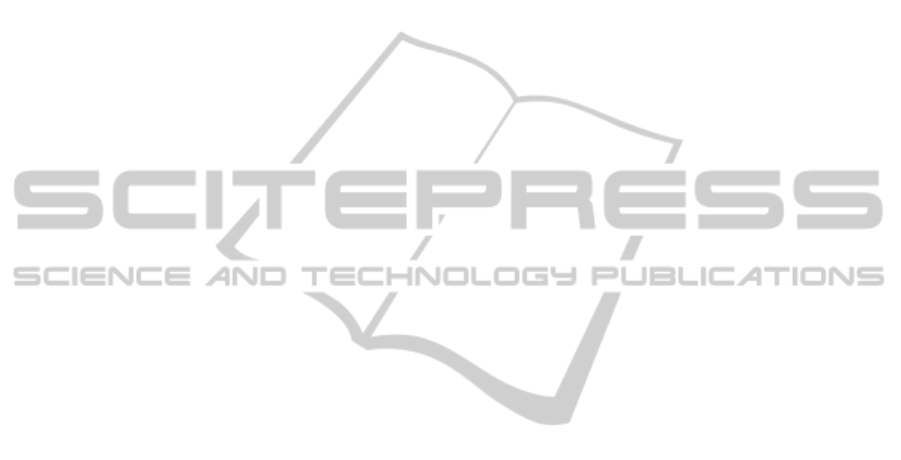
risks for cardio-metabolic diseases and implement
personalized prevention actions.
In the following, we will review and analyse
some basic aspects of face semeiotics so as to define
the traits of a related computational model.
2 MEDICAL SEMEIOTICS
The face is a fine descriptor of a person’s well-being
state and, people, not only doctors, commonly derive
from the observation of the face significant clues
about psychophysical condition. Evidence on the
state of nutrition, fitness, and mental state can be
obtained. In addition, conditions affecting the colour
or the appearance of the skin can be also revealed.
The appearance and features of the face allow the
distinction among ethnicity, gender, age and
emotions (such as happiness, sadness, fear, anxiety,
and pain). Face changes can be due to alterations of
skeletal and/or muscular structure, subcutaneous
tissue, colour of the skin and eyeballs appearance.
For examples, chronic endocrinological diseases
(achondroplasia, acromegaly) and congenital anemia
(thalassemia) may produce characteristic alterations
of bone structures. Diseases of the nervous system
(Parkinson, myasthenia, tetanus) may cause typical
variations of the muscular structures. Other local and
systemic illness may induce modifications of the
superficial tissues due to changes of water content,
growth of adipose tissue, and deposition of
mucoproteins such as in the case of myxoedema
(hypothyroidism). Haemoglobin concentration,
oxygen saturation, vasodilation or vasoconstriction
affects the colour of facial skin (pallor, redness, and
cyanosis); moreover the deposit of other substances
may be responsible of pathologic appearance of the
skin, as bilirubin in jaundice. Local accumulation of
cholesterol may become evident with the appearance
of xanthelasmas in the eyelid and arcus cornealis, a
white ring in front of the periphery of the iris.
Moreover some clusters of characteristic features of
the face are considered pathognomonic of specific
medical conditions such as mitral face (mitral
stenosis), Hippocratic face (sepsis), lunaris face
(Cushing's syndrome, obesity) and other well-known
semeiotic facies.
From this brief summary, it is evident that
building a comprehensive model of face semeiotics
able to capture all the available pieces of
information is an extremely complex task.
Therefore, focusing on a specific application helps
to make the problem tractable. Moreover, working
with a “real world” setting is expected offer a
significantly general framework for further
utilization.
That led us to focus on cardiovascular diseases
(CVD) and cardio-metabolic risk for which the need
of personalized prevention strategies has gained a
universal acceptance.
3 CARDIOMETABOLIC RISK
Atherosclerotic cardiovascular diseases (CVDs),
including heart disease and stroke, are the leading
causes of mortality worldwide (World Health
Organization, 2008). The atherosclerotic illness
develops insidiously, and clinical manifestations
often become evident in its advanced stages.
Altogether, frequently, the major events, such as
serious health complications, disability and death
occur between 40 and 60 years of age. Moreover, the
majority of patients who survive a myocardial
infarction do not fully recover the ventricular
function, and many stroke survivors have physical
limitation in the daily activities. This explains why
CVDs represent one of the major challenges to the
health systems and considerable efforts are profuse
to treat clinical manifestations of CVDs. These
efforts have granted significant advances with actual
improvements in patients’ outcome, quod ad vitam
and valitudinem (Ford et al., 2007).
Despite the success of the pharmacological,
interventional, and surgical treatment of the CVDs,
it is obvious that all these therapies cannot modify
the epidemiological impact of the disease.
Moreover, the cost of health systems grows
exponentially with the widespread use of complex,
and often inappropriate, diagnostic procedures, as
well as with population aging. At present, the
strategy of prevention, which attempts to modify
some pathophysiological factors related to the
genesis of the disease, seems to be the only way to
limit the epidemic growth of CVDs (Graham et al.,
2007). In a recent paper (Pandya et al., 2013) on
forecasting cardiovascular disease in the USA
through the year 2030, an inversion of the
epidemiologic trend was found, which predicts an
increase in the overall incidence of cardiovascular
disease. This trend is related to two independent
factors: the aging of the population and the
incidence of obesity and diabetes.
Cardio-metabolic risk is a cluster of risk factors
indicative of a patient's overall risk for CVD and
type-2 diabetes. These risk factors include: incorrect
dietary habits, physical inactivity, smoke, alcohol
abuse, abnormal lipid metabolism, hyper-glycaemia,
and arterial hypertension (Grundy et al., 2005,
National Cholesterol Education Program, 2002,
MovingMedicalSemeioticstotheDigitalRealm-SEMEOTICONSApproachtoFaceSignsofCardiometabolicRisk
607