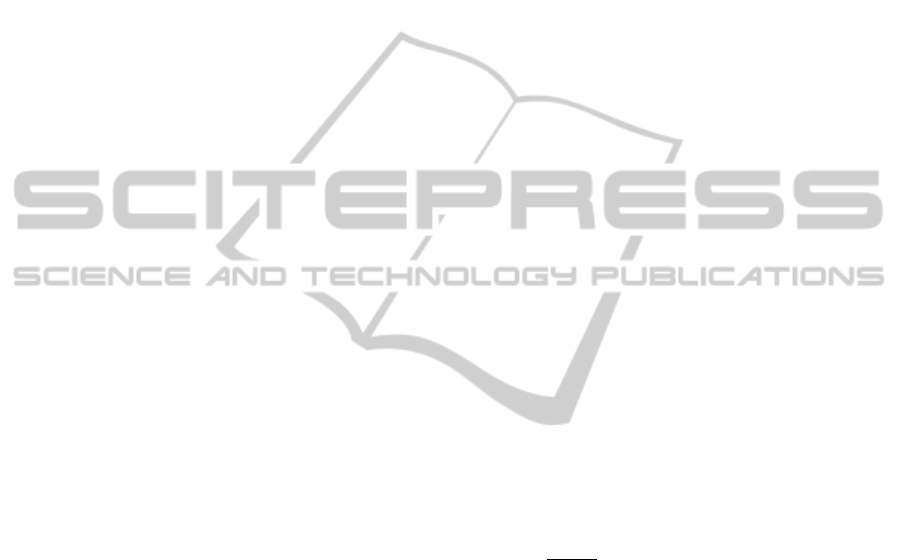
Simchi-Levi, 2012); (Yano and Gilbert, 2005);
(Elmaghraby and Keskinocak, 2003); (Petruzzi and
Dada, 1999). In general, the basic choices in joint
price-demand and inventory models are identified as
the following set of structural attributes. Primarily,
the structure of the chosen price-demand model
plays a significant role in the setting of the problem
and the optimal policy obtained. Other attributes are:
(i) incorporating capacity restrictions on the quantity
ordered; (ii) using cost structures like fixed ordering
costs or price adjustment costs; (iii) incorporating
pricing policies like static pricing where the price is
fixed for a few periods once it is set; (iv) the
structure of the inventory replenishment policy,
namely the availability of multiple replenishments in
the planning period or a single replenishment like in
revenue management; (v) the number of planning
periods being single or multiple; (vi) the number of
products being single or multiple; and (vii) the
structure of the supply chain being competitive,
coordinated or cooperative. As for the latter, the
perspective of the model is essential: does it support
the buyer, the seller, or the system as a whole.
In this study our aim is to explore the existing
price-demand models that have been applied in
inventory and production management so far and to
identify new potential structures that may have been
applied in marketing research but have not been
touched yet in inventory and production research. In
particular, our focus will be on dynamic pricing
structures for competing products, since our
exploration so far revealed that they have not been
studied exhaustively in price-demand inventory
literature.
As summarized in (Chen and Simchi-Levi,
2012), the most common price-demand structure
used in joint price-demand-inventory models is the
linear demand
,∈
0,
⁄
,
0,b0 where demand
is a linearly
decreasing function of price . In spite of its
computational simplicity, this structure is questioned
in terms of its validity due to the linearity
assumption and possibility of obtaining negative
demands at high price levels. At this point, the
exponential demand structure
exp
,0,0 is introduced as an applicable
alternative. The third common structure is the iso-
elastic demand
,0,1 where
the demand elasticity is the same for all price
levels. On the other hand, the logit demand structure
exp
/1exp
is an
alternative to all of the above structures by allowing
a fixed potential demand which is multiplied by
the probability of buying a product at a price . It
should be noted that all these models can be
extended to reflect the effect of complement or
substitute products’ prices on the demand of a
certain product in a competitive environment.
In a stochastic setting, random demand
,
is
defined as a function of the price and a random
noise . Standard approaches include the additive
model
,
, with
0, and the
multiplicative model
,
,with
0and)1. Yet, there are hybrid structures
of the additive and multiplicative models (Chen and
Simchi-Levi, 2012).
Moreover, we have the price-demand models
with intertemporal effect, i.e., the models that
incorporate the effect of prices in the previous
periods on the current demand of an item. Ahn, et al.
(2007) and Gümüş and Kaminsky (2010) provide
models with substitute products and multiple periods
where the total demand in a period is the sum of the
linear demand function of price, the intertemporal
effect, and the substitution effect.
In addition to the price-demand models
mentioned above, marketing theory includes several
other approaches. Among these, market share
attraction (MSA) models are used to calculate the
demand of a product in competitive environments
moderated by 1 substitute products. Assuming
that there is a fixed potential demand
, the demand
of substitute product ,
, for
1,2,…, is obtained by multiplying by the market
share
of product . Here, the market share
is
defined as the ratio of the so-called attraction
of
product with the total attractions of all products,
i.e.,
∑
. Depending on how the attraction
caused by the price of an item is formulated, MSA
models can have different forms (Lilien et al., 1992).
The above MSA approach is a good example of a
technique that has proven its value in marketing
theory, but is still of limited importance when it
comes to price-demand inventory and production
models.
Aim of this positioning paper is to unfold a line
of research to change this. Specifically, we propose
a price-demand-inventory model framework for
optimizing joint pricing, production, inventory and
transportation decisions in a supply chain with
multiple products, multiple factories, and multiple
markets operating in multiple periods. The factories
operate in a cooperative environment where these
decisions are given centrally so as to maximize the
total profit. Competition between the various
product types is achieved centrally by setting market
specific prices for each product type in each market
ICORES2014-InternationalConferenceonOperationsResearchandEnterpriseSystems
318