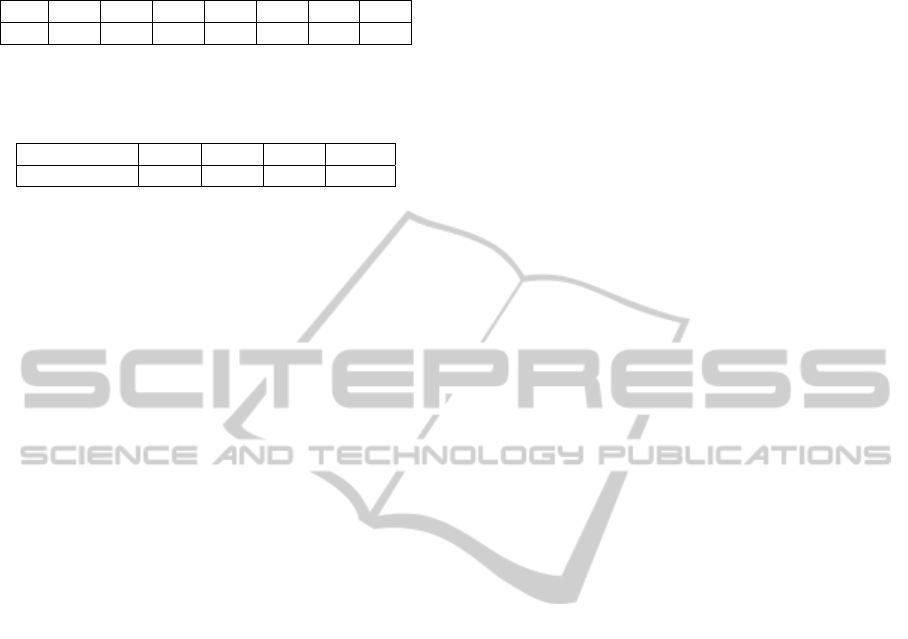
Table 3: Accuracy rate (ACC) as a function of the Number
of Bins (NB) in the case of holistic (global) method.
BN 2 5 10 20 30 50 100
ACC 56.63 63.63 64.36 64.61 64.52 64.19 61.19
Table 4: Accuracy rate for the four bin number estimation
rules: SC, ST, FD, LMSE in the case of holistic (global)
method
Rule SC ST FD LMSE
Accuracy rate 66.11 64.52 66.52 65.36
Table 4 shows that the FD method gives the best
results with 2 points more with respect to the best
result obtained by exploring different fixed BN
values displayed in Table 3. This proves that
searching for an optimal NB can be attractive
practically. The results also show that better
accuracy recognition rates can be achieved with data
driven bin number choices rather than fixed bin
numbers. Indeed, the rule proposed by Sturges only
depends on the sample number N and this is
equivalent to a fixed bin number since all the images
have the same size (112x92 samples). The bin
number choice for the other approaches is data
driven since the numbers either depend on the
standard deviation (SC), the IQR (FD) and the
correlation coefficient and standard deviation
(LMSE).
Since the LMSE method gives an optimal BN
that depends on the correlation parameter between
the data (the correlation is not taken into account
with the three other methods SC, ST, FD), the
accuracy rate for this method should be better since
intra class images are highly correlated. However,
the LMSE method assumes normal distribution of
the data. This hypothesis is not fulfilled with the
pixel distribution of the image strips of the full
image.
However, it should be noticed that the
computational cost for the LMSE method is far
lower than the FD method.
5 CONCLUSIONS
In many works in the literature dealing with face
recognition, local methods are shown to be
preferable than global ones (Hermosillo and
Faugeras, 2001). However, many databases need
alignment procedures. This task is often difficult to
achieve. In this paper, we show that without the
alignment procedure, holistic methods are better
than local ones with the ORL database using MI
based similarity measures.
The automatic BN searching procedure on the data
tended to improve the accuracy rate in a recognition
faces system based on MI histogram estimations.
The searching procedure is based on criteria
which are sensitive to the statistical properties of the
data. Adapting the criterion to the exact properties of
the data constitutes a perspective of this study.
Other works will be investigated using more
“difficult” datasets including effects of illumination
variation, pose, and facial expressions. A deeper
comparison of local and global approaches will be
carried out using such databases (e.g. the extended
Yale face database B).
REFERENCES
Ait Kerroum, M., Hammouch, A. and Aboutajdine, D.
(2010) 'Textural feature selection by joint mutual
information based on Gaussian mixture model for
multispectral image classification', Pattern
Recognition Letters, vol. 31, no. 10, July, pp. 1168-
1174.
Cover, T. M. and Thomas, J. A. (2006) Elements of
information theory, 2
nd
edition, Wiley Series in
telecommunications and Signal Processing.
Darbellay, G. A. and Vajda, I. (1999) 'Estimation of the
information by an adaptive partitioning of the
observations space', IEEE Transactions on
Information Theory, vol. 45, no. 4, May, pp. 1315–
1321.
Drugman, T., Gurban, M. and Thiran, J. P. (October 2007)
'Relevant Feature Selection for Audio-visual Speech
recognition', Proc. of the International Workshop on
Multimedia Signal Processing, Crete, Greece, 179 -
182.
Freedman, D. and Diaconis, P. (1981) 'On the Maximum
Deviation Between the Histogram and the Underlying
Density', Zeitschrift fur Wahrscheinlichkeitstheorie
und verwandte Gebiete, vol. 58, no. 2, pp. 139-167.
Hacine-Gharbi, A., Deriche, M., Ravier, P., Harba, R. and
Mohamadi, T. (2013) 'New Histogram-based
Estimation Technique of Entropy and Mutual
Information using Mean Squared Error Minimization',
Computers and Electrical Engineering , vol. 39, no. 3,
pp. 918–933.
Hacine-Gharbi, A., Ravier, P., Harba, R. and Mohamadi,
T. (2012) 'Low bias histogram-based estimation of
mutual information for feature selection', Pattern
Recognition Letters, vol. 33, no. 10, pp. 1302–1308.
Hermosillo, G. and Faugeras, O. (2001) 'Dense image
matching with global and local statistical criteria: a
variational approach', Computer Vision and Pattern
Recognition, vol. 1, pp. 73–78.
Jain, A. K., Duin, R. P. W. and Mao, J. (2000) 'Statistical
Pattern Recognition: A Review', IEEE Transactions
on Pattern Analysis and Machine Intelligence, vol. 22,
no. 1, pp. 4-37.
OntheBinNumberChoiceofJointHistogramEstimationAppliedtoMutualInformationbasedFaceRecognition
781