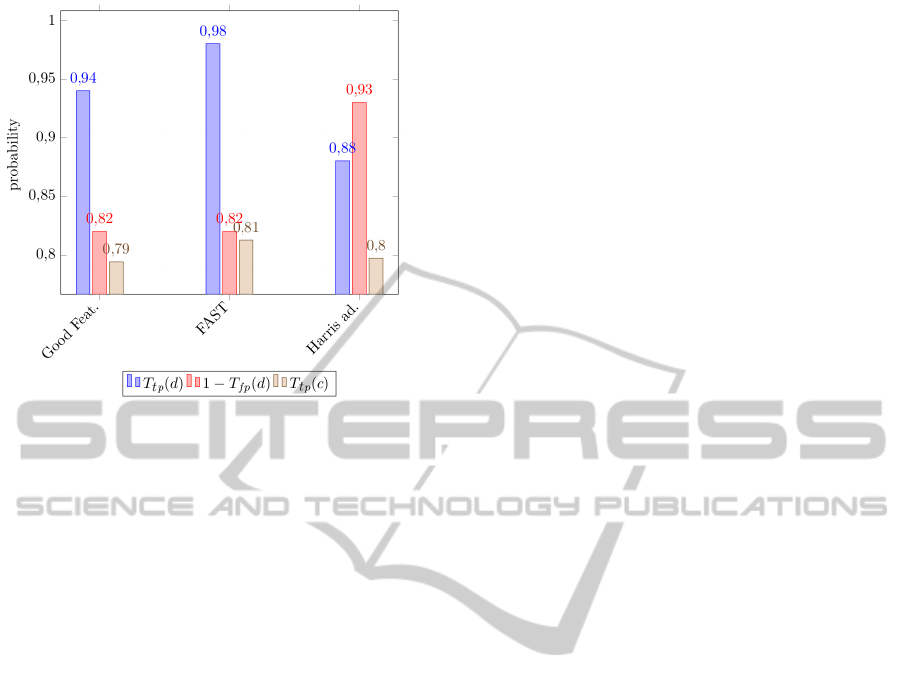
Figure 14: True positive rate (T
t p
(d)) and specificity (1 −
T
t p
(d)) for detection and true positive rate for classification
(T
t p
(c)).
detection of gestural reactions within the eyes region
was developed in previous research, but interest op-
erator analysis for motion detection was not studied
in detail. This paper analyzes different methods for
the selection of the interest points, determines the best
configuration parameters for each one of them, and it
also analyzes its behavior according to different clas-
sifiers. Results obtained with new interest points de-
tectors surpass the previous approach in terms of ac-
curacy.
In clinical terms, the choice of a suitable interest
points detector for this domain may improve the accu-
racy in the detection and interpretation of the gestural
reactions.
Future works will involve an extension of the
training dataset so a robust classifier can be trained
with the configurations established by this work. This
classifier may then by applied over the video se-
quences in order to detect the relevant movements
and, thus, serve to assist the audiologists.
ACKNOWLEDGEMENTS
This research has been partially funded by Ministerio
de Ciencia e Innovacin of the Spanish Government
through the research projects TIN2011-25476.
REFERENCES
Bay, H., Ess, A., Tuytelaars, T., and Van Gool, L. (2008).
Speeded-up robust features (surf). Comput. Vis. Image
Underst., 110(3):346–359.
Bouguet, J.-Y. (2000). Pyramidal implementation of the
Lucas-Kanade feature tracker: Description of the al-
gorithm. Intel Corporation, Microprocessor Research
Labs.
Chew, S., Rana, R., Lucey, P., Lucey, S., and Sridharan, S.
(2012). Sparse temporal representations for facial ex-
pression recognition. In Ho, Y.-S., editor, Advances in
Image and Video Technology, volume 7088 of Lecture
Notes in Computer Science, pages 311–322. Springer
Berlin Heidelberg.
Davis, A. (1989). The prevalence of hearing impairment
and reported hearing disability among adults in great
britain. Int J Epidemiol., 18:911–17.
Davis, A. (1995). Prevalence of hearing impairment. In
Hearing in adults, chapter 3, pages 46–45. London:
Whurr Ltd.
Espmark, A., Rosenhall, U., Erlandsson, S., and Steen, B.
(2002). The two faces of presbiacusia. hearing impair-
ment and psychosocial consequences. Int J Audiol,
42:125–35.
Fernandez, A., Ortega, M., Penedo, M., Cancela, B., and
Gigirey, L. (2013). Automatic eye gesture recogni-
tion in audiometries for patients with cognitive de-
cline. In Image Analysis and Recognition, volume
7950 of Lecture Notes in Computer Science, pages
27–34. Springer Berlin Heidelberg.
Hall, M., Frank, E., Holmes, G., Pfahringer, B., Reutemann,
P., and Witten, I. H. (2009). The WEKA data min-
ing software: An update. SIGKDD Explor. Newsl.,
11(1):10–18.
Happy, S., George, A., and Routray, A. (2012). A real time
facial expression classification system using local bi-
nary patterns. In Intelligent Human Computer Inter-
action (IHCI), 2012 4th International Conference on,
pages 1–5.
Harris, C. and Stephens, M. (1988). A combined corner and
edge detector. In Proceedings of the 4th Alvey Vision
Conference, pages 147–151.
IMSERSO (2008). Las personas mayores en Espaa. In In-
stituto de Mayores y Servicios Sociales.
Lowe, D. G. (2004). Distinctive image features from scale-
invariant keypoints. Int. J. Comput. Vision, 60(2):91–
110.
Lucas, B. D. and Kanade, T. (1981). An iterative image
registration technique with an application to stereo vi-
sion. In Proceedings of the 7th international joint
conference on Artificial intelligence - Volume 2, IJ-
CAI’81, pages 674–679, San Francisco, CA, USA.
Morgan Kaufmann Publishers Inc.
Murlow, C., Aguilar, C., Endicott, J., Velez, R., Tuley, M.,
Charlip, W., and Hill, J. (1990). Asociation between
hearing impairment and the quality of life of elderly
individuals. volume 38, pages 45–50. J Am Geriatr
Soc.
Rosten, E. and Drummond, T. (2005). Fusing points and
lines for high performance tracking. In Computer
Vision, 2005. ICCV 2005. Tenth IEEE International
Conference on, volume 2, pages 1508–1515.
Rosten, E. and Drummond, T. (2006). Machine learning for
high-speed corner detection. In Proceedings of the 9th
ICAART2014-InternationalConferenceonAgentsandArtificialIntelligence
228