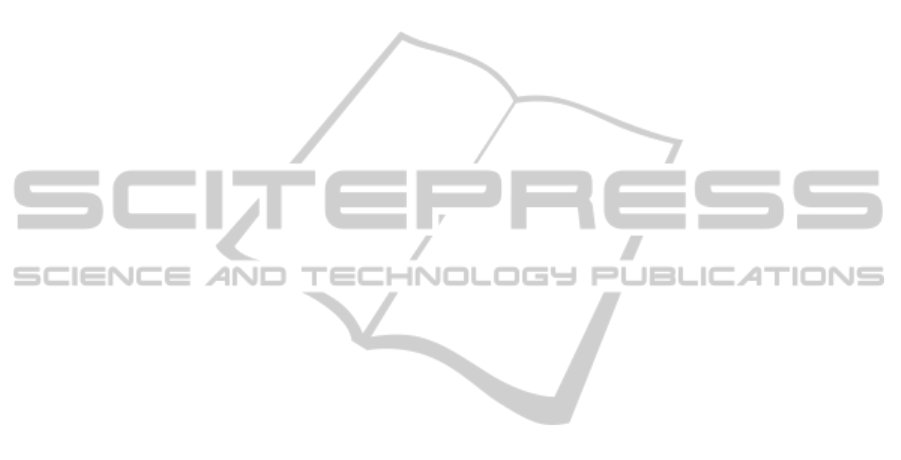
We think that our iRDA framework can have the
capacity of finding small size gene signatures with a
potentially high predictive power that, in turn, could
disclose biological information regarding gene
synergy.
REFERENCES
Albrecht, A., Vinterbo, S. A. & Ohno-Machado, L. 2003.
An Epicurean learning approach to gene-expression
data classification. Artificial Intelligence in Medicine,
28, 75-87.
Alon, U., Barkai, N., Notterman, D. A., Gish, K., Ybarra,
S., Mack, D. & Levine, A. J. 1999. Broad patterns of
gene expression revealed by clustering analysis of
tumor and normal colon tissues probed by
oligonucleotide arrays. Proceedings of the National
Academy of Sciences, 96, 6745-6750.
Bell, D. A. & Wang, H. 2000. A formalism for relevance
and its application in feature subset selection. Machine
Learning, 41, 175-195.
Brown, G., Pocock, A., Zhao, M.-J. & Luj N, M. 2012.
Conditional likelihood maximisation: A unifying
framework for information theoretic feature selection.
The Journal of Machine Learning Research, 13, 27-66.
Coussens, L. M., Zitvogel, L. & Palucka, A. K. 2013.
Neutralizing tumor-promoting chronic inflammation: a
magic bullet? Science, 339, 286-291.
Cover, T. M. & Thomas, J. A. 2012. Elements of
Information Theory, John Wiley & Sons.
Davies, S. & Russell, S. NP-completeness of searches for
smallest possible feature sets. Proceedings of the 1994
AAAI Fall Symposium on Relevance, 1994. 37-39.
Ding, C. & Peng, H. 2005. Minimum redundancy feature
selection from microarray gene expression data.
Journal of Bioinformatics and Computational Biology,
3, 185-205.
Ein-Dor, L., Zuk, O. & Domany, E. 2006. Thousands of
samples are needed to generate a robust gene list for
predicting outcome in cancer. Proceedings of the
National Academy of Sciences, 103, 5923-5928.
Fleuret, F. 2004. Fast binary feature selection with
conditional mutual information. The Journal of
Machine Learning Research, 5, 1531-1555.
Gheyas, I. A. & Smith, L. S. 2010. Feature subset
selection in large dimensionality domains. Pattern
Recognition, 43, 5-13.
Guyon, I., Weston, J., Barnhill, S. & Vapnik, V. 2002.
Gene selection for cancer classification using support
vector machines. Machine Learning, 46, 389-422.
Kim, S.-Y. 2009. Effects of sample size on robustness and
prediction accuracy of a prognostic gene signature.
BMC Bioinformatics, 10, 147.
Kohavi, R. & John, G. H. 1997. Wrappers for feature
subset selection. Artificial Intelligence, 97, 273-324.
Lai, H.-M., Albrecht, A. & Steinhofel, K. 2013. Gene
selection guided by feature interdependence. World
Academy of Science, Engineering and Technology
(WASET), 1432-1438.
Lazar, C., Taminau, J., Meganck, S., Steenhoff, D.,
Coletta, A., Molter, C., De Schaetzen, V., Duque, R.,
Bersini, H. & Now , A. 2012. A survey on filter
techniques for feature selection in gene expression
microarray analysis. IEEE/ACM Transactions on
Computational Biology and Bioinformatics (TCBB), 9,
1106-1119.
Mundra, P. A. & Rajapakse, J. C. 2010. SVM-RFE with
MRMR filter for gene selection. NanoBioscience,
IEEE Transactions on, 9, 31-37.
Nevins, J. R. & Potti, A. 2007. Mining gene expression
profiles: expression signatures as cancer phenotypes.
Nature Reviews Genetics, 8, 601-609.
Nutt, C. L., Mani, D., Betensky, R. A., Tamayo, P.,
Cairncross, J. G., Ladd, C., Pohl, U., Hartmann, C.,
Mclaughlin, M. E. & Batchelor, T. T. 2003. Gene
expression-based classification of malignant gliomas
correlates better with survival than histological
classification. Cancer Research, 63, 1602-1607.
Saeys, Y., Inza, I. & Larra Aga, P. 2007. A review of
feature selection techniques in bioinformatics.
Bioinformatics, 23, 2507-2517.
Subramanian, A., Tamayo, P., Mootha, V. K., Mukherjee,
S., Ebert, B. L., Gillette, M. A., Paulovich, A.,
Pomeroy, S. L., Golub, T. R. & Lander, E. S. 2005.
Gene set enrichment analysis: a knowledge-based
approach for interpreting genome-wide expression
profiles. Proceedings of the National Academy of
Sciences, 102, 15545-15550.
Wang, J., Duncan, D., Shi, Z. & Zhang, B. 2013. WEB-
based GEne SeT AnaLysis Toolkit (WebGestalt):
update 2013. Nucleic Acids Research, 41, W77-W83.
Yu, L. & Liu, H. 2004. Efficient feature selection via
analysis of relevance and redundancy. The Journal of
Machine Learning Research, 5, 1205-1224.
Zhou, X. & Tuck, D. P. 2007. MSVM-RFE: extensions of
SVM-RFE for multiclass gene selection on DNA
microarray data. Bioinformatics, 23, 1106-1114.
ICAART2014-InternationalConferenceonAgentsandArtificialIntelligence
220