (2004). Support vector data
description. In Machine Learning, vol. 54, no. 1, pp.
45-66.
Camastra, F. and Verri, A. (2005). A novel kernel method
for clustering. In IEEE transactions on Pattern Anal-
ysis and Machine Intelligence, vol. 27, no. 5, pp. 801-
805.
Chang, L., Deng, X.M., Zheng, S.W. and Wang, Y.Q.
(2008). Scaling up kernel grower clustering method
for large data sets via core-sets. In Acta Automatica
Sinica, vol. 34, no. 3, pp. 376-382.
Slimene, A. and Zagrouba, E. (2011). A new PSO based
kernel clustering method for image segmentation. In
SITIS, Signal Image Technology and Internet Based
Systems, pp. 350-357 .
Park, J., Kang, D., Kim, J., Kwok, J.T. and Tsang, I.W.
(2007). SVDD-Based Pattern Denoising. In Neural
Computation, vol. 19, no. 7, pp.1919-1938.
Lee, S.W. and Park, J. (2006). Low resolution face recog-
nition based on support vector data description. In
Pattern Recognition, vol. 39, no. 9, pp. 1809-1812.
Banerjee, A., Burlina, P. and Meth, R. (2007). Fast Hyper-
spectral Anomaly Detection via SVDD. In IEEE In-
ternational Conference on Image Processing, vol. 4,
pp. 101-104.
Chu, C.S., Tsang, I.W. and Kwok, J.T. (2004). Scaling up
support vector data description by using core-sets. In
IEEE International Joint Conference on Neural Net-
works, vol. 1, pp. 425-430.
Kumar, P., Mitchell, J.S.B. and Yildirim, E.A. (2003). Ap-
proximate Minimum Enclosing Balls in High Dimen-
sions Using Core-Sets. In Experimental Algorithmics,
vol.8, no.1, pp. 1.
Bishop, C. (1995). Neural Networks for Pattern Recogni-
tion. Oxford University Press, USA.
Forghani, Y., Effati, S., Yazdi, H.S. and Tabrizi, R.S. (2011).
Support vector data description by using hyper-ellipse
instead of hyper-sphere. In International Conference
on Computer and Knowledge Engineering, pp. 22-27.
Platt, J.C., (1999). Fast training of support vector machines
using sequential minimal optimization. In Advances
in Kernel Methods: Support Vector Learning, pp. 185-
208.
Keerthi, S.S. (2002). Convergence of a Generalized SMO
Algorithm for SVM Classifier Design. In Machine
Learning, vol. 46, pp. 351-360.
Kumar, P. and Yildirim, E.A. (2005). Minimum-Volume
Enclosing Ellipsoids and Core Sets. In Optimization
theory and applications, vol. 126, no. 1, pp. 1-21.
Frank, A. and Asuncion, A. (2010).
Mldata, Machine Learning Data set Repository. (2009).
Martin, D., Fowlkes, C., Tal, D. and Malik, J. (2001).
A Database of Human Segmented Natural Images
and Its Application to Evaluating Segmentation Algo-
rithms and Measuring Ecological Statistics.
Unnikrishnan, R., Pantofaru, C. and Hebert, M. (2007). To-
ward objective evaluation of image segmentation al-
gorithms. IEEE Transactions on pattern analysis and
machine intelligence, vol. 29, no. 6, pp. 929-944.
ImprovingKernelGrowerMethodsusingEllipsoidalSupportVectorDataDescription
349