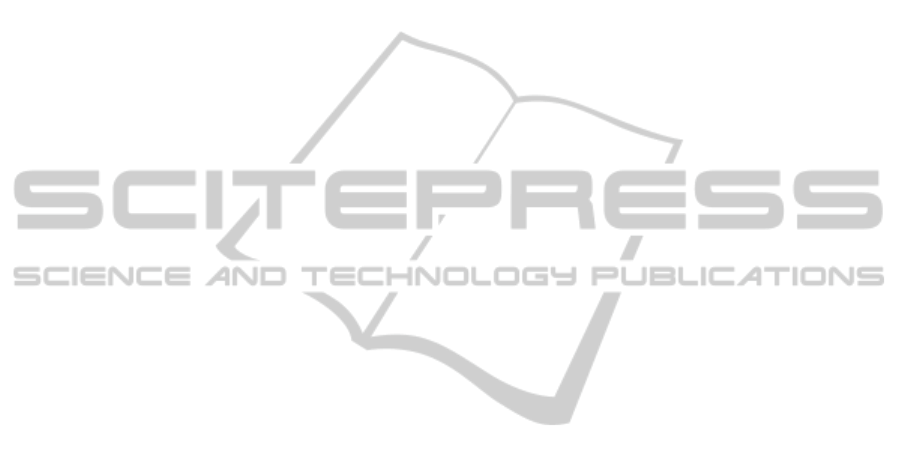
Figure-1 shows the correlation of the LSA
assigned scores (using the two spaces) to the score
assigned by expert-1 for each of the components,
and the correlation between the two experts.
Dimension-3 is the well-known dominant
misconception in the domain (that the heavy truck
will exert a greater force on the small car than the
other way around). We see that LSA is comparable
to the experts for component-1 (correct energy
formulation) and component-2 (correct momentum
formulation), although it performs lower than the
experts in component-3 (the dominant
misconception on the subject). Component-4 is the
correct force formulation of the problem.
It is important to note that TASA alone, which is
general space, produces results that are overall
comparable to the results produced with the addition
of a target-specific physics space. This plot shows
that by using human raters to rate a relatively small
number of documents, LSA can generally classify
documents on which it was not trained, with a
correlation which can be comparable to that of
different human experts. The exception in this case
seems to be the correct force formulation (which
states that the forces exerted by the car and truck on
each other are equal). It is not clear why this rubric
component faired so much worse than the rest. It is
worth noting that the experts were in perfect
agreement on this component (the correlation is one,
over all relevant answers).
Figure-3 shows results from two additional
questions, one in Astronomy, analyzed with TASA
and the same Physics test used in the Physics
questions, and one in Biology, analysed with TASA
and an open source Biology text. We see that in both
cases the system is consistently comparable to the
experts, especially when the general English space is
augmented with subject-specific texts.
3 CONCLUSIONS AND FUTURE
WORK
Although this is an ongoing project, the results so far
show that student essays, even of lengths that are
generally on the borderline of being too short for
treatment by LSA, can indeed give results that are
comparable to expert raters’, although some
challenges still remain. One of the questions that
will be important to the method, is the extend to
which the nature of the space in which the texts are
projected (eg. a general space like TASA versus a
discipline-specific space like the one we developed
from the textbooks) affects performance, and we
plan to conduct additional studies with a variety of
discipline-specific texts to address this question.
Perhaps the greatest limitation of the method is the
fact that, at this stage, the dominant misconceptions
are still being discovered “by hand” as it were, with
experts combing through large amounts of textual
data. Tools like Ed’s Tools can improve the logistics
of that search, and tools like LSA can improve the
logistics of identifying these misconceptions in very
large populations, but the discovery phase still
depends exclusively on experts. We plan to address
this limitation in future work, by using LSA to point
out possible new misconceptions that can then be
rated by content experts.
REFERENCES
Bloom, B. S., The 2 Sigma Problem: the Search for
Methods of Group Instruction as Effective as One-on-
One Tutoring, Educ. Res. 13, 4 (1984).
Garvin-Doxas, K. and M. W. Klymkowsky.
Understanding Randomness and its impact on Student
Learning: Lessons from the Biology Concept
Inventory (BCI). CBE Life Sci Educ 7: 227-233
(2008).
Garvin-Doxas, K., I. Doxas, and M.W. Klymkowsky. Ed's
Tools: A web-based software toolset for accelerated
concept inventory construction. Proceedings of the
National STEM Assessment Conference 2006. D.
Deeds & B. Callen, eds. Pp. 130-139 (2007).
Hake, R., Interactive engagement versus traditional
methods: A six-thousand-student survey of mechanics
test data for introductory physics courses, American
Journal of Physics, 66, pp. 64 (1998).
Kalas P, O'Neill A, Pollock C, Birol G., Development of a
meiosis concept inventory, CBE Life Sci Educ.
12(4):655-64. doi: 10.1187/cbe.12-10-0174 (2013).
Kintsch, E., D. Steinhart, G. Stahl, Developing
summarization skills through the use of LSA-based
feedback, Interactive Learning Environments, 8
(2000).
Klymkowsky, M.W. and K. Garvin-Doxas. Recognizing
Student Misconceptions through Ed's Tool and the
Biology Concept Inventory. PLoS Biology, 6(1): e3.
doi:10.1371/journal.pbio.0060003, (2008).
Landauer, T. K., P. Foltz, and D. Laham, An introduction
to Latent Semantic Analysis. Discourse Processes, 25,
259-284 (1998).
Landauer, T. K. and Dumais, S. T., A solution to Plato's
problem: the Latent Semantic Analysis theory of
acquisition, induction and representation of
knowledge. Psychological Review, 104(2), 211-240
(1997).
Libarkin, J., and S. Anderson, Development of the
Geoscience Concept Inventory, Proceedings of the
National STEM Assessment Conference, Washington
CSEDU2014-6thInternationalConferenceonComputerSupportedEducation
306