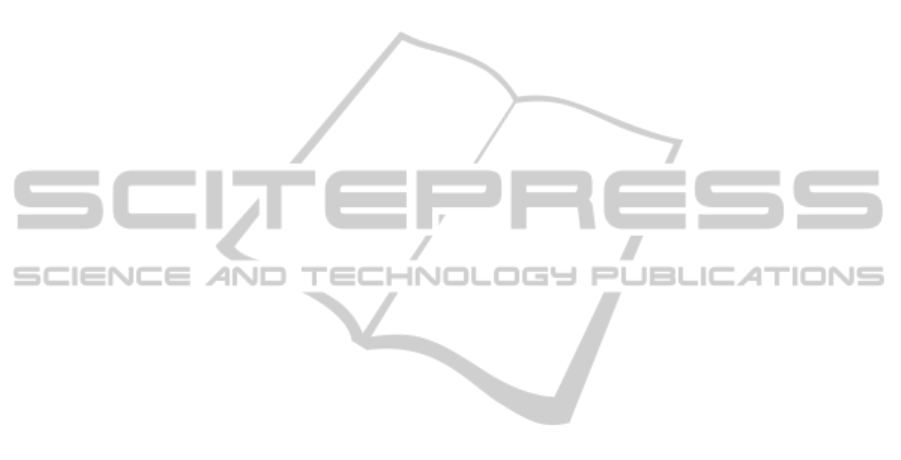
edge about genetic variants that influence the suscep-
tibility to complex diseases. In the process, it may
help gain progress across multiple-domains, includ-
ing:
• machine learning and data mining: statistical
models and techniques for dealing with GWAS
data that take account high-dimensionality and
variable correlation will be designed; methods for
the improvement of models through integrating
additional knowledge will be proposed;
• medicine: the methods designed intend to bring
new evidence of genetic disease susceptibility
as well as individual genetic susceptibility to
drugs, thus offering perspectives for personalized
medicine;
• public health: our work will contribute to account-
ing for the societal evolution trend toward early
gene susceptibility detection to improve preven-
tion or surveillance;
• economy: The animal and plant biology domains
are also concerned with respect to the selection of
phenotypes of interest in agronomy as well;
• high-performance computing for GWASs: cutting
edge techniques for implementing GWAS strate-
gies and large-scale machine learning approaches
will be implemented.
REFERENCES
Abel, H. J. and Thomas, A. (2011). Accuracy and computa-
tional efficiency of a graphical modeling approach to
linkage disequilibrium estimation. Statistical applica-
tions in genetics and molecular biology, 10(1).
Auber, D. (2004). Tulip: A huge graph visualization frame-
work. In Graph Drawing Software, pages 105–126.
Springer.
Balding, D. J. (2006). A tutorial on statistical methods for
population association studies. Nature Reviews Ge-
netics, 7(10):781–791.
Botta, V., Hansoul, S., Geurts, P., and Wehenkel, L. (2008).
Raw genotypes vs haplotype blocks for genome wide
association studies by random forests. In Proc. of
MLSB 2008, second workshop on Machine Learning
in Systems Biology.
Browning, B. L. and Browning, S. R. (2009). A unified ap-
proach to genotype imputation and haplotype-phase
inference for large data sets of trios and unrelated in-
dividuals. The American Journal of Human Genetics,
84(2):210–223.
Dagum, L. and Menon, R. (1998). Openmp: an industry
standard api for shared-memory programming. Com-
putational Science & Engineering, IEEE, 5(1):46–55.
Fabregat-Traver, D. and Bientinesi, P. (2012). Comput-
ing petaflops over terabytes of data: The case of
genome-wide association studies. arXiv preprint
arXiv:1210.7683.
Friedman, N., Linial, M., Nachman, I., and Pe’er, D. (2000).
Using bayesian networks to analyze expression data.
Journal of computational biology, 7(3-4):601–620.
Gabriel, E., Fagg, G. E., Bosilca, G., Angskun, T., Don-
garra, J. J., Squyres, J. M., Sahay, V., Kambadur, P.,
Barrett, B., Lumsdaine, A., et al. (2004). Open mpi:
Goals, concept, and design of a next generation mpi
implementation. In Recent Advances in Parallel Vir-
tual Machine and Message Passing Interface, pages
97–104. Springer.
Han, B., Kang, H., Seo, M. S., Zaitlen, N., and Eskin, E.
(2008). Efficient association study design via power-
optimized tag snp selection. Annals of human genet-
ics, 72(6):834–847.
Hechter, E. (2011). On genetic variants underlying common
disease. PhD thesis, Oxford University.
Klein, R. J., Zeiss, C., Chew, E. Y., Tsai, J.-Y., Sackler,
R. S., Haynes, C., Henning, A. K., SanGiovanni, J. P.,
Mane, S. M., Mayne, S. T., et al. (2005). Complement
factor h polymorphism in age-related macular degen-
eration. Science, 308(5720):385–389.
Kollar, D. and Friedman, N. (2009). Probabilistic graphical
models: principles and techniques. The MIT Press.
Laramie, J. M., Wilk, J. B., DeStefano, A. L., and Myers,
R. H. (2007). Haplobuild: an algorithm to construct
non-contiguous associated haplotypes in family based
genetic studies. Bioinformatics, 23(16):2190–2192.
Lee, P. H. and Shatkay, H. (2006). Bntagger: improved tag-
ging snp selection using bayesian networks. In ISMB
(Supplement of Bioinformatics), pages 211–219.
Moore, J. H., Asselbergs, F. W., and Williams, S. M. (2010).
Bioinformatics challenges for genome-wide associa-
tion studies. Bioinformatics, 26(4):445–455.
Mourad, R., Sinoquet, C., and Leray, P. (2010). Learning
hierarchical bayesian networks for genome-wide asso-
ciation studies. In Proceedings of COMPSTAT’2010,
pages 549–556. Springer.
Mourad, R., Sinoquet, C., and Leray, P. (2011). A hier-
archical bayesian network approach for linkage dis-
equilibrium modeling and data-dimensionality reduc-
tion prior to genome-wide association studies. BMC
bioinformatics, 12(1):16.
Mourad, R., Sinoquet, C., Zhang, N. L., Liu, T., Leray, P.,
et al. (2013). A survey on latent tree models and ap-
plications. J. Artif. Intell. Res.(JAIR), 47:157–203.
Nefian, A. V. (2006). Learning snp dependencies using em-
bedded bayesian networks. In IEEE Computational
Systems, Bioinformatics Conference, pages 1–6.
Patil, N., Berno, A. J., Hinds, D. A., Barrett, W. A.,
Doshi, J. M., Hacker, C. R., Kautzer, C. R., Lee,
D. H., Marjoribanks, C., McDonough, D. P., et al.
(2001). Blocks of limited haplotype diversity revealed
by high-resolution scanning of human chromosome
21. Science, 294(5547):1719–1723.
Pattaro, C., Ruczinski, I., Fallin, D., and Parmigiani, G.
(2008). Haplotype block partitioning as a tool for
dimensionality reduction in snp association studies.
BMC genomics, 9(1):405.
BIOSTEC2014-DoctoralConsortium
80