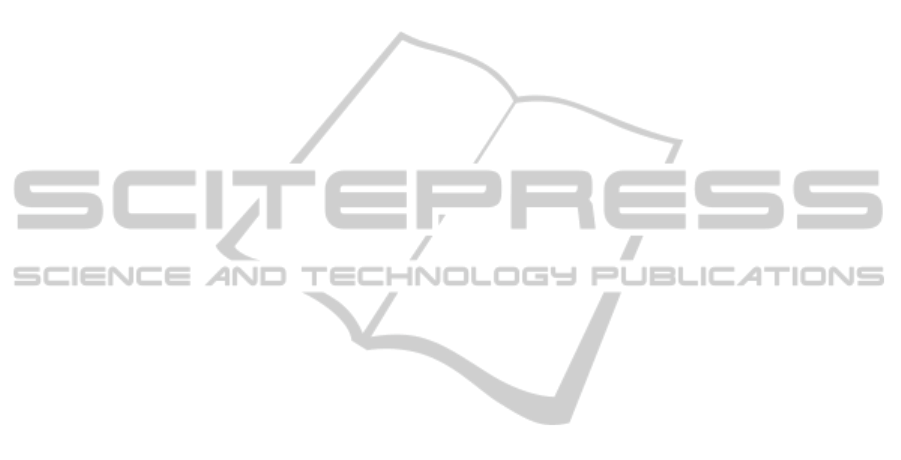
less successful in cooperation with intuitive learners,
who prefer abstract materials.
The research’s results also show that students
with low working memory capacity are more tended
to visual learning style, but this doesn’t mean that
learners with visual learning style have low working
memory capacity. It could be explained by dual
coding theory of multimedia learning.
The sensing learning style requires concrete and
specified learning materials. The sensing learners are
more careful and attentive during a learning process,
but intuitive learners are more tended to abstract
materials and they have tendency of not being
patient and careful.
The results of sequential/global dimension shows
that sequential learners are tended to understand the
concepts by building them from smaller parts to the
whole solution. We also noticed rather close relation
between sensing/intuitive and sequential/global
learning dimensions. These dimensions correlated
with each other.
5 CONCLUSIONS
This paper analyses the learners’ cognitive traits and
learning styles. Felder-Silverman learning style
model and cognitive trait model was used for the
research analysis.
Considering the relationship between cognitive
traits and learning styles it is possible to obtain
additional information about a learner, which could
improve the overall students’ mode. The research
shows that students with active, sensing visual and
global learning style have lower memory than
reflective, intuitive and sequential. This could help
to support learner’s cognitive load with appropriate
instructional design automation and integration in
learning systems.
Within the research the use of electronic learning
materials at high schools was analysed, and it was
concluded that most of the materials do not meet the
necessary requirements for supporting students’
cognitive traits and learning styles.
Learning styles can improve identification of
cognitive traits; if the learning style is already
detected then it will improve indication of cognitive
traits. But at the same time cognitive traits can also
help to identify learning styles. Such interaction can
better show students working characteristics and
provide the analyses of not only learning styles but
also the cognitive traits of students. This analysis
can lead to more accurate representation of materials
which will give ability to provide learning without
cognitive overload. Such analysis can improve
pedagogical models to provide more adaptive
learning, with better effect.
The further work is necessary on the statistical
analyses of survey results which could allow analyse
the correlation between different learning
dimensions. It would also be useful to make more
explicit analyses on students’ behaviour and to find
the ability to detect automatically the learning style
from the student’s behaviour in learning system.
Definitely more research on learning styles and
cognitive traits should be made, to provide more
adaptive electronic learning materials.
ACKNOWLEDGEMENTS
This research is part of a project „Competence
Centre of Information and Communication
Technologies” run by “IT kompetences centrs” Ltd,
contract No. L-KC-11-0003, co-financed by
European Regional Development Fund.
REFERENCES
Bannert, M., 2002. Managing cognitive load — recent
trends in cognitive load theory. Learn. Instr. 12, 139–
146.
Chen, S.Y., Macredie, R.D., 2002. Cognitive styles and
hypermedia navigation: Development of a learning
model. J. Am. Soc. Inf. Sci. Technol. 53, 3–15.
Fadel, C., Lemke, C., 2008. Multimodal Learning Through
Media : What the Research Says. Cisco Syst. 1–24.
Felder, R.M., Silverman, L.K., 1998. LEARNING AND
TEACHING STYLES. Engr. Educ. 78, 674–681.
Felder, R.M., Soloman, B.A., 1997. Index of Learning
Styles questionaire [WWW Document]. URL http://
www.engr.ncsu.edu/learningstyles/ (accessed 9.20.13).
Gerven, P.W.M. Van, Paas, F.G.W.C., Merrie, J.J.G. Van,
2002. Cognitive load theory and aging : effects of
worked examples on training efficiency. Learn. Instr.
12, 87–105.
Hollender, N., Hofmann, C., Deneke, M., Schmitz, B.,
2010. Integrating cognitive load theory and concepts
of human–computer interaction. Comput. Human
Behav. 26, 1278–1288.
Honey, P., Mumford, A., 1982. The Manual of Learning
Styles. P. Honey & L. Mumford.
Kalyuga, S., 2011. Effects of information transiency in
multimedia learning. Procedia - Soc. Behav. Sci. 30,
307–311.
Kolb, D.., 1984. Experiential learning : experience as the
source of learning and development. Engelwood
Cliffs, NJ: Prentice Hall.
Kuljis, J., Liu, F., 2005. A Comparison of Learning Style
Theories on the Suitability for elearning. Web
ICEIS2014-16thInternationalConferenceonEnterpriseInformationSystems
142