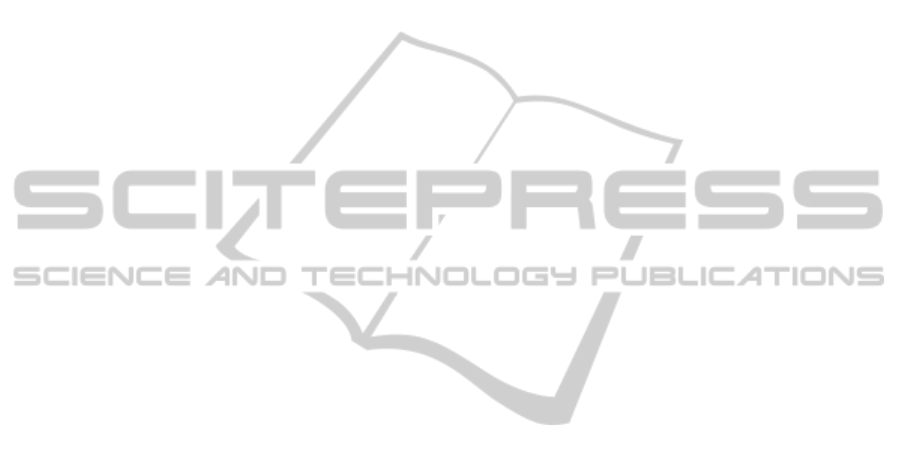
academic semester; (5) at the end of the first semester,
the students who give up had the average grades of
passing courses lower than completers, and finally,
(6) comparing Figure 1 and Figure 2, the student who
dropped out have a good grade about (74-78), but in
very few courses.
With respect of the still-enrolled students that
were observed in the investigated subset. We
observed that they maintained their enrolment active
by the year/semester of 2009-1. They have the
following features: (1) they are enrolled at about 5
courses during the undergraduate course; (2) they
decrease the number of passing course in each
semester; it is less than completers students but higher
than the ones who quit; (3) during the semesters, they
have higher possibilities of failing a course, compared
to others classes of students, and finally, (4) in the
first semesters they have less than one course with fail
or AF grading, in the following semesters the number
of course is above one; (5) the average of grade of
passing courses is the lowest comparing with two
other classes.
With regard to students who complete the course,
they have the following features: (1) maintain a high
number of courses enrolment in each semester; (2)
have a high rate of pass courses; (3) the number of fail
courses is close to zero throughout during the stay in
the university; (4) the average of courses with fail and
AF grading is less than one; (5) maintain the average
of pass courses close to the value of the CGPA until
the 8th semester of the degree. Students who
completed the degree present a regular behaviour
throughout all semesters; they enrolled in a high
number of courses and got high average passing
grades.
5 CONCLUSIONS
This paper compared five classification algorithms;
the results allowed us to investigate a three-class
problem related to the situation of the students of the
largest Brazilian PFBU. The SVM algorithm was
unfeasible to be used because the time spent to
construct the model was too high. The algorithm
Naïve Bayes has an interpretable model and its
numerical results can easily be converted into graphs.
In this paper, we presented a quantitative approach
using the results of such algorithm. The quality of the
results opens the novel possibilities of further
investigations, the development of an information
system capable of facilitating the management of
academic universities. The direct benefits of applying
data mining in this context are: (i) identify the course
students more likely to dropout and still-enrolled
students; (ii) allow the FPBU to use not only
statistical analysis of facing the problem of high
dropout rate.
REFERENCES
Baker, R. S. J. D., Yacef, K., 2009. The state of educational
data mining in 2009: A review and future visions. J. of
Educational Data Mining 1.1, 3-17.
Baker, R., Isotani, S., Carvalho, A., 2011. Mineração de
Dados Educacionais: Oportunidades para o Brasil.
Revista Brasileira de Informática na Educação.
Dekker, G., Pechenizkiy, M., Vleeshouwers, J., 2009.
Predicting Students Drop Out: A Case Study. In
Proceedings of the int. Conf. on Educational Data
Mining. Cordoba, Spain, 41-50.
Huang, S., 2011. Predictive Modeling and Analysis of
Student Academic Performance in an Engineering
Dynamics Course. Ph.D. Thesis, Utah State University,
Logan, USA.
Kotsiantis, S., Pierrakeas, C., Pintelas, P., 2003. Preventing
student dropout in distance learning using machine
learning techniques. KES, eds. V. Palade, R. Howlett &
L. Jain, Springer, v. 2774 LNCS, pp. 267–274, 1087-
6545.
Lobo, M. B. C. M., 2011. Panorama da evasão no ensino
superior brasileiro: aspectos gerais das causas e
soluções. Instituto Lobo & Associados Consultoria.
Lykourentzou, I. et al., 2009. Dropout prediction in e-
learning courses through the combination of machine
learning techniques, Computers & Education, v. 53, N.
3, pp. 950-965.
Manhães, L. M. B., Cruz, S. M. S., Costa, R. J. M, Zavaleta,
J. and Zimbrão. Identificação dos Fatores que
Influenciam a Evasão em Cursos de Graduação através
de Sistemas Baseados em Mineração de Dados: Uma
abordagem Quantitativa. In: VIII Simpósio Brasileiro de
Sistemas de Informação (SBSI 2012) (São Paulo, Brasil,
May 16-18, 2012), 468-479.
MEC, 1997. Ministério da Educação e Cultura. Diplomação,
Retenção e Evasão nos Cursos de Graduação em
Instituições de Ensino Superior Públicas.
Pal, S., 2012. Mining educational data to reduce dropout
rates of engineering students. International Journal of
Information Engineering and Electronic Business
(IJIEEB), 4(2), 1.
Romero, C., Ventura, S., 2010. Educational Data Mining: A
Review of the State of the Art, Systems, Man, and
Cybernetics, Part C: Applications and Reviews, IEEE
Transactions, v.40, n.6, 601-618.
Romero, C., Ventura, S., 2013. Data Mining in Education.
Wiley Interdisciplinary Reviews: Data Mining and
Knowledge Discovery, In Press. v. 3, n. 1, 12-27.
Soares, I. S. 2006. Evasão, retenção e orientação acadêmica:
UFRJ. In: Anais do XXXIV COBENGE - Congresso
Brasileiro de Ensino de Engenharia.
TheImpactofHighDropoutRatesinaLargePublicBrazilianUniversity-AQuantitativeApproachUsingEducational
DataMining
129