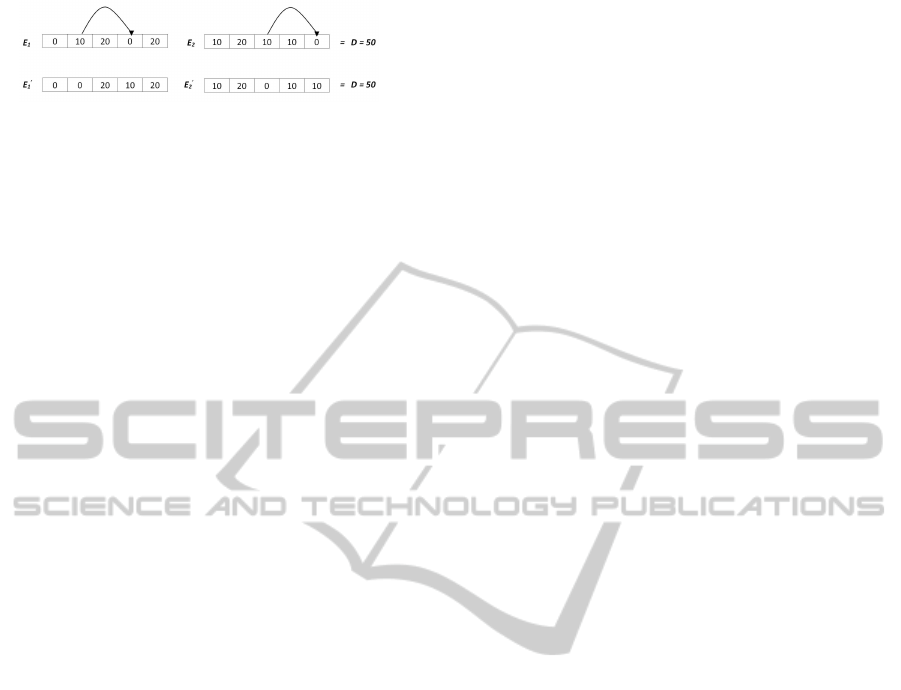
Figure 3: Example of mutation operator.
5 CONCLUSIONS
In this position paper, we rely on a two echelon sup-
ply chain problem dealing with a supplier selection
issue in order to resolve it based on an Evolutionary
Algorithm adapted to dynamic optimization. Our aim
is to find a response for how to change the set of sup-
pliers during time. Based on the optimum behavior
after each change, we proceed to select suppliers for
each sub-period. Given the dynamic parameters of the
problem and its complexity, the choice of a solver for
the resolution may be inadequate for medium to large
size problems. Hence, the choice to search for an
approximate solution seems appropriate in this case.
For further studies, we are planning to extend and im-
plement the problem to make in consideration more
operations on supply chain related to forecasts of or-
ders, inventories and adding other actors (distributors,
retailers, etc.). An algorithm-based memory that de-
tects changes and keeps the best individuals over time
can converge quickly to the best solution as it was
demonstrated in many Benchmarks. In addition other
approach adapted for dynamic optimization problem,
like anticipation, need to be developed if we want to
take into account further operations in a global supply
chain.
ACKNOWLEDGEMENTS
This research is supported by the European com-
munity related to the region of Auvergne in France,
through the project LABEX IMOBS3. These sup-
ports are gratefully acknowledged.
REFERENCES
Bosman, L. P. (2007). Learning and anticipation in on line
dynamic optimization. Studies in computational intel-
ligence, 51:129–152.
Cruz, C., Gonz
´
alez, J. R., and Pelta, D. a. (2010). Optimiza-
tion in dynamic environments: a survey on problems,
methods and measures. Soft Computing, 15(7):1427–
1448.
Deng, X., Hu, Y., Deng, Y., and Mahadevan, S. (2014).
Supplier selection using AHP methodology extended
by D numbers. Expert Systems with Applications,
41(1):156–167.
Ding, H., Benyoucef, L., and Xie, X. (2005). A simulation
optimization methodology for supplier selection prob-
lem. International Journal of Computer Integrated
Manufacturing, 18(2-3):210–224.
Ghodsypour, S. and O’Brien, C. (1998). A decision sup-
port system for supplier selection using an integrated
analytic hierarchy process and linear programming.
International Journal of Production Economics, 56-
57:199–212.
Goldberg, D. (1989). Genetic Algorithm In Search, Op-
timization And Machine Learning. Addison-Wesley,
Reading, MA.
Kristianto, Y., Gunasekaran, A., Helo, P., and Sandhu, M.
(2012). A decision support system for integrating
manufacturing and product design into the reconfigu-
ration of the supply chain networks. Decision Support
Systems, 52(4):790–801.
Lee, H. L. (2004). The triple-A supply chain. Harvard
business review, 82(10):102–12, 157.
Lepagnot, J., Nakib, A., Oulhadj, H., and Siarry, P. (2010).
A new multiagent algorithm for dynamic continuous
optimization. International Journal of Applied Meta-
heuristic Computing, 1(1):16–38.
Nazari-Shirkouhi, S., Shakouri, H., Javadi, B., and Kera-
mati, A. (2013). Supplier selection and order alloca-
tion problem using a two-phase fuzzy multi-objective
linear programming. Applied Mathematical Mod-
elling, 37(22):9308–9323.
Nguyen, T. T., Yang, S., and Branke, J. (2012). Evolution-
ary dynamic optimization: A survey of the state of the
art. Swarm and Evolutionary Computation, 6:1–24.
Oh, S., Ryu, K., and Jung, M. (2011). Reconfiguration
framework of a supply network based on flexibility
strategies. Computers & Industrial Engineering.
Osman, H. and Demirli, K. (2010). A bilinear goal pro-
gramming model and a modified Benders decompo-
sition algorithm for supply chain reconfiguration and
supplier selection. International Journal of Produc-
tion Economics, 124(1):97–105.
Pillac, V., Gendreau, M., Gu
´
eret, C., and Medaglia, A. L.
(2013). A review of dynamic vehicle routing prob-
lems. European Journal of Operational Research,
225(1):1–11.
Ryu, K. and Jung, M. (2003). Agent-based fractal archi-
tecture and modelling for developing distributed man-
ufacturing systems. International Journal of Produc-
tion Research, 41(17):4233–4255.
Ware, N. R., Singh, S., and Banwet, D. (2014). A mixed-
integer non-linear program to model dynamic supplier
selection problem. Expert Systems with Applications,
41(2):671–678.
Wu, W.-Y., Sukoco, B. M., Li, C.-Y., and Chen,
S. H. (2009). An integrated multi-objective
decision-making process for supplier selection with
bundling problem. Expert Systems with Applications,
36(2):2327–2337.
DealingwithVariationsforaSupplierSelectionProbleminaFlexibleSupplyChain-ADynamicOptimizationApproach
327