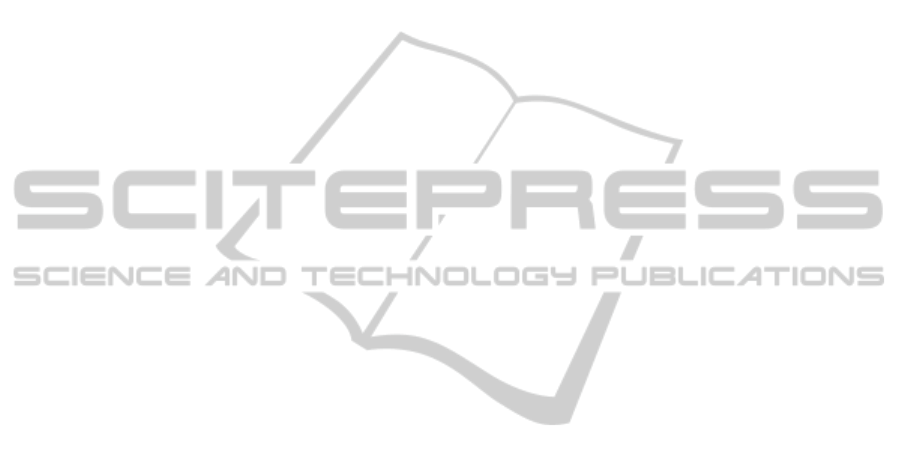
for working with data from social network sites. To-
gether we published a paper describing the guidelines
and applying them to our research.
Using these guidelines and elements from value
sensitive design (Friedman et al., 2006), we con-
cluded that our research was ethically justifiable as
a value trade-off taking into account the interests of
people investigated, people who’s account is included
in a candidate set as false positive, the ISZW and all
Dutch citizens. One important factor was also that,
although requesting welfare support is not really by
choice, the receiver is not obliged to do so. By re-
questing welfare support, someone voluntarily gives
up some privacy to allow the government to investi-
gate if he rightfully does so. This aspect also shows
that using our design to investigate other groups has
to be considered for its own merits. For more details,
we refer to (Been, 2013).
8 CONCLUSIONS
Fraud risk analysis on data from formal information
sources, being a paper reality, suffers from blindness
to false information. Moreover, the very act of provid-
ing false information is a strong indicator for fraud.
As a step towards the vision of harnessing real-world
data from social media and internet for fraud risk
analysis, we present a novel iterative search, mon-
itor, and match approach for finding on-line pres-
ences of people. The approach needs only limited
name/address input data available to governmental or-
ganizations responsible for fraud detection. A real-
world experiment showed that Twitter accounts can
be effectively found: from a voluntary sign-up subject
group of 22 subjects, the correct account was almost
always captured. Our initial attempt at pinpointing
the correct account for each subject proved ineffec-
tive, but we expect this to be a matter of choosing
other features and classification techniques, since the
correct account is included and rich data is gathered.
We also experimented with a larger subject group of
85 subjects from the ISZW. Finally, an analysis is
given of the ethics surrounding the application of such
technology for fraud risk analysis. We aim to extend
IMatcher to search for more kinds of on-line pres-
ences such as other social networks, extract and mon-
itor more characteristics, and improve the person vs.
on-line presence matching.
ACKNOWLEDGMENTS
This publication was supported by the Dutch national
program COMMIT/.We thank Victor de Graaff for neo-
geo support and ISZW for providing the data.
REFERENCES
Back, M. D., Stopfer, J. M., Vazire, S., Gaddis, S.,
Schmukle, S. C., Egloff, B., and Gosling, S. D.
(2010). Facebook profiles reflect actual person-
ality, not self-idealization. Psychological science,
21(3):372–374.
Been, H. (2013). Finding you on the internet: Entity resolu-
tion on twitter accounts and real world people. Mas-
ter’s thesis, Univ. Twente, Netherlands.
Brizan, D. and Tansel, A. (2006). A survey of entity resolu-
tion and record linkage methodologies. Communica-
tions of the IIMA, 6(3):41–50.
Friedman, B., Kahn Jr, P. H., and Borning, A. (2006). Value
sensitive design and information systems. Human-
computer interaction in management information sys-
tems: Foundations, 5:348–372.
Habib, M. B. and van Keulen, M. (2013). A generic
open world named entity disambiguation approach
for tweets. In KDIR 2013, Vilamoura, Portugal.
SciTePress.
Hofmann, C., Horn, E., Keller, W., Renzel, K., and
Schmidt, M. (1996). The field of software architec-
ture. Technical Report TUM-I9641, TU Munich, Ger-
many.
Inspectie SZW (2012). Bestandskoppeling bij fraudebestri-
jding. Technical Report Nvb-Info 12/062, Ministerie
van Sociale Zaken en Werkgelegenheid.
Jain, P. and Kumaraguru, P. (2012). Finding nemo: Search-
ing and resolving identities of users across online so-
cial networks. Technical Report arXiv:1212.6147,
arXiv.
Minder, P. and Bernstein, A. (2011). Social network aggre-
gation using face-recognition. In SDoW 2011, Bonn,
Germany, volume 830. CEUR. ISSN 1613-0073.
Narayanan, A. and Shmatikov, V. (2009). De-anonymizing
social networks. In 30th IEEE Symposium on Security
and Privacy, pages 173–187.
Perito, D., Castelluccia, C., Kaafar, M. A., and Manils,
P. (2011). How unique and traceable are user-
names? In Privacy Enhancing Technologies, pages
1–17. Springer.
Suchanek, F., Kasneci, G., and Weikum, G. (2007). Yago:
a core of semantic knowledge. In WWW 2007, Banff,
Canada, pages 697–706.
van Keulen, M. (2012). Managing uncertainty: The road
towards better data interoperability. IT - Information
Technology, 54(3):138–146.
Veldman, I. (2009). Matching profiles from social network
sites. Master’s thesis, Univ. Twente, Netherlands.
FindingYouontheInternet-AnApproachforFindingOn-linePresencesofPeopleforFraudRiskAnalysis
705