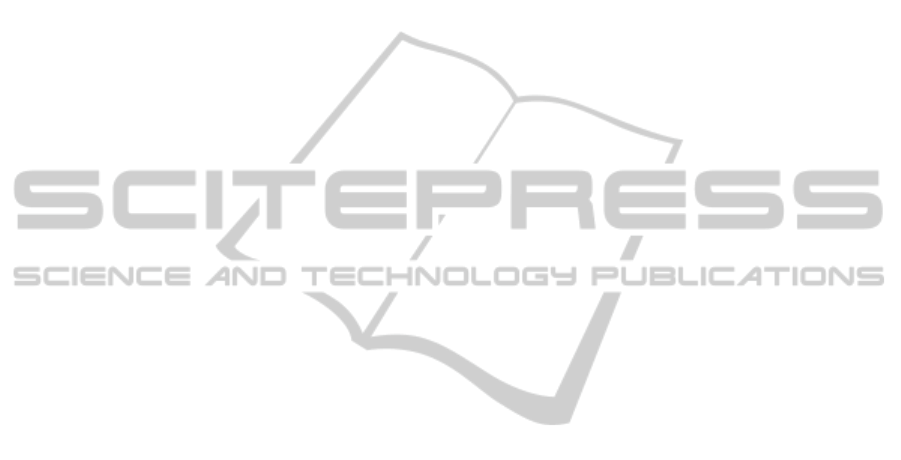
6 EXPECTED OUTCOME
The expected outcome of this project is the
development of a novel whole-building energy
model. The model will take advantage of historical
measured data of commercial buildings in order to
generate accurate prediction of heating and cooling
load. Data analysis will be one of the milestones of
this project, since usually measurements include
missing values due to equipment malfunction,
maintenance, etc. An efficient method of dealing
with missing values related with acquired datasets
will be the first outcome of the project.
Once a comprehensive dataset is obtained, the
most suitable methodology for this application is
going to be selected between regression, SVM and
ANN models. An evaluation of the developed
models will take place based on the accuracy of each
model and its ability to train on-line or not. The
selection of the most appropriate model will be the
second outcome.
After the selection process, the chosen model
will be evaluated based on its scalability. The ability
of forecasting heating and cooling loads of two
different given building within the same level of
accuracy will be the criterion. If the chosen model
does not have the desired scalability, then another
model will be selected from the previous procedure
and examined based on its scalability. Finally, the
effort required for commissioning and maintenance
of the model should be as little as possible. The final
outcome should be a scalable model with minimum
commissioning and maintenance requirements.
Ideally, this novel approach of estimating the
thermal and cooling load of commercial buildings
could be implemented to the control of the BEMS.
In this way, the efficiency of the HVAC systems of
the building could be improved reducing the energy
consumption at the same time. This will also lead to
a reduction of the energy cost of commercial
buildings.
REFERENCES
Aranda, A. et al., 2012. Multiple regression models to
predict the annual energy consumption in the Spanish
banking sector. Energy and Buildings, Volume 49, pp.
380-387.
ASHRAE, 2009. Energy Estimating and Modeling
Methods. In: ASHRAE Handbook-Fundamentals.
Atlanta: American Society of Heating, Refrigerating
and Air-Conditioning Engineers, Inc., pp. 32.1-32.33.
Aydinalp-Koksal, M. & Ugursal, V. I., 2008. Comparison
of neural network, conditional demand analysis, and
engineering approaches for modeling end-use energy
consumption in the residential sector. Applied Energy,
85(4), pp. 271-296.
Bauer, M. & Scrartezzini, J., 1998. A simplified
correlation method accounting for heating and cooling
loads in energy-efficient buildings. Energy and
Buildings, 27(2), pp. 147-154.
Catalina, T., Iordache, V. & Caracaleanu, B., 2013.
Multiple regression model for fast prediction of the
heating energy demand. Energy and Buildings,
Volume 57, pp. 302-312.
Catalina, T., Virgone, J. & Blanco, E., 2008. Development
and validation of regression models to predict monthly
heating demand for residential buildings. Energy and
Buildings, Volume 40, pp. 1825-1832.
Dombayci, A., 2010. The prediction of heating energy
consumption in a model house by using artificial
neural networks in Denizli–Turkey. Advances in
Engineering Software, 41(2), pp. 356-362.
Dong, B., Cao, C. & Lee, S. E., 2005. Applying support
vector machines to predict building energy
consumption in tropical region. Energy and Buildings,
37(5), pp. 545-553.
Ekici, B. B. & Aksoy, U. T., 2009. Prediction of building
energy consumption by using artificial neural
networks. Advances in Engineering Software, 40(5),
pp. 356-362.
Fausett, L., 1994. Fundamentals of neural networks:
architectures, algorithms, and applications. Upper
Saddle River, NJ, USA: Prentice-Hall, Inc.
Foucquier, A. et al., 2013. State of the art in building
modelling and energy performances prediction: A
review. Renewable and Sustainable Energy Reviews,
Volume 23, pp. 272-288.
González, P. A. & Zamarreño, J. M., 2005. Prediction of
hourly energy consumption in buildings based on a
feedback artificial neural network. Energy and
Buildings, 37(6), pp. 595-601.
Hou, Z. & Lian, Z., 2009. An Application of Support
Vector Machines in Cooling Load Prediction. Wuhan,
s.n., pp. 1-4.
Kalogirou, S. A., Neocleous, C. C. & Schizas, C. N., 1997.
Building heating load estimation using artificial neural
networks. s.l., s.n., pp. 1-8.
Kalogirou, S., Florides, G., Neocleous, C. & Schizas, C.,
2001. Estimation of Daily Heating and Cooling Loads
Using Artificial Neural Networks. Napoli, Clima
2000.
Lam, J. C., Wan, K. K. W., Liu, D. & Tsang, C. L., 2010.
Multiple regression models for energy use in air-
conditioned office buildings in different climates.
Energy Conversion and Management, 51(12), pp.
2692-2697.
Li, Q. et al., 2009. Applying support vector machine to
predict hourly cooling load in the building. Applied
Energy, 86(10), pp. 2249-2256.
Li, Q. et al., 2009. Predicting hourly cooling load in the
building: A comparison of support vector machine and
ResearchonTechniquesforBuildingEnergyModel
29