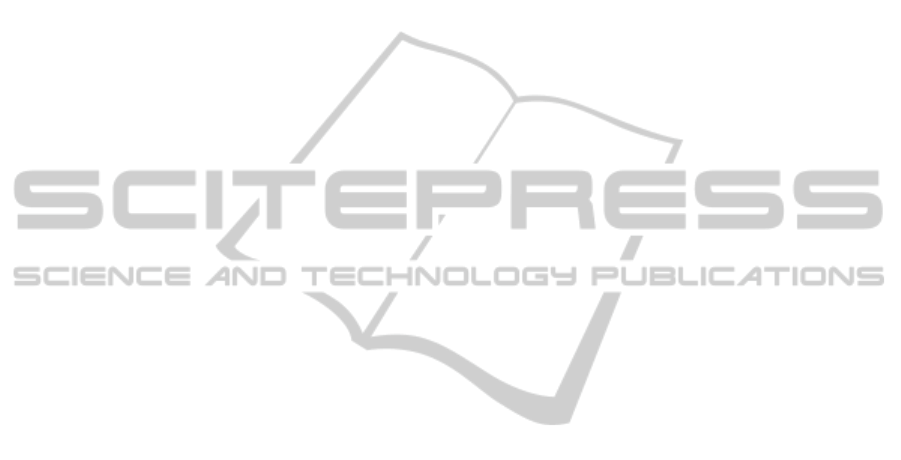
Media Labs pioneered the use of wireless tags that
captures relational information as well as body
language, including tone, volume and pitch, from the
communicator. The tags, whose size is similar to
those of name cards can be worn like any ordinary
ID card, are unobtrusive in nature. It ubiquitously
captures the ‘when’, ‘who’, ‘whom’ and ‘how’ of
the communication but not the ‘what’. In other
words, it does not capture content. Therefore, at any
point in time, it is possible for the communication
pattern of individuals to be captured. Furthermore,
with the use of Radio Frequency ID tags also
available these days, it is possible for these tags to
be used to capture relational data, particularly when
individuals deal with non human resources such as
computers, machines, and so on. Pentland used the
patterns of communication captured to associate
with individual and team success. In reality, the
association can be made with other social
phenomenon such as creativity, coordination, etc.
In a similar manner, reverting back to the
example of the hospital ED, it becomes possible for
us to understand how organisational complexity
associates with operational performance such as
patient queues and waiting times. Here, one would
capture the organisational complexity of the ED as a
whole, having these tags in place in both human and
non-human resources. This enables us to capture all
relations and interdependencies at various points in
time. It is also important that at these points in time,
data relating to the dependent variables - patient
queues and waiting times, for instance, should also
be recorded. To illustrate, the relational snapshots
can be taken at every 3 hours in a 24 period, yielding
8 data points. If one does this for a week, there
would be 56 data points and for two weeks, 112 data
points. A distribution of the NoC and DoI can then
be computed, and the mean values for each of these
variables can serve as the relative cutpoints for the
complexity profiles to be obtained. In this manner,
one can compare which organisational complexity
states perform better (e.g. when at the ‘simple’
profile or at the ‘complicated’ profile) in terms of
operational performance.
5 CONCLUSIONS
Complexity is still a controversial topic, one that is
multi-faceted in epistemological stance, in definition
and in oeprationalisation. In general, literature in
complexity studies can be categorised in to
deterministic, algorithmic and aggregate complexity.
In this position paper, I focus particularly on
aggregate complexity and argue that it is possible to
capture structural aspects of complexity in both
static and dynamic forms. Based on Kannampallil’s
theoretical framework for computing complexity, it
is proposed here that complexity, in an aggregate
sense, can be evaluated in terms of (i) the number of
components (NoC) there are within a socio-technical
organisation and (ii) the degree of interrelatedness
(DoI) between these components.
Given these variables, it is then possible to
characterise complexity in terms of simple,
complicated, relatively complex and complex
profiles. These profiles serve as useful toolkits for
indicating the complexity level a team, a department
or the entire organisation is at for useful
interventions or insights to be made. Adapting the
ideas of Pentland, I also argue that with
technological advances in Information Systems,
organisations are now able to capture relational or
social network data with relative ease, to construct
useful network and complexity maps of individuals,
teams and organisations in real time.
REFERENCES
Bar-Yam, Y. (2006). Improving the Effectiveness of
Health Care and Public Health: A Multiscale Complex
Systems Analysis. American Journal of Public Health,
96, 459-466.
Chung, K. S. K., Young, J., & White, K. (2013, 25 - 28
August). Towards a Network-enabled Complexity
Profile for Examining Responsibility for Decision-
making by Healthcare Professionals. Paper presented
at the International Symposium on Network Enabled
Health Informatics, Biomedicine and Bioinformatics,
Niagara Falls, Canada.
Johnson, N. (2007). Simply Complexity. Oxford: Oneworld
Publications.
Kannampallil, T. G., Schauer, G. F., Cohen, T., & Patel,
V. L. (2011). Considering Complexity in Healthcare
Systems. Journal of Biomedical Informatics, 44(6),
943-947.
Manson, S. M. (2001). Simplifying Complexity: A
Review of Complexity Theory. Geoforum, 32(3), 405-
414.
Mitchell, M. (2009). Complexity: A Guided Tour. New
York: Oxford University Press.
Mitchell, R. E., & Trickett, E. J. (1980). Task Force
Report: Social Networks as Mediators of Social
Support. An Analysis of the Effects and Determinants
of Social Networks. Community Mental Health
Journal, 16(1), 27-44.
Pentland, A. S. (2012). The New Science of Building
Great Teams. Harvard Business Review, 90(4), 60-70.
Pfeil, U., & Zaphiris, P. (2009). Investigating Social
Network Patterns within an Empathic Online
ICEIS2014-16thInternationalConferenceonEnterpriseInformationSystems
470