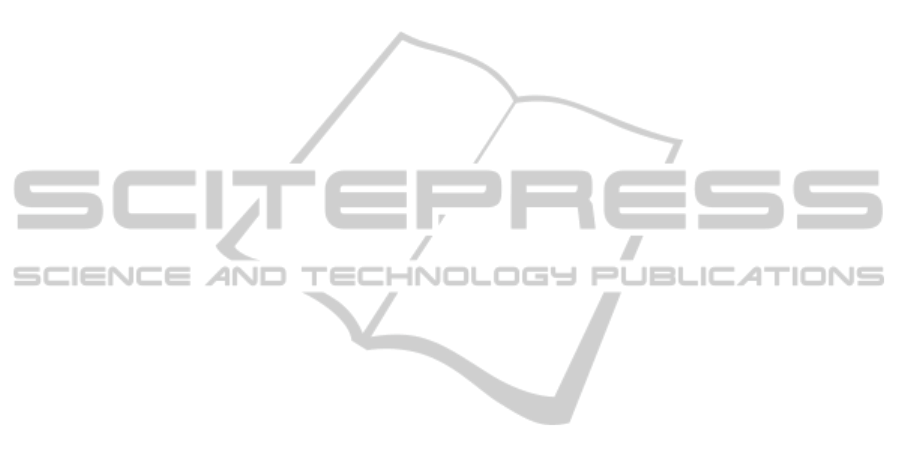
Bounded Rationality. American Economic Review Pa-
pers and Proceedings, 81(2):353–359.
Arthur, W. B. (1993). On designing economic agents that
behave like human agents. Journal of Ecolutionary
Economics, 3:1–22.
Ballinger, T. P., Palumbo, M. G., and Wilcox, N. T.
(2003). Precautionary saving and social learning
across generations: an experiment. Economic Jour-
nal, 113(490):920–947.
Binmore, K. (1999). Why Experiment in Economics? Eco-
nomic Journal, 109(453):16–24.
Birchenhall, C. R. (1995). Genetic algorithms, classifier
systems and genetic programming and their use in the
models of adaptive behaviour and learning. The Eco-
nomic Journal, 105(430):788–795.
Booker, L. B., Goldberg, D. E., and Holland, J. H. (1989).
Classifier systems and genetic algorithms. Artificial
Intelligence, 40(1-3):235–282.
Brock, W. A. and Hommes, C. H. (1997). A rational route
to randomness. Econometrica, 65(5):1059–1096.
Camerer, C. F., Loewenstein, G., and Rabin, M. (2004). Ad-
vances in Behavioral Economics. Princeton Univer-
sity Press, Princeton.
Carroll, C. (2012a). Lecture notes on solution methods for
microeconomicdynamic stochastic optimization prob-
lems. Lecture Notes - John Hopkins University.
Carroll, C. D. (1996). Buffer-stock saving and the life cy-
cle/permanent income hypothesis. NBER Working
Papers 5788, National Bureau of Economic Research,
Inc.
Carroll, C. D. (2012b). Representing consumption and sav-
ing without a representative consumer. In Measuring
Economic Sustainability and Progress, NBER Chap-
ters. National Bureau of Economic Research, Inc.
Caverzasi, E. and Godin, A. (2013). Stock-flow consistent
modeling through the ages. Economics working paper
archive, Levy Economics Institute, The.
Chen, S.-H. (2012). Varieties of agents in agent-based com-
putational economics: A historical and an interdisci-
plinary perspective. Journal of Economic Dynamics
and Control, 36(1):1–25.
Chen, S.-H. and Yu, T. (2011). Agents learned, but
do we? knowledge discovery using the agent-based
double auction markets. In Frontiers of Electrical
and Electronic Engineering in China, pages 159–170.
Springer.
Coller, M. and Williams, M. (1999). Eliciting individual
discount rates. Experimental Economics, 2(2):107–
127.
Copeland, M. A. (1949). Social accounting for moneyflows.
The Accounting Review, page 254264.
Copeland, M. A. (1962). Some illustrative analytical uses of
flow-of-funds data. In The Flow-of Funds Approach to
Social Accounting, pages 195–238. National Bureau
of Economic Research, Inc.
Cubitt, R. and Read, D. (2007). Can intertemporal choice
experiments elicit time preferences for consumption?
Experimental Economics, 10:369–89.
Davis, D. D. and Holt, C. A. (1993). Experimental eco-
nomics: Methods, problems and promise. Estudios
Economicos, 8(2):179–212.
Dawid, H. (1996). Adaptive Learning by Genetic Algo-
rithms: Analytical Results and Applications to Eco-
nomic Models. Springer Verlag New York, Inc.
Dawid, H. and Dermietzel, J. (2006). How robust is the
equal split norm? responsive strategies, selection
mechanisms and the need for economic interpretation
of simulation parameters. Computational Economics,
28(4).
Delli Gatti, D., Gaffeo, E., Gallegati, M., Giulioni, G.,
and Palestrini, A. (2008). Emergent Macroeconomics.
Springer Verlag, Berlin.
Dosi, G., Fagiolo, G., Napoletano, M., and Roventini, A.
(2013). Income distribution, credit and fiscal policies
in an agent-based keynesian model. Journal of Eco-
nomic Dynamics and Control, 37(8):1598–1625.
Dosi, G., Fagiolo, G., and Roventini, A. (2010). Schum-
peter meeting keynes: A policy-friendly model of en-
dogenous growth and business cycles. Journal of Eco-
nomic Dynamics and Control, 34(9):1748–1767.
Duffy, J. (2006). Agent-based models and human subject
experiments. In Tesfatsion, L. and Judd, K. L., editors,
Handbook of Computational Economics, volume 2 of
Handbook of Computational Economics, chapter 19,
pages 949–1011. Elsevier.
Duffy, J. (2008). experimental macroeconomics. In
Durlauf, S. N. and Blume, L. E., editors, The New Pal-
grave Dictionary of Economics, volume 3. Palgrave
Macmillan, Basingstoke, second edition.
Epstein, J. M. (2007). Agent-based computational mod-
els and generative social science. In Generative So-
cial Science Studies in Agent-Based Computational
Modeling, Introductory Chapters. Princeton Univer-
sity Press.
Fagiolo, G., Birchenhall, C., and Windrum, P. (2007a). Em-
pirical Validation in Agent-based Models: Introduc-
tion to the Special Issue. Computational Economics,
30(3):189–194.
Fagiolo, G., Moneta, A., and Windrum, P. (2007b). A
critical guide to empirical validation of agent-based
models in economics: Methodologies, procedures,
and open problems. Computational Economics,
30(3):195–226.
Giulioni, G., Bucciarelli, E., Silvestri, M., and D’Orazio,
P. (2013). Agent-based computational economics and
experimental economics: a bridge to progress macroe-
conomics. manuscript submitted.
Gode, D. and Sunder, S. (1991). Allocative efficiency of
markets with zero intelligence (z1) traders: Market
as a partial substitute for individual rationality. Gsia
working papers, Carnegie Mellon University, Tepper
School of Business.
Greiner, B. (2004). An online recruitment system
for economic experiments. Forschung und wis-
senschaftliches rechnen 2003, GWDG Bericht.
Hansen, L. P. and Heckman, J. J. (1996). The empirical
foundations of calibration. Journal of Economic Per-
spectives, 10(1):87–104.
Harrison, G. W., Harstad, R. M., and Rutstrm, E. E. (1995).
Experimental methods and elicitation of values. Dis-
cussion paper serie b, University of Bonn, Germany.
ICAART2014-DoctoralConsortium
20