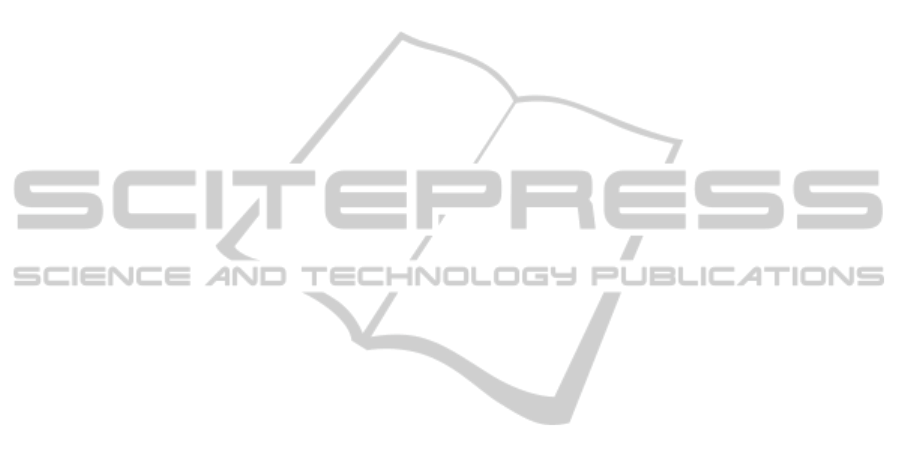
5 CONCLUSION
In this paper, we have proposed a new approach of
detecting social interests. This approach is based on
analyzing the tagging behaviour of each user. In fact,
the analyze aims to extract the most accurate tags ac-
cording to their relevance to the content of the tagged
resources. We have tested our approach in Delicious
database. The validation of the results is done by
comparing the tags of each user with the result of tags
issued from our approach using users’ neighbours (his
community). The proposed approach is able to de-
tect potential user’s interests by analyzing their social
behaviour. Moreover, it improves the quality of the
detected interests (tags) by decreasing implicitly their
ambiguity. Then, it could be used for a purpose of
adaptation since it provides a solution for detecting
users’ interests.
In future works, we will validate our approach ac-
cording to another type of neighbours such as the ego-
centric network. This test allows us to deduce the
neighbours which reflect the most the user’s interests.
REFERENCES
Astrain, J. J., Cordoba, A., Echarte, F., and Villadangos,
J. (2010). An algorithm for the improvement of tag-
based social interest discovery. pages 49–54.
Cazabet, R., Amblard, F., and Hanachi, C. (2010). Detec-
tion of overlapping communities in dynamical social
networks. In 2010 IEEE Second International Confer-
ence on Social Computing (SocialCom), pages 309–
314.
Godoy, D. and Amandi, A. (2008). Hybrid content and
tag-based profiles for recommendation in collabora-
tive tagging systems. In Latin American Web Confer-
ence, 2008. LA-WEB ’08., pages 58–65.
Ivan, C., Peter, B., and Tsvi, K. (2011). HetRec ’11: Pro-
ceedings of the 2Nd International Workshop on In-
formation Heterogeneity and Fusion in Recommender
Systems. ACM, New York, NY, USA.
Kim, H.-N., Alkhaldi, A., El Saddik, A., and Jo, G.-
S. (2011). Collaborative user modeling with user-
generated tags for social recommender systems. Ex-
pert Systems with Applications, 38(7):8488–8496.
Ma, Y., Zeng, Y., Ren, X., and Zhong, N. (2011). User in-
terests modeling based on multi-source personal infor-
mation fusion and semantic reasoning. In Proceedings
of the 7th International Conference on Active Media
Technology, AMT’11, page 195205, Berlin, Heidel-
berg. Springer-Verlag.
Meo, P. D., Quattrone, G., and Ursino, D. (2010). A query
expansion and user profile enrichment approach to im-
prove the performance of recommender systems op-
erating on a folksonomy. User Modeling and User-
Adapted Interaction, 20(1):41–86.
Mezghani, M., Zayani, C. A., Amous, I., and Gargouri, F.
(2012a). An extended architecture for adaptation of
social navigation. In Krempels, K.-H. and Cordeiro,
J., editors, WEBIST 2012 - Proceedings of the 8th In-
ternational Conference on Web Information Systems
and Technologies, Porto, Portugal, 18 - 21 April,
2012, pages 540–545. SciTePress.
Mezghani, M., Zayani, C. A., Amous, I., and Gargouri, F.
(2012b). A user profile modelling using social anno-
tations: A survey. In Proceedings of the 21st Inter-
national Conference Companion on World Wide Web,
WWW ’12 Companion, page 969976, New York, NY,
USA. ACM.
Milicevic, A. K., Nanopoulos, A., and Ivanovic, M. (2010).
Social tagging in recommender systems: a survey of
the state-of-the-art and possible extensions. Artificial
Intelligence Review, 33(3):187–209.
Rebai, R. Z., Zayani, C. A., and Amous, I. (2013). An adap-
tive navigation method for semi-structured data. In
Morzy, T., Hrder, T., and Wrembel, R., editors, Ad-
vances in Databases and Information Systems, num-
ber 186 in Advances in Intelligent Systems and Com-
puting, pages 207–215. Springer Berlin Heidelberg.
Song, Y., Zhang, L., and Giles, C. L. (2011). Automatic tag
recommendation algorithms for social recommender
systems. ACM Trans. Web, 5(1):4:14:31.
Tchuente, D., Canut, M.-F., Jessel, N., Peninou, A., and
Sdes, F. (2013). A community-based algorithm for
deriving users profiles from egocentrics networks: ex-
periment on facebook and DBLP. Social Network
Analysis and Mining, 3(3):667–683.
Vallet, D., Cantador, I., and Jose, J. M. (2010). Personaliz-
ing web search with folksonomy-based user and doc-
ument profiles. In Gurrin, C., He, Y., Kazai, G., Kr-
uschwitz, U., Little, S., Roelleke, T., Rger, S., and Ri-
jsbergen, K. v., editors, Advances in Information Re-
trieval, number 5993 in Lecture Notes in Computer
Science, pages 420–431. Springer Berlin Heidelberg.
White, R. W., Bailey, P., and Chen, L. (2009). Predicting
user interests from contextual information. In Pro-
ceedings of the 32Nd International ACM SIGIR Con-
ference on Research and Development in Information
Retrieval, SIGIR ’09, page 363370, New York, NY,
USA. ACM.
Zayani, C. A., Pninou, A., Canut, C. M.-F., and Sedes,
F. (2007). Towards an adaptation of semi-structured
document querying. In Doan, B.-L., Jose, J. M.,
and Melucci, M., editors, Proceedings of the CIR’07
Workshop on Context-Based Information Retrieval in
conjunction with CONTEXT-07, Roskilde, Denmark,
20 August 2007, volume 326 of CEUR Workshop Pro-
ceedings. CEUR-WS.org.
AnalyzingTaggedResourcesforSocialInterestsDetection
345