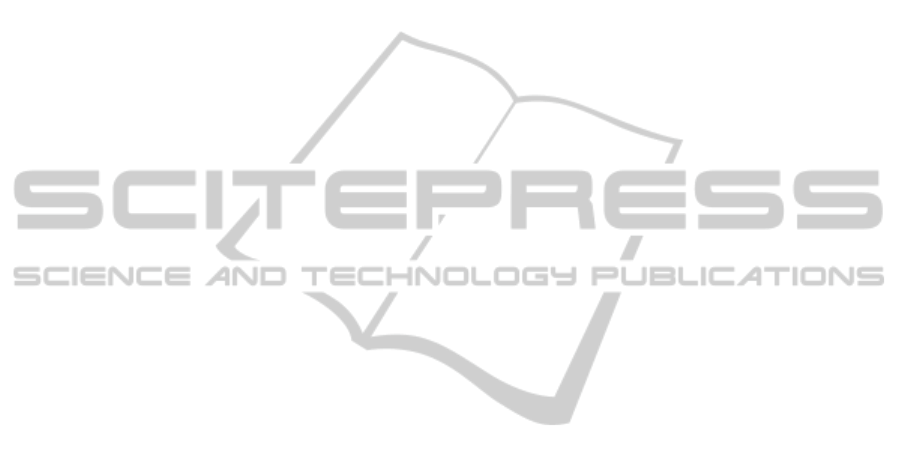
ticipation. Teacher’s peers see when they col-
lect these rewards. These extrinsic rewards are
much more effective if people can use them for
bragging rights, rather than just having some
extra trophy graphic that nobody else will see.
A final remark on the game design concerns the
whole presentation of the metaservices. The de-
sign, the look and feel, the interaction style and the
communication process of the e-learning environment
need a specific care and an incremental production of
mock-ups anytime that new users requirements ap-
pear. Moreover, it is import to test our prototype as
early as possible. One of the most repeated mistake
is to make assumptions about how the target audience
will use the product. The only way the designers can
understand it is to put the system in front of them,
watch them use it, and to document the experience in
order to pay attention to how long it takes to make the
correct input, and to watch through teachers’ eyes for
seeing where they look first on the screen mockups.
5 CONCLUDING REMARKS
The main goal of the e-Teaching Assistant is to of-
fer a new opportunity for supporting teachers by ex-
ploiting the contributions of a SN able to enhance
and enrich didactic contents proposed by their mem-
bers. To this aim, the paper proposes a social oriented
solution based on three metaservices for exchang-
ing high quality didactic materials, retrieving content
through a computationally intelligent recommenda-
tion service and stimulating the teachers involvement
through gamification strategies. Other metaservices
are under design for offering a semi-automatic combi-
nation of modules according to the requirements and
skills characterizing them and by fitting the teachers’
expectation according to their profile and background.
REFERENCES
ADL - Advances Distributed Learning (2004). Sharable
content object reference model (SCORM). Overview.
http://www.adlnet.gov/About/alexandria/default.aspx.
Apolloni, B., Bassis, S., Malchiodi, D., and Pedrycz, W.
(2008). The Puzzle of Granular Computing, vol-
ume 138 of Studies in Computational Intelligence.
Springer Verlag.
Atkins, D. E., Brown, J., and Hammonds, A. (2007). A
review of the open educational resources (OER)
movement: achivements, challenges, and new
opportunities. www.hewlett.org/uploads/files/
ReviewoftheOERMovement.pdf.
Bell, R., Koren, Y., and Volinsky, C. (2009). IEEE Com-
puter. Matrix factorization techniques for recom-
mender systems, 42(8):30–37.
Breese, J. S., Heckerman, D., and Kadie, C. (1998). Empir-
ical analysis of predictive algorithms for collaborative
filtering. In Proc. of the 14th Conf. on Uncertainty in
Artificial Intelligence, Madison, WI, July 1998.
Brusilovsky, P. (1996). Methods and techniques of adaptive
hypermedia. User modeling and user-adapted inter-
action, 6(2):87–129.
Brusilovsky, P. (2012). Adaptive hypermedia for education
and training. Adaptive Technologies for Training and
Education, 46.
Deterding, S., Sicart, M., Nacke, L., O’Hara, K., and Dixon,
D. (2011). Gamification. using game-design elements
in non-gaming contexts. In CHI ’11 Extended Ab-
stracts on Human Factors in Computing Systems, CHI
EA ’11, pages 2425–2428, New York, USA. ACM.
eLearn Magazine Staff and Contributors (2011). Predictions
for e-learning in 2011. eLearn, 2011(1).
Frias-Martinez, E., Chen, S. Y., and Liu, X. (2006). Survey
of data mining approaches to user modeling for adap-
tive hypermedia. IEEE Trans. on Systems, Man, and
Cybernetics, Part C, 36(6):734–749.
IEEE (2006). Standard for Learning Object Metadata
(LOM): Conceptual Schema IEEE 1484.12.1.
Ivino, S., Bocconi, S., Boytchev, P., Earp, J., and Sarti, L.
(2009). Sharing digital resources in teacher education:
an ontology-based approach. In Demetra EOOD.
Kim, K. J. and Ahn, H. (2012). Hybrid recommender sys-
tems using social network analysis. World Academy
of Science Engineering and Technology, 64.
Melville, P., Mooney, R. J., and Nagarajan, R. (2002).
Content-boosted collaborative filtering for improved
recommendations. In Proc. of the 18th National Conf.
on Artificial Intelligence (AAAI-02), pages 187–192.
Mooney, R. J. and Roy, L. (2000). Content-based book rec-
ommending using learning for text categorization. In
Proc. of Digital Libraries Conf., pages 195–204.
Mulwa, C., Lawless, S., Sharp, M., Arnedillo-Sanchez, I.,
and Wade, V. (2010). Adaptive educational hyperme-
dia systems in technology enhanced learning: a lit-
erature review. In Proc. of the 2010 ACM Conf. on
Information technology education, pages 73–84.
Muntean, C. I. (2011). Raising engagement in e-learning
through gamification. In The 6th International Conf.
on Virtual Learning ICVL 2011, pages 323–329.
Nah, F. F.-H., Telaprolu, V. R., Rallapalli, S., and Venkata,
P. R. (2013). Gamification of education using com-
puter games. In HCI (15), pages 99–107.
Pazzani, M. J. and Billsus, D. (1997). Learning and revis-
ing user profiles: The identification of interesting web
sites. Machine Learning, 27(3):313–331.
Raymer, R. (2011). Gamification: Using game mechanics
to enhance elearning. eLearn Magazine, 2011(9):3.
Schein, A., Popescul, A., Ungar, L. H., and Pennock, D. M.
(2002). Methods and metrics for cold-start recommen-
dations. In Proc. of the 25th annual Intern. Conf. on
Research and Development in Information Retrieval
(SIGIR), pages 253–260, NY, USA, 2002. ACM.
CSEDU2014-6thInternationalConferenceonComputerSupportedEducation
574