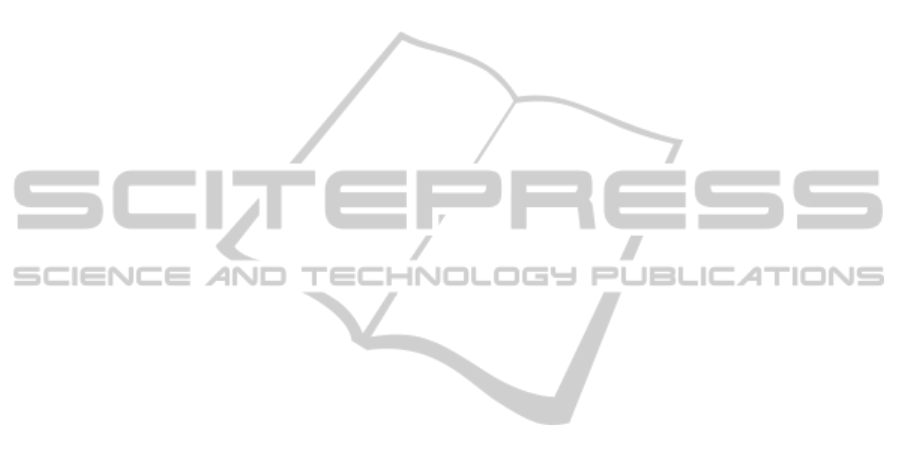
PASSED_EXAM_TOOK_EXAM, were considered
for the principal component analysis based on their
correlation matrix. The proportion of variability
explained by the first, the first two and the first three
components is respectively 58%, 74% and 84%, and
the expression of the first three components is the
following:
PC1= 0.40 TOOK_EXAM - 0.37OPP_TAKE_EXAM
+ (-0.39)·NUM_EXAM_PASS +
0.50•PASSED_EXAM_REGISTERED +
0.43•PASSED_EXAM_TOOK_EXAM +
0.34 AV_MARK_10 (1)
PC2= (-0.59)•TOOK_EXAM + 0.31 OPP_TAKE_
EXAM + (-0.46)•NUM_EXAM_PASS -
0.23 PASSED_EXAM_REGISTERED +
0.33 PASSED_EXAM_TOOK_EXAM +
0.42•AV_MARK_10 (2)
PC3= 0.03 TOOK_EXAM + 0.45 OPP_
TAKE_EXAM + (-0.17)•NUM_EXAM_PASS +
0.26 PASSED_EXAM_REGISTERED +
0.46 PASSED_EXAM_TOOK_EXAM -
(-0.70)•AV_MARK_10 (3)
The first component is clearly a weighted average of
the different variables, assigning positive weights for
the variables for which high values indicate good
results and negative weights for the two variables for
which low values indicate good results
OPP_TAKE_EXAM and NUM_EXAM_PASS. The
modules that score higher in this first component can
thus be considered as the most successful modules,
in terms of student behaviour and results. As for the
second component the following interpretation is
suggested: PC2 measures the difference between the
difficulty perceived by the students before the exam
and the actual difficulty to pass the module. Indeed
it can be written as the sum of two terms: (-
TOOK_EXAM - PASSED_EXAM_REGISTERED +
OPP_TAKE_EXAM) and (AV_MARK_10 +
PASSED_EXAM_TOOK_EXAM - NUM_EXAM_
PASS). The first term takes its highest values for
modules where a low proportion of students take the
exam and therefore the proportion of students that
pass the module with respect to the registered
students is low and the number of opportunities used
to actually take the exam for the first time is high.
The influence of the different factors (Stage,
temporal location in the academic year, course, and
assigned ECTS value) on the principal components
scores was explored. Differences between the
different courses at the School for Industrial
Engineering was found for the PC2, where two
courses scored typically higher: the Industrial
Management Engineer course and the Automatism
and Industrial Electronics course, where a significant
part of the students work and therefore have a higher
tendency to use several opportunities before taking
an exam. Principal components plots (PC2 versus
PC1) were also provided to follow the temporal
evolution of the module scores. As an example, the
scores obtained for the considered years by the
modules of the first stage of the Industrial
Engineering course are shown in Figure 1. This kind
of plot allows monitoring the evolution, for a given
module, of the student behaviour and results and
detecting possible difficulties.
4.2 Data-Set S
For dataset S five variables MARK_SLC,
DURATION, MARK_GRAD, OPP_TAKE_EXAM,
NUM_EXAM_PASS were considered for the
principal component analysis based on their
correlation matrix. The proportion of variability
explained by the first, the first two and the first three
components is respectively 55%, 75% and 85%, and
the expression of the first three components is:
PC1= 0.37·MARK_SLC + (-0.47)·DURATION +
+0.46·MARK_GRAD - 0.45·OPP_TAKE_EXAM-
-0.46 NUM_EXAM_PASS (4)
PC2= -0.56 MARK_SLC+ -0.48 DURATION -
0.37·MARK_GRAD -0.54·OPP_TAKE_EXAM +
0.19 NUM_EXAM_PASS (5)
PC3= 0.74 MARK_SLC - 0.06 DURATION -
0.42·MARK_GRAD -0.25 OPP_TAKE_EXAM +
0.46·NUM_EXAM_PASS (6)
As for dataset M, the first component is easy to
interpret as a global score of the graduated student’s
success: it consists of a weighted average of all
variables, with positive weights for variables that
translate positively in terms of the student’s results
and negative weights for variables for which high
values would indicate worst results. The second
component is interpreted as the sum of two terms:
MARK_SLC+MARK_GRAD - NUM_EXAM_
PASS and DURATION + OPP_TAKE_EXAM.
The weights have been omitted, which reflect on
one hand the student’s performance (their marks and
the number of times they need to take an exam to
pass the module) and on the other hand their
apprehension before taking an exam
(OPP_TAKE_EXAM) which have of course an
influence on the duration of their studies. The
CSEDU2014-6thInternationalConferenceonComputerSupportedEducation
586