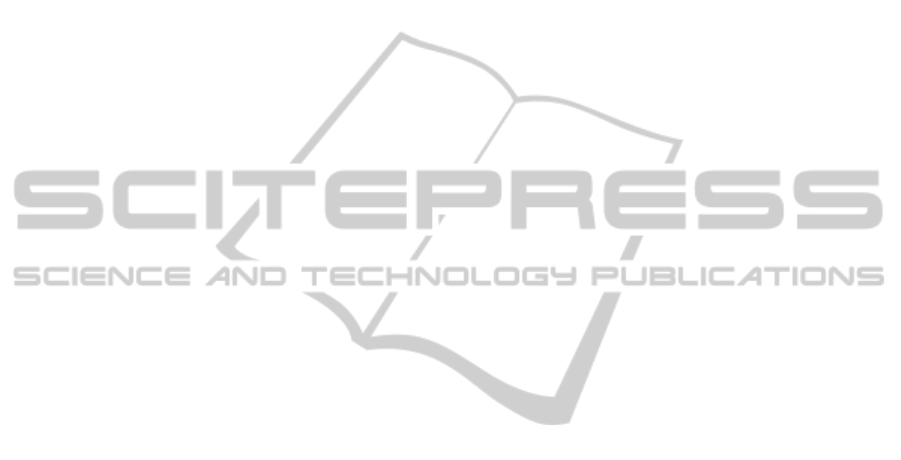
Bol´on-Canedo, V., S´anchez-Maro˜no, N., and Alonso-
Betanzos, A. (2009). A combination of discretiza-
tion and filter methods for improving classification
performance in kdd cup 99 dataset. In Neural Net-
works, 2009. IJCNN 2009. International Joint Con-
ference on, pages 359–366. IEEE.
Bol´on-Canedo, V., S´anchez-Maro˜no, N., and Alonso-
Betanzos, A. (2010a). On the effectiveness of dis-
cretization on gene selection of microarray data. In
International Joint Conference on Neural Networks.
IJCNN 2010, pages 3167–3174. IEEE.
Bol´on-Canedo, V., S´anchez-Maro˜no, N., and Alonso-
Betanzos, A. (2011b). Feature selection and classi-
fication in multiple class datasets: An application to
kdd cup 99 dataset. Expert Systems with Applications,
38(5):5947–5957.
Bol´on-Canedo, V., S´anchez-Maro˜no, N., and Alonso-
Betanzos, A. (2011c). On the behavior of feature se-
lection methods dealing with noise and relevance over
synthetic scenarios. In Neural Networks (IJCNN), The
2011 International Joint Conference on, pages 1530–
1537. IEEE.
Bol´on-Canedo, V., S´anchez-Maro˜no, N., and Alonso-
Betanzos, A. (2011d). Toward an ensemble of filters
for classification. In Intelligent Systems Design and
Applications (ISDA), 2011 11th International Confer-
ence on, pages 331–336. IEEE.
Bol´on-Canedo, V., S´anchez-Maro˜no, N., and Alonso-
Betanzos, A. (2012). An ensemble of filters and classi-
fiers for microarray data classification. Pattern Recog-
nition, 45(1):531–539.
Bol´on-Canedo, V., S´anchez-Maro˜no, N., and Alonso-
Betanzos, A. (2013a). Data classification using an en-
semble of filters. Neurocomputing (In Press).
Bol´on-Canedo, V., S´anchez-Maro˜no, N., and Alonso-
Betanzos, A. (2013b). A distributed filter approach
for microarray data classification. Applied Soft Com-
puting (Under Review).
Bol´on-Canedo, V., S´anchez-Maro˜no, N., and Alonso-
Betanzos, A. (2013c). A distributed wrapper approach
for feature selection. In European Symposium on Arti-
ficial Neural Networks, ESANN 2013, pages 173–178.
Bol´on-Canedo, V., S´anchez-Maro˜no, N., and Alonso-
Betanzos, A. (2013d). A review of feature selection
methods on synthetic data. Knowledge and informa-
tion systems, 34(3):483–519.
Bol´on-Canedo, V., S´anchez-Maro˜no, N., and Alonso-
Betanzos, A. (2014). mc-relieff: An extension of
relieff for cost-based feature selection. In 6th Inter-
national Conference on Agents and Artificial Intelli-
gence (ICAART) (Accepted).
Bol´on-Canedo, V., S´anchez-Maro˜no, N., Alonso-Betanzos,
A., Ben´ıtez, J., and Herrera, F. (2013). An insight into
microarray datasets and feature selection methods: a
framework for ongoing studies. Information Sciences
(Under review).
Bol´on-Canedo, V., S´anchez-Maro˜no, N., Alonso-Betanzos,
A., and Hernandez-Pereira, E. (2010b). Feature selec-
tion and conversion methods in KDD Cup 99 dataset:
A comparison of performance. In Proceedings of the
10th IASTED International Conference, pages 58–66.
Bol´on-Canedo, V., S´anchez-Maro˜no, N., and Cervi˜no-
Rabu˜nal, J. (2013). Scaling up feature selection: A
distributed filter approach. In Advances in Artificial
Intelligence, pages 121–130. Springer.
Chidlovskii, B. and Lecerf, L. (2008). Scalable feature se-
lection for multi-class problems. In Machine Learning
and Knowledge Discovery in Databases, pages 227–
240. Springer.
Dash, M. and Liu, H. (1997). Feature selection for classifi-
cation. Intelligent data analysis, 1(3):131–156.
Frank, A. and Asuncion, A. (2010). UCI Machine Learn-
ing Repository. http://archive.ics.uci.edu/ml. [Online;
accessed December-2013].
Guyon, I., Gunn, S., Nikravesh, M., and Zadeh, L. (2006).
Feature extraction: foundations and applications, vol-
ume 207. Springer.
Hern´andez-Pereira, E., Bol´on-Canedo, V., S´anchez-
Maro˜no, N.,
´
Alvarez-Est´evez, D., Moret-Bonillo, V.,
and Alonso-Betanzos, A. (2014). A comparison of
performance of k-complex classification methods us-
ing feature selection. Information Sciences (Under re-
view).
Hughes, G. (1968). On the mean accuracy of statistical pat-
tern recognizers. Information Theory, IEEE Transac-
tions on, 14(1):55–63.
Kohavi, R. and John, G. H. (1997). Wrappers for feature
subset selection. Artificial intelligence, 97(1):273–
324.
Liu, H. and Yu, L. (2005). Toward integrating feature
selection algorithms for classification and clustering.
Knowledge and Data Engineering, IEEE Transactions
on, 17(4):491–502.
Loscalzo, S., Yu, L., and Ding, C. (2009). Consensus
group stable feature selection. In Proceedings of
the 15th ACM SIGKDD international conference on
Knowledge discovery and data mining, pages 567–
576. ACM.
Peng, Y., Wu, Z., and Jiang, J. (2010). A novel feature
selection approach for biomedical data classification.
Journal of Biomedical Informatics, 43(1):15–23.
Peteiro-Barral, D., Bolon-Canedo, V., Alonso-Betanzos,
A., Guijarro-Berdinas, B., and S´anchez-Maro˜no, N.
(2012). Scalability analysis of filter-based methods
for feature selection. Advances in Smart Systems Re-
search, 2(1):21–26.
Peteiro-Barral, D., Bol´on-Canedo, V., Alonso-Betanzos,
A., Guijarro-Berdi˜nas, B., and S´anchez-Maro˜no, N.
(2013). Toward the scalability of neural networks
through feature selection. Expert Systems with Ap-
plications, 40(8):2807–2816.
Porto-D´ıaz, I., Bol´on-Canedo, V., Alonso-Betanzos, A.,
and Fontenla-Romero, O. (2011). A study of perfor-
mance on microarray data sets for a classifier based
on information theoretic learning. Neural Networks,
24(8):888–896.
Provost, F. (2000). Distributed data mining: Scaling up and
beyond. Advances in distributed and parallel knowl-
edge discovery, pages 3–27.
Rego-Fern´andez, D., Bol´on-Canedo, V., and Alonso-
Betanzos, A. (2014). Scalability analysis of mrmr
for microarray data. In 6th International Conference
NovelFeatureSelectionMethodsforHighDimensionalData
13