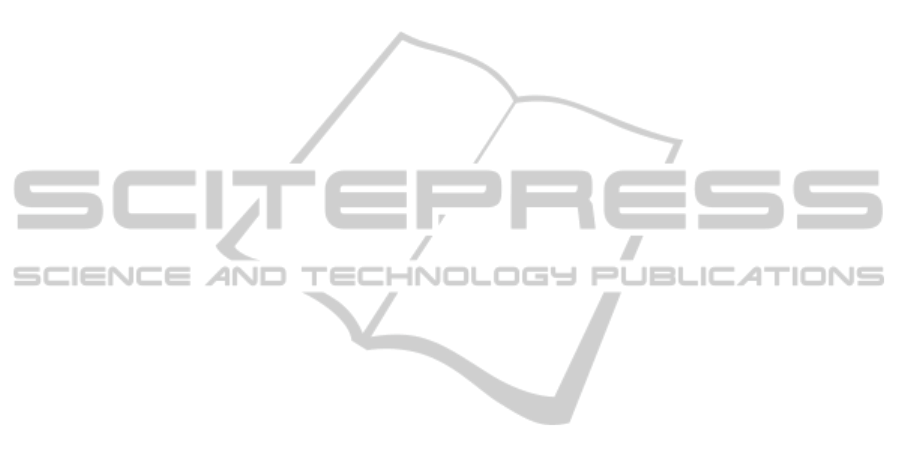
seems to be successfully identify even novel miR-
NAs for breast cancer still there are many question
to be answered. MiRNA networks or miRNA simi-
larity can be defined in other ways such as comparing
the sequence or based on single nucleotide polymor-
phisms (SNP) or by using conservation scores. After
a thorough investigation of the target prediction algo-
rithms we can include predicted miRNA-target pairs
in the analysis to increase the number of miRNAs in
the study. Last with the growing number of avail-
able data it will be possible soon to build more tissue
specific miRNA networks. This way we will have a
heterogeneous data source and we can make a good
use of the MKL method as it can be used to com-
pare the importance of the different similarity scores
or data sources based on the learned weights of the
kernels. Future work will concentrate on answering
these questions.
REFERENCES
Aerts, S., Lambrechts, D., Maity, S., Van Loo, P., Coessens,
B., De Smet, F., Tranchevent, L., De Moor, B., Mary-
nen, P., Hassan, B., Carmeliet, P., and Moreau, Y.
(2006). Gene prioritization through genomic data fu-
sion. Nature Biotechnology, 24(5):537–544.
Arany, A., Bolg´ar, B., Balogh, B., Antal, P., and M´atyus,
P. (2013). Multi-aspect candidates for repositioning:
Data fusion methods using heterogeneous information
sources. Current Medicinal Chemistry, 20(1):95–107.
Bolg´ar, B., Arany, A., Temesi, G., Balogh, B., Antal, P., and
M´atyus, P. (2013). Drug repositioning for treatment
of movement disorders: from serendipity to rational
discovery strategies. CURRENT TOPICS IN MEDIC-
INAL CHEMISTRY, 13(18):2337–63.
Bornigen, D., Tranchevent, L., Bonachela-Capdevila, F.,
Devriendt, K., De Moor, B., De Causmaecker, P., and
Moreau, Y. (2012). An unbiased evaluation of gene
prioritization tools. Bioinformatics, 28(23):3081–
3088.
Burbidge, R., Trotter, M., Buxton, B., and Holden, S.
(2001). Drug design by machine learning: sup-
port vector machines for pharmaceutical data analysis.
Comput. Chem. (Oxford, U. K.), 26(1):5–14.
Chen, K. and Rajewsky, N. (2007). The evolution of gene
regulation by transcription factors and micrornas. Nat
Rev Genet, 8(2):93–103.
De Bie, T., Tranchevent, L.-C., Van Oeffelen, L. M., and
Moreau, Y. (2007). Kernel-based data fusion for gene
prioritization. Bioinformatics, 23(13):i125–i132.
Eckert, H. and Bajorath, J. (2007). Molecular similarity
analysis in virtual screening: foundations, limitations
and novel approaches. Drug Discovery Today, 12(5-
6):225–233.
Ficarra, E. et al. (2012). One decade of development and
evolution of microrna target prediction algorithms.
Genomics, proteomics & bioinformatics.
Freudenberg, J. and Propping, P. (2002). A similarity-
based method for genome-wide prediction of disease-
relevant human genes. Bioinformatics, 18:S110–
S115.
Ginn, C., Turner, D., Willett, P., Ferguson, A., and Her-
itage, T. (1997). Similarity searching in files of three-
dimensional chemical structures: Evaluation of the
eva descriptor and combination of rankings using data
fusion. J. Chem. Inf. Model., 37(1):23–37.
Ginn, C., Willett, P., and Bradshaw, J. (2000). Combina-
tion of molecular similarity measures using data fu-
sion. Perspect. Drug Discovery Des., 20(1):1–16.
Hsu, S.-D., Lin, F.-M., Wu, W.-Y., Liang, C., Huang, W.-
C., Chan, W.-L., Tsai, W.-T., Chen, G.-Z., Lee, C.-J.,
Chiu, C.-M., et al. (2011). mirtarbase: a database cu-
rates experimentally validated microrna–target inter-
actions. Nucleic acids research, 39(suppl 1):D163–
D169.
Iacucci, E., Tranchevent, L., Popovic, D., Pavlopoulos, G.,
De Moor, B., Schneider, R., and Moreau, Y. (2012).
Reliance: a machine learning and literature-based pri-
oritization of receptor-ligand pairings. Bioinformat-
ics, 28(18):I569–I574.
Jacobsen, A., Silber, J., Harinath, G., Huse, J. T., Schultz,
N., and Sander, C. (2013). Analysis of microrna-target
interactions across diverse cancer types. Nature struc-
tural & molecular biology.
Johnson, M. and Maggiori, G. (1990). Concepts and appli-
cations of molecular similarity. Wiley.
Kim, K., Chadalapaka, G., Lee, S., Yamada, D., Sastre-
Garau, X., Defossez, P.-A., Park, Y.-Y., Lee, J.-S., and
Safe, S. (2011). Identification of oncogenic microrna-
17-92/zbtb4/specificity protein axis in breast cancer.
Oncogene, 31(8):1034–1044.
Kohler, S., Bauer, S., Horn, D., and Robinson, P. (2008).
Walking the interactome for prioritization of candi-
date disease genes. American Journal of Human Ge-
netics, 82(4):949–958.
Lanckriet, G., De Bie, T., Cristianini, N., Jordan, M.,
and Noble, W. (2004). A statistical framework for
genomic data fusion. Bioinformatics, 20(16):2626–
2635.
Lee, I., Blom, U., Wang, P., Shim, J., and Marcotte,
E. (2011). Prioritizing candidate disease genes by
network-based boosting of genome-wide association
data. Genome Research, 21(7):1109–1121.
Li, X., Wang, Q., Zheng, Y., Lv, S., Ning, S., Sun, J.,
Huang, T., Zheng, Q., Ren, H., Xu, J., Wang, X.,
and Li, Y. (2012). Prioritizing human cancer mi-
crornas based on genes? functional consistency be-
tween microrna and cancer. Nucleic Acids Research,
40(16):7653–7665.
Liekens, A., De Knijf, J., Daelemans, W., Goethals, B.,
De Rijk, P., and Del-Favero, J. (2011). Biograph: un-
supervised biomedical knowledge discovery via auto-
mated hypothesis generation. Genome Biology, 12(6).
Moreau, Y. and Tranchevent, L. (2012). Computational
tools for prioritizing candidate genes: boosting dis-
ease gene discovery. Nature Reviews Genetics,
13(8):523–536.
BIOINFORMATICS2014-InternationalConferenceonBioinformaticsModels,MethodsandAlgorithms
284