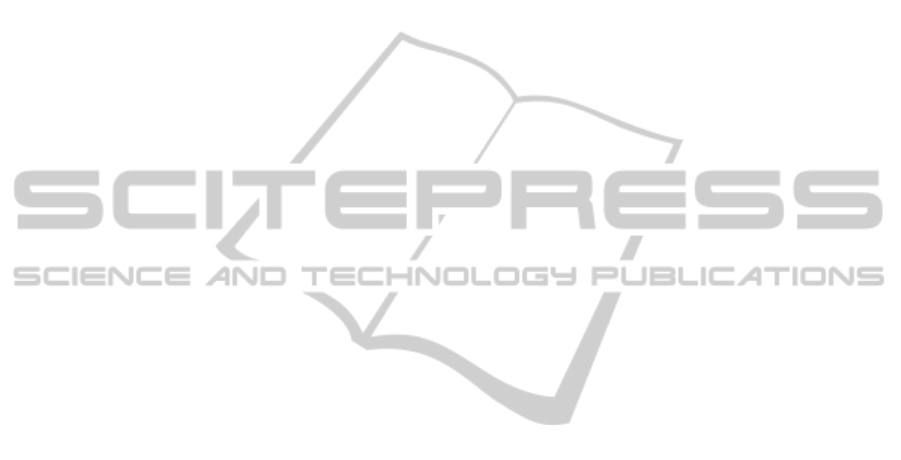
individually, as well as the whole system, against
real urinalysis cases, in order to verify if they are
able to reach correct conclusions in comparison with
expert performance and (ii) providing the system to
urinalysis professionals and students and evaluate its
effectiveness as training and decision support tool
(i.e. whether or not it improves their capabilities).
5 CONCLUDING REMARKS
Urinalysis is a relatively inexpensive but powerful
and very important laboratory test, commonly
employed in patient examination. In spite of that, for
numerous reasons, it has not received the needed
attention to achieve its whole power, which has its
roots mainly on the lack of professional qualification
and insufficient knowledge about the test.
Given that most of the problem relies on
cognitive tasks, suitable to computational modelling,
it was formulated the hypothesis that it is possible to
develop a knowledge-based system representing
urinalysis domain and that this system can be useful
to enhance this laboratory exam. We also believe
that this usefulness can be materialized in many
ways – contributing to professional qualification,
decision support and even to urinalysis automation.
With the aim of testing such hypothesis, this
paper proposes the development of such a system.
To achieve this objective, we decided to use
ontologies to model the domain, BNs to treat its
uncertain aspect and task templates to formalize the
reasoning tasks needed to well perform the analysis.
After literature immersion and interviews with an
expert in the domain, the system was designed and
the ontology construction has started.
Even though being an apparently simple
analysis, dealing with a single and so trivial body
fluid, urinalysis revealed itself as a rather complex
area. This challenging nature can be exemplified by
the great amount of concepts involved (over 250,
selected from an initial list of about a thousand of
them), by the intense flow of information during the
analysis, and the resultant intricate heuristics needed
to treat it – which demanded a handful of task
templates to represent. It is indeed a domain that
would certainly take several years to be mastered by
a novice professional.
Nevertheless, precisely due to that challenging
nature of the domain, the importance of this work
grows stronger. Besides an intelligent system to
support urinalysis, accepting different interface
layers according to the intended use, it is also being
developed an ontology model that has valuable in
itself. This model may serve as base for a series of
useful applications for the domain, not even
imagined yet. Still, considering that the
methodological knowledge to be developed during
this work may extrapolate its domain, our work may
serve as guidance to similar initiatives in correlate
domains, such as other laboratory exams.
REFERENCES
Akerkar, R., Sajja, P. 2009. Knowledge-Based Systems,
Jones and Bartlett Publishers. Sudbury, US, 1
st
edition.
Clinical and Laboratory Standards Institute (CLSI), 2001.
Approved Guideline GP16-A2: Urinalysis and
Collection, Transportation, and Preservation of Urine
Specimens, CLSI. Wayne, Pa., 2
nd
edition.
Grau, B. C., Horrocks, I., Motik, B., Parsia, B., Patel-
Schneider, P., Sattler, U., 2008. OWL2: The next step
for OWL. In: Web Semantics: Science, Services and
Agents on the World Wide Web 6.
Gruber, T. R., 1993. A Translation Approach to Portable
Ontology Specifications. In: Knowledge Acquisition.
Guizzardi, G.; Wagner, G., 2005. Some applications of a
unified foundational ontology in business modelling.
In: Business systems analysis with ontologies, Idea
Group.
Koller, C., D., Pfeffer, A., 1997. Object-Oriented Bayesian
Networks. In: 13th Annual Conference on Uncertainty
in AI (UAI).
Matsumoto, S., Carvalho, R.N., Ladeira, M., Costa,
P.C.G, Santos, L.L., Silva, D., Onishi, M., Machado,
E., 2011. UnBBayes: a Java Framework for
Probabilistic Models in AI. In: Java in Academia and
Research. iConcept Press Ltd.
Pearl, J., 1985. Bayesian Networks: A Model of Self-
Activated Memory for Evidential Reasoning. 7
th
Annual Conference of the Cognitive Science Society.
Schreiber, A. T., Akkermans, H., Anjewierden, A., de
Hoog, R., Shadbolt, N., Van de Velde, W., Wielinga,
B., 1999. Knowledge engineering and management:
the CommonKADS methodology, MIT Press.
Cambridge, MA.
Schwartz, A., Elstein, A. S., 2008. Clinical reasoning in
medicine. In: Clinical Reasoning in the Health
Professions. Elsevier, 3
rd
edition.
Rubin, D. L., Knublauch, H., Fergerson, R. W., Dameron,
O., Musen, M. A., 2005. Protégé-OWL: Creating
Ontology-Driven Reasoning Applications with the
Web Ontology Language. In: AMIA Annual
Symposium Proceedings 2005. American Medical
Informatics Association.
Knowledge-basedSystemforUrinalysis
519