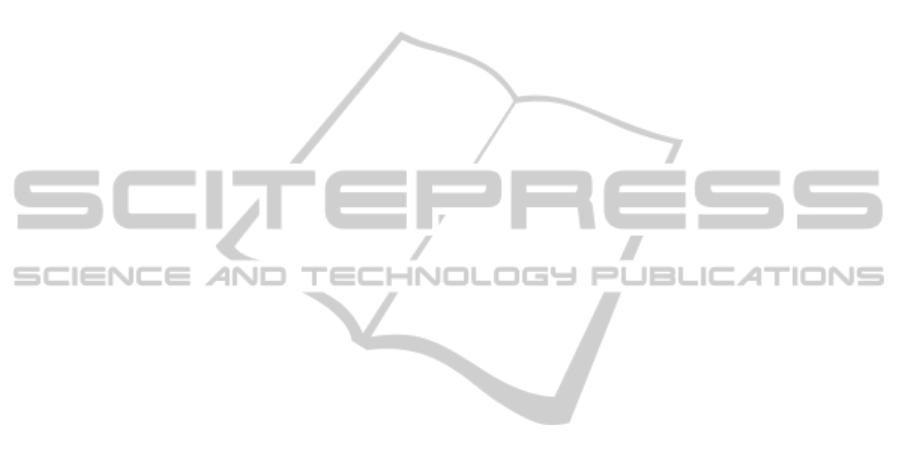
inexpensive to take advantage of customer
flexibility.
In earlier research (Akgun et al., 2011; 2012;
2013) we have shown the benefit of partial
flexibility in homogeneous systems, in which
different groups of servers are stochastically
identical. More work needs to be done, in terms of
investigating the performance of, and developing
protocols for, systems with heterogeneous server
stations and user populations.
2 RESULTS FOR
HOMOGENEOUS SERVERS
If flexible customers are given the option to choose
which queue to join based on queue length, they
would clearly join the shortest queue (JSQ)
assuming server stations are homogeneous, service
times are exponential, and the service discipline is
FCFS. My co-authors and I showed the optimality of
JSQ (join-the-shortest-queue) routing in a very
strong, sample-path, sense (Akgune et al., 2011).
The system is modelled as a queuing system with c
parallel multiple-server stations that have
exponentially distributed service times with the
same service rate μ. All of these servers follow a
nonidling but otherwise arbitrary service discipline
(FCFS, LCFS, etc.). Arrivals to the system form an
arbitrary process that is independent of the state of
the system. Some (dedicated) arrivals are obliged to
use a particular station, while others (flexible) have
the ability to use any of the c stations (or, more
generally, they can use an arbitrary subset of size at
least two of the stations. Dedicated arrivals are
equally likely to require any particular station, so the
arrival process is homogeneous across stations. Let
A be the set of arrival points, and let F
⊆
A denote
the time points where a flexible arrival occurs. Note
that F is an arbitrary subset of A.
We used weak majorization and developed a new
approach for coupling potential service completions
to prove the optimality of JSQ (join-the-shortest-
queue) in the sample path sense. We also showed
that when flexible customers follow JSQ, the total
number of customers in the system is stochastically
decreasing in the proportion of flexible customers,
so there is a strong advantage to having customer
flexibility. Note that minimizing the total number in
the system is equivalent to minimizing the mean
waiting time from Little’s law. We also showed that
the waiting time for dedicated customers is
decreasing in the proportion of flexible customers.
That is, the monolingual customers, on average,
benefit from having bilingual customers.
We also considered several practically important
extensions. For example, suppose customers may
abandon, but they only abandon from the queue (a
reasonable assumption for, e.g., a call center), and
suppose the abandonment rate is greater than the
service rate. We showed, under these abandonment
assumptions, that JSQ no longer minimizes the
number of customers in the system, but it still
maximizes the service completion process. Other
extensions that we considered included finite
buffers, resequencing, random yields, and randomly
varying service rates.
While in Akgun et al., (2011) we showed that
stationary waiting time is stochastically decreasing
in the proportion of flexible customers, p, in Akgun,
Righter, and Wolff (2012) we studied the marginal
impact of customer flexibility, that is, the convexity
of waiting time in p. Convexity means that the
marginal advantage of flexibility is largest at small
proportions. That is, roughly, “a little bit of
flexibility goes a long way.” Unfortunately, it is not
possible to obtain convexity in the strong sense for
which monotonicity holds. We considered a
modified model, which we called the inventory
model, where we obtained a sample-path convexity
result using majorization of the queue lengths. In
this model, there are two servers and they never idle
but instead build up inventory when no customers
are waiting. This may be reasonable in production
environments where demand is high and where it is
expensive to idle machines, e.g., due to high
backorder costs or server shutdown costs. Although
convexity in p is intuitive for our original model, in
which servers idle when they have no customers, it
does not hold in the same strong sense that
monotonicity holds, and it is surprisingly difficult to
prove. We developed a new approach that combines
marginal analysis with coupling to show that the
stationary mean waiting time is convex in p. We
considered a tagged customer in steady-state that has
lowest preemptive priority relative to the other
customers so that the other customers are unaffected
by the tagged customer. We showed that the
derivative of the stationary waiting time with respect
to p (the marginal value of customer flexibility) can
be expressed in terms of the difference in expected
waiting time between going to the long and the short
queue for the tagged customer. We then showed,
using another coupling argument, that this difference
is decreasing in p.
Now consider a slightly different policy, where
flexible customers “virtually” join all of the queues
ICORES2014-InternationalConferenceonOperationsResearchandEnterpriseSystems
302