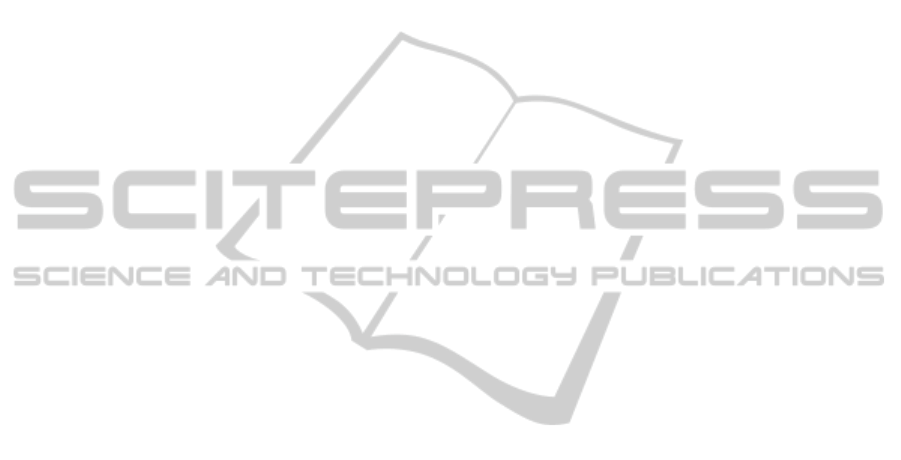
ideas of this paper. The section will explain the
concept of ubiquitous logistics and its preceding
background. Furthermore, the section will cover
aspects of data integration that are reused in the
introduced approach of this paper.
2.1 Autonomous Logistics
Interpretation of logistics as a multi-agent system
(MAS) is discussed in literature for several years
(Moore et al., 1997). In 2005, Davidsson et. al.
published a survey about the use of MAS in
transportation and traffic management (Davidsson et
al., 2005). Findings indicate that many logistics
problems have characteristics similar to those of
agent-based systems. A similar argumentation is
suggested for the collaborative research centre 637
for autonomous logistics in Bremen, Germany
(Scholz-Reiter et al., 2004). According to (Gehrke et
al., 2010), autonomous logistics can be enabled by
situation-aware agents, actively gaining information
about their surroundings. Based on an agent’s
observations, decisions can be made about logistics
processes. An example for the implementation of
situation-aware agents in logistics is the intelligent
container that is described in (Lang et al., 2011). A
comprehensive study on multi-agent coordination
enabling autonomous logistics is provided by
(Schuldt, 2012).
The argumentation of autonomous agents in
logistics offers far reaching possibilities for future
urban freight transport. In order to utilize
possibilities, the focus of planning and control of
urban transport needs to shift from a centralized
perspective towards a decentralized one. At the same
time, efficiency in urban freight transport is
increased by taking into account all available
logistics resources an urban environment provides.
2.2 Ubiquitous Logistics
In the ubiquitous logistics concept, public and
private transportation means that are able to
transport goods (parcels) within a city are considered
as logistics resources of that same city (i.e. private
vehicles, trucks, trams, buses, etc.). Primary
objective of the concept is to avoid additional traffic
for freight transport by utilizing existing logistics
resources best.
In order to manage the multitude of logistics
resources within a city, transported parcels feature
an autonomous, situation-aware agent that can be
human or artificial. The agent collects information
and takes decisions required to complete the
scheduled transportation task for a certain parcel.
The decision process each agent has to perform is
based on a multi-criteria problem. The problem
concerns the selection and realization of an optimum
route from the current location to a target destination
within a dynamic environment. Potential routes are
created and destroyed dynamically based on the
availability of local logistics resources. A situation-
aware agent picks logistics resources in order to
make progress against its scheduled transportation
task. Completion of a transportation task might
require taking different logistics resources (i.e.
vehicles or transportation modes).
The assignment of parcel and resource depends
on criteria like transportation time, load efficiency,
service level, stock level, as well as transportation
and storage cost (Nyhuis and Wiendahl, 2013).
Furthermore, environmental dynamics need to be
taken into account including for instance traffic
condition, delivery order changes, weather
conditions, and availability of logistics resources.
The decision process requires large amounts of
most recent information from distributed sources
like other agents, traffic management systems and
logistics providers. Therefore, the underlying
information infrastructure needs to be capable of
handling several thousands of decentralized
information requests and offers at the same time. In
this infrastructure, each information offer is
represented by a specific service. Services can be
provided by different organizations with different
data source structures. Complex decisions require
that several information services are requested and
integrated.
2.3 Data Integration
Information services are based on data sources.
From a technical point of view, significant data
sources in logistics are data processing systems, data
storage systems and sensors deployed in the field.
The data processing systems are decision making
agents, infrastructure management systems and other
necessary systems to handle processes in logistics.
Data storage systems are used to aggregate data in
logistics. This includes for instance generic data
bases, data warehouse systems, warehouse
management systems and product lifecycle
management systems. Sensors are typically used to
collect field data. This data may contain
measurements like temperature, light intensity and
mechanical forces. The described data is allocated in
different ways, for instance as a CSV file, text file or
database. It can be accessed by using HTTP (hyper
SemanticDataIntegrationforUbiquitousLogistics-AnApproachSupportingAutonomousLogisticsinUrban
Environments
653