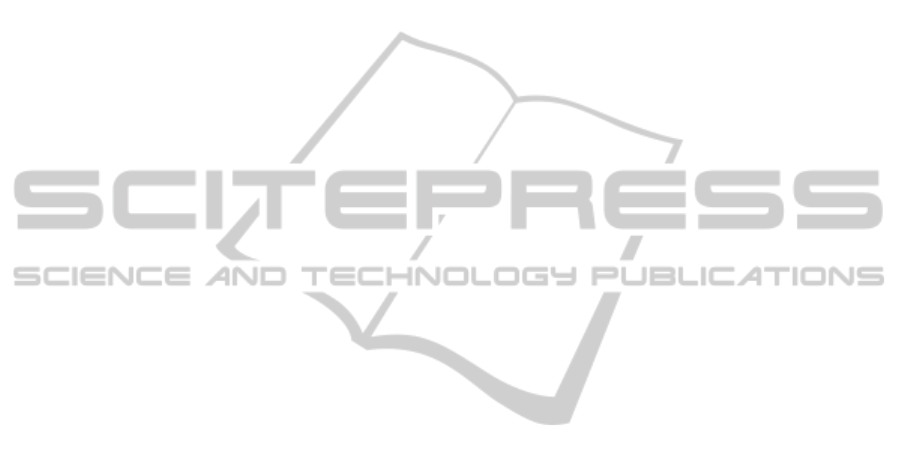
While presented in the context of matching
knowledge users with knowledge creators, this
solution can also be applied to similar problems like
matching projects or tenders with contractors. In
addition, more complex set of features that include
citation, and co-citation data can be incorporated in
future versions to enhance user experience and
provide improved matching performance. Another
way to enhance user experience is by incorporating
more data sources. It would be interesting to find
matches between patents, research publications and
business organizations. It also would be interesting
to find whether this prototype or similar ones can be
reengineered to retrieve data not just by keyword
search, but also by a particular research paper or job
post, shifting the focus from a bunch of keywords to
only one or a set of research papers or job posts. It
would be interesting to see how the solution would
perform under these conditions, and how matches
are found and computed.
ACKNOWLEDGEMENTS
We are indebted to the Ministry of Higher Education
in Saudi Arabia for their continued support.
REFERENCES
Antezana, E., Kuiper, M. and Mironov, V., 2009.
Biological knowledge management: The emerging
role of the Semantic Web technologies. Briefings in
Bioinformatics, 10, pp.392–407.
Bielefeld University, 2014. About Bielefeld Academic
Search Engine (BASE).
Bilenko, M. and Mooney, R.J., 2003. On evaluation and
training-set construction for duplicate detection. In
Proceedings of the KDD-2003 Workshop on Data
Cleaning, Record Linkage, and Object Consolidation.
pp. 7–12.
Bozeman, B., 2000. Technology transfer and public
policy: a review of research and theory. Research
Policy, 29, pp.627–655.
Campbel, S. and Swigart, S., 2014. Go Beyond Google:
Gathering Internet Intelligence 5th editio., Cascade
Insight.
Carmel, D. et al., 2001. Static index pruning for
information retrieval systems. In Proceedings of the
24th annual international ACM SIGIR conference on
Research and development in information retrieval -
SIGIR ’01. pp. 43–50.
Chitika Insights, 2013. Online Ad CTR: Impact of
Referring Google Result Position
Chung, W., 2004. An automatic text mining framework
for knowledge discovery on the Web. University of
Arizona.
Cohen, W.W., 1998. Integration of heterogeneous
databases without common domains using queries
based on textual similarity. Proceedings of the 1998
ACM SIGMOD international conference on
Management of data, 27, pp.201–212.
Colas, F. and Brazdil, P., 2006. Comparison of SVM and
some older classification algorithms in text
classification tasks. IFIP International Federation for
Information Processing, 217, pp.169–178.
Council of Canadian Academies, 2012. The State of
Science and Technology in Canada, Ottawa, Ontario.
Deerwester, S. et al., 1990. Indexing by latent semantic
analysis. Journal of the American Society for
Information Science, 41, pp.391–407.
Dooris, M.J., 1989. Organizational Adaptation and the
Commercialization of Research Universities. Planning
for Higher Education, 17(3), pp.21–31.
Dorneles, C.F., Gonçalves, R. and Santos Mello, R., 2010.
Approximate data instance matching: a survey.
Knowledge and Information Systems, 27(1), pp.1–21.
Elmagarmid, A.K., Ipeirotis, P.G. and Verykios, V.S.,
2007. Duplicate record detection: A survey. IEEE
Transactions on Knowledge and Data Engineering, 19,
pp.1–16.
Ertek, G., Tapucu, D. and Arin, I., 2013. Text mining with
rapidminer. In M. Hofmann and R. Klinkenberg, eds.
RapidMiner: Data Mining Use Cases and Business
Analytics Applications. Boca Raton, FL: CRC Press,
pp. 241–261.
Etzkowitz, H., 2002. Incubation of incubators: innovation
as a triple helix of university–industry–government
networks Henry. Science and Public Policy, 29,
pp.115–128.
Etzkowitz, H. and Peters, L.S., 1991. Profiting from
knowledge: Organisational innovations and the
evolution of academic norms. Minerva, 29(2), pp.133–
166.
Fellegi, I.P. and Sunter, A.B., 1969. A Theory for Record
Linkage. Journal of the American Statistical
Association, 64, pp.1183–1210.
Kannan, A. et al., 2011. Matching unstructured product
offers to structured product specifications. In
Proceedings of the 17th ACM SIGKDD international
conference on Knowledge discovery and data mining -
KDD ’11. New York, New York, USA: ACM Press,
pp. 404–412.
Karlsson, M., 2004. Commercialization of Research
Results in the United States: An Overview of Federal
and Academic Technology Transfer, Washington, DC.
Köpcke, H., Thor, A. and Rahm, E., 2010. Evaluation of
entity resolution approaches on real-world match
problems. Proceedings of the VLDB Endowment, 3,
pp.484–493.
Li, F. and Yang, Y., 2003. A Loss Function Analysis for
Classification Categorization Methods in Text. In
Proceedings of the Twentieth International
Conferenceon Machine Learning. pp. 472–479.
MatchingKnowledgeUserswithKnowledgeCreatorsusingTextMiningTechniques
13