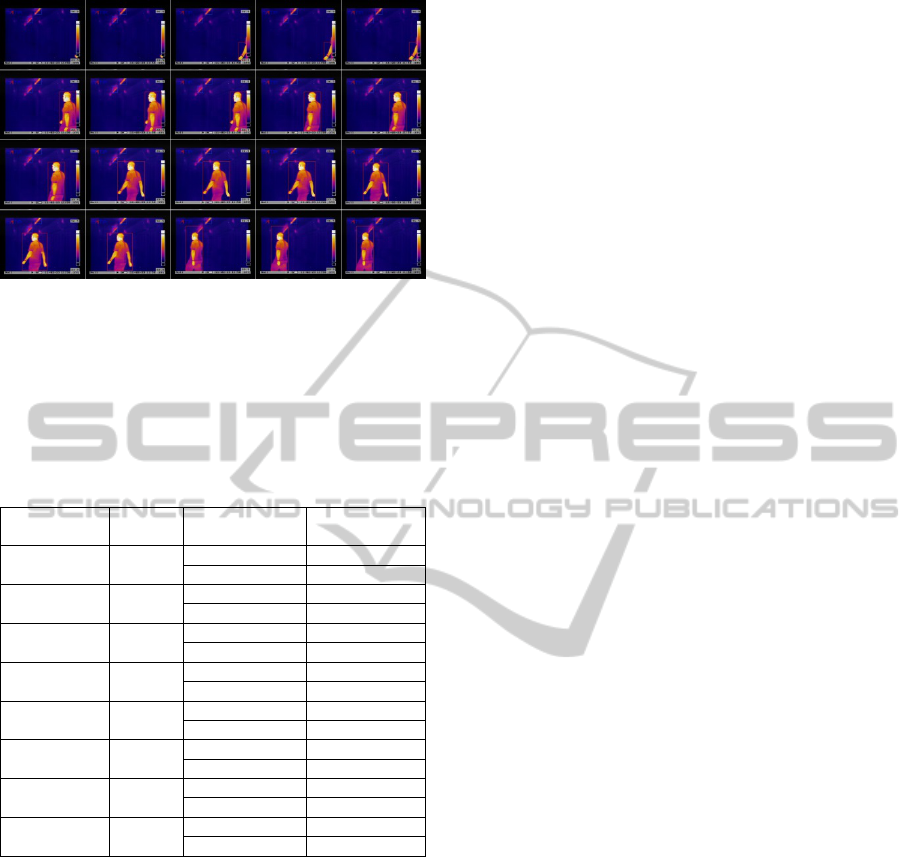
Figure 8: Detection of “iev”, frames 158-177.
It is noted that these criteria, the algorithm does not
seem particularly effective, but if, for example, is
considered an acceptable detection to detect a person
at different points, only for IP and IP2 videos would
be 100% of detections.
Table 6: Accuracy on the person detection.
Type of
video
Total
Acceptable /
Improvable
Accuracy (%)
IP 33
20 60,61
13 39,39
IP2 113
42 37,17
71 62,83
IEV 86
38 44,18
48 55,81
IEF 121
35 28,93
86 71,07
EP 45
8 17,78
37 82,22
EEV 12
12 100
0 0
EEF 73
2 2,74
71 97,26
EEF2 85
8 9,41
77 90,59
5 DISCUSSION
AND CONCLUSSIONS
This work has developed an algorithm detector heat
sources. This algorithm needs the support of a
calibration tool , which is a graphical user interface
that generates some of the sensing parameters. To do
this the user must use a fixed thermal image (photo),
representative of the scenario in question. The
sensing device consists of an infrared camera with
its video output connected to the input of an
acquisition card inserted into a computer. Recording
in AVI format generated is input to the algorithm,
which generates a video output with the same
number of frames, and frames per second that the
detected foci are framed by a rectangle. These boxes
can be different colors and indicate the nature of the
sources found. By default, the cuasidinámicos
framed by orange lights, that is, those who are
continuously detected in the scene and whose
position and dimensions vary slightly over time.
They are framed in blue static red lights and
dynamic . When a scene foci appear and disappear
continuously, are considered static those whose
position and dimensions vary slightly detection in
dynamic detection and those whose evolution is
more noticeable.
ACKNOWLEDGEMENTS
This work is supported by funds from The Spanish
Government, under Grant MCINN TEC2012-38630-
C04-02.
REFERENCES
Liew, A.L., Lim, A. Kwoh, L., 2005. A Stochastic Model
for Active Fire Detection Using the Thermal Bands of
MODIS Data. In IEEE Geoscience and Remote
Sensing Letters, Vol. 2, Issue 3, pp. 337 – 341.
Vodacek, A., Kremens, R.L., Ononye, A., Tang, C., 2005.
A Hybrid Contextual Approach to Wildland Fire
Detection Using Multispectral Imagery, In IEEE
Geoscience and Remote Sensing Letters, 43(9), pp.
2115 - 2126.
Ishino, R., 2002. Detection of a Faulty Power Distribution
Apparatus by Using Thermal Images. In IEEE Power
Engineering Society Winter Meeting, Vol. 2, pp. 1332
– 1337.
Carter, L.J., O’Sullivan, M.J., Hung, Y.J., Teng, J.C-C.,
1998. Thermal Imaging for Landmine Detection. In
Second International Conference on the Detection of
Abandoned Land Mines, pp. 110 – 114.
Azak, M.D., Akgün, S., Azak, S.I., Torun E., 2003.
Thermal Detection of Buried Circular Objects with a
Rule-Based Fast Shape Detection Algorithm. In
Proceedings of IEEE Sensors, Vol.2, pp. 765 - 768.
Heo, J., Savvides, M., Vijayakumar, B.V.K., 2005.
Performance Evaluation of Face Recognition using
Visual and Thermal Imagery with Advanced
Correlation Filters. In IEEE Computer Society
Conference on Computer Vision and Pattern
Recognitions, pp. 9.
Socolinsky, D.A., Selinger, A., 2004. Thermal Face
Recognition in an Operational Scenario. In
Proceedings of the IEEE Computer Society
Conference on Computer Vision and Pattern
Recognition, pp. 1012 - 1019.
Jiang, L., Yeo, A., Nursalim, J., Wu, S., Jiang, X., Lu,
DetectionandTrackingoftheHumanHotSpot
329