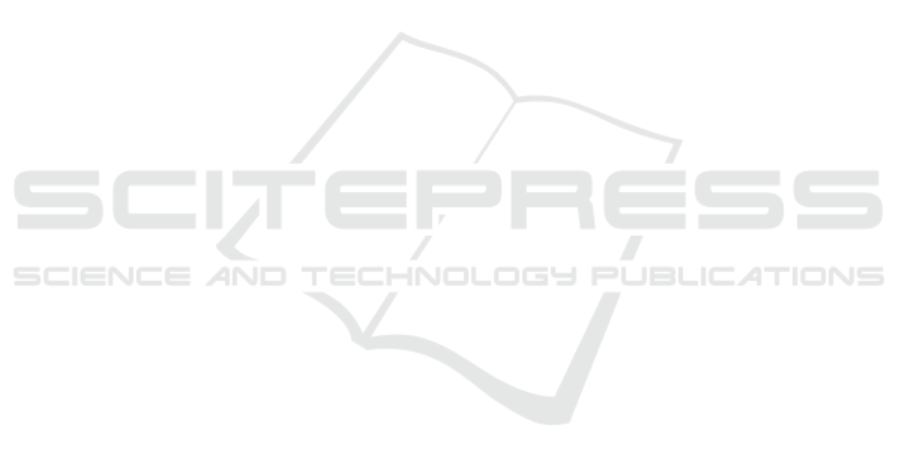
Paris-rue-Madame Database
A 3D Mobile Laser Scanner Dataset for Benchmarking Urban Detection,
Segmentation and Classification Methods
Andr´es Serna
1
, Beatriz Marcotegui
1
, Franc¸ois Goulette
2
and Jean-Emmanuel Deschaud
2
1
MINES ParisTech, CMM-Center for Mathematical Morphology, 35 rue St Honor´e, 77305 Fontainebleau, France
2
MINES ParisTech, CAOR-Center for Robotics, 60 boulevard Saint-Michel, 75272 Paris, France
Keywords:
3D Database, Mobile Laser Scanner, Urban Analysis, Segmentation, Classification, Point-wise Evaluation.
Abstract:
This paper describes a publicly available 3D database from the rue Madame, a street in the 6
th
Parisian district.
Data have been acquired by the Mobile Laser Scanning (MLS) system L3D2 and correspond to a 160 m long
street section. Annotation has been carried out in a manually assisted way. An initial annotation is obtained
using an automatic segmentation algorithm. Then, a manual refinement is done and a label is assigned to
each segmented object. Finally, a class is also manually assigned to each object. Available classes include
facades, ground, cars, motorcycles, pedestrians, traffic signs, among others. The result is a list of (X, Y, Z,
reflectance, label, class) points. Our aim is to offer, to the scientific community, a 3D manually labeled dataset
for detection, segmentation and classification benchmarking. With respect to other databases available in the
state of the art, this dataset has been exhaustively annotated in order to include all available objects and to
allow point-wise comparison.
1 INTRODUCTION
Nowadays, LiDAR technology (“light detection and
ranging”) has been prospering in the remote sensing
community thanks to developments such as: Aerial
Laser Scanning (ALS), useful for large scale build-
ings survey, roads and forests; Terrestrial Laser Scan-
ning (TLS), for more detailed but slower urban sur-
veys in outdoor and indoor environments; Mobile
Laser Scanning (MLS), less precise than TLS but
much more productive since the sensors are mounted
on a vehicle; and more recently, “stop and go” sys-
tems, easy transportable TLS systems making a trade
off between precision and productivity.
Thanks to all these technologies, the amount of
available 3D geographical data and processing tech-
niques has bloomed in recent years. Many semi-
automatic and automatic methods aiming at analyzing
3D urban point clouds can be found in the literature. It
is an active research area. However, there is not a gen-
eral consensus about the best detection, segmentation
and classification methods. This choice is application
dependent. One of the main drawbacks is the lack of
publicly available databases allowing benchmarking.
In the literature, most available urban data consist
in close-range images, aerial images, satellite images
but a few laser datasets (ISPRS, 2013; IGN, 2013).
Moreover, manual annotations and algorithm outputs
are rarely found in available 3D repositories (N¨uchter
and Lingemann, 2011; CoE LaSR, 2013).
Some available data include Oakland dataset
(Munoz et al., 2009), which contains 1.6 million
points collected around Carnegie Mellon University
campus in Oakland, Pittsburgh, USA. Data are pro-
vided in ASCII format: (X, Y, Z, label, confidence)
one point per line, vrml files and label counts. The
training/validation and testing data contains 5 labels
(scatter misc, default wires, utility poles, load bear-
ing and facades). Ohio database (Golovinskiy et al.,
2009) is a combination of ALS and TLS data in Ot-
tawa city (Ohio, USA). It contains 26 tiles 100 ×
100 meters each with several objects such as build-
ings, trees, cars and lampposts. However, ground
truth annotations only consists in a 2D labeled point
in the center of each object. In that sense, segmenta-
tions results cannot be evaluated point by point. En-
schede database (Zhou and Vosselman, 2012) con-
tains residential streets approximatively 1 km long in
the Enschede city (The Netherlands). Ground truth
annotation consists in 2D geo-referenced lines mark-
ing curbstones. A well- defined evaluation method
is available using buffers around each 2D line. The
819
Serna A., Marcotegui B., Goulette F. and Deschaud J..
Paris-rue-Madame Database - A 3D Mobile Laser Scanner Dataset for Benchmarking Urban Detection, Segmentation and Classification Methods.
DOI: 10.5220/0004934808190824
In Proceedings of the 3rd International Conference on Pattern Recognition Applications and Methods (USA-2014), pages 819-824
ISBN: 978-989-758-018-5
Copyright
c
2014 SCITEPRESS (Science and Technology Publications, Lda.)