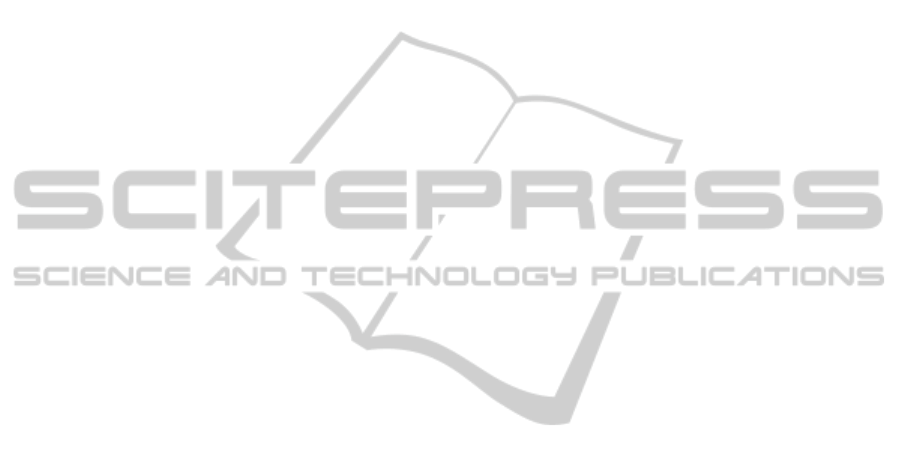
flags could be understood as acceptable given the
types of services rendered or due to the provider’s
operating environment, there was a preponderance of
evidence suggesting that at least 12 of these 17
providers (71%) with three or more flags should be
immediately referred for audit and potentially to law
enforcement.
6 CONCLUSIONS AND FUTURE
RESEARCH
We structure our design science contribution
according to the Hevner et al. (2004) framework and
address a relevant problem in healthcare fraud
detection. This paper offers an artifact and a
description of a method for applying outlier
detection to healthcare fraud along with an
evaluation of this model in practice to a state-wide
database of actual healthcare claims with over 500
providers. The model is evaluated by applying it in
practice to actual healthcare data and having experts
review the results of the analysis. The paper
contributes to the literature by providing a roadmap
for future applications of outlier detection in
healthcare and potentially other corollary domains.
We used the domain context of Medicaid and
discussed considerations for its use in different data
contexts. We communicated the model to
stakeholders, including applying the overall process
and specific scoring methods in practice.
Through this research, we learned many insights
about antifraud efforts. Extensive healthcare subject
matter expertise is required to design analysis
techniques and interpret their results. Identifying 17
out of 360 (5%) primary dental providers for further
investigation, of which 12 of 17 (71%) have been
evaluated and deemed appropriate for formal
investigation can be considered a successful
outcome. As compared with prior comparative
success rates of roughly 10% (Major & Riedinger
2002), we see great opportunity in building upon this
model in various ways. Future research will dive
deeper, including evaluating specific outlier
techniques relevant to different types of healthcare
fraud, and look more broadly at methods and models
for storing and preserving the scoring metadata and
provenance information to allow for more automated
scoring, model adaptability, and reconstruction. With
this research we hope to both advance the state of the
art in healthcare fraud detection and prevention, as
well as materially assist tax payers and law
enforcement in confronting this important societal
challenge.
REFERENCES
Aggarwal, C. C., 2013. Outlier analysis, New York:
Springer.
Aral, K. D. et al., 2012. A prescription fraud detection
model. Computer Methods and Programs in
Biomedicine, 106(1), pp.37–46.
Bolton, R. J. & Hand, D. J., 2002. Statistical fraud
detection: A review. Statistical Science, 17(3),
pp.235–255.
Colin, C. et al., 1994. Data quality in a DRG-based
information system. International Journal for Quality
in Health Care, 6(3), pp.275–280.
Department of Health and Human Services, 1998.
Medicare A/B Reference Manual - Chapter 21 -
Benefit Integrity and Program Safeguard Contractors.
Available at: https://http://www.novitas- solutions.
com/refman/chapter-21.html [Accessed February 21,
2013].
District of New Jersey U.S. Attorneys Office, 2013. South
Jersey Doctor Admits Making Half-a-Million Dollars
in Fraud Scheme Involving Home Health Care for
Elderly Patients. Available at: http://www.fbi.gov/
newark/press-releases/2013/south-jersey-doctor-
admits-making-half-a-million-dollars-in-fraud-
scheme-involving-home-health-care-for-elderly-
patients [Accessed March 28, 2013].
District of Texas U.S. Attorneys Office, 2013. Physician
Pleads Guilty to Role in Health Care Fraud
Conspiracy. Available at: http://www.fbi.gov/dallas/
press-releases/2013/physician-pleads-guilty-to-role-in-
health-care-fraud-conspiracy [Accessed March 1,
2013].
Forgionne, G. A., Gangopadhyay, A. & Adya, M., 2000.
An intelligent data mining system to detect healthcare
fraud. In Healthcare information systems: challenges
of the new millennium. Hershey PA: IGI Global, pp.
148–169.
Hernández, M. A. & Stolfo, S. J., 1998. Real-world data is
dirty: Data cleansing and the merge/purge problem.
Data mining and knowledge discovery, 2(1), pp.9–37.
Hevner, A. R. et al., 2004. Design Science in Information
Systems Research. MIS Quarterly, 28(1), pp.75–105.
Iyengar, V. S., Hermiz, K. B. & Natarajan, R., 2013.
Computer-aided auditing of prescription drug claims.
Health Care Management Science, (July), pp.1–12.
Kelley, R. R., 2009. Where can $700 billion in waste be
cut annually from the US healthcare system? Ann
Arbor, MI: Thomson Reuters, TR-7261 10/09 LW.
Lu, F. & Boritz, J. E., 2005. Detecting fraud in health
insurance data: Learning to model incomplete
Benford’s law distributions. In Machine Learning:
ECML 2005. Springer, pp. 633–640.
Major, J. A. & Riedinger, D. R., 2002. EFD: A Hybrid
Knowledge/Statistical-Based System for the Detection
Outlier-basedHealthInsuranceFraudDetectionforU.S.MedicaidData
693