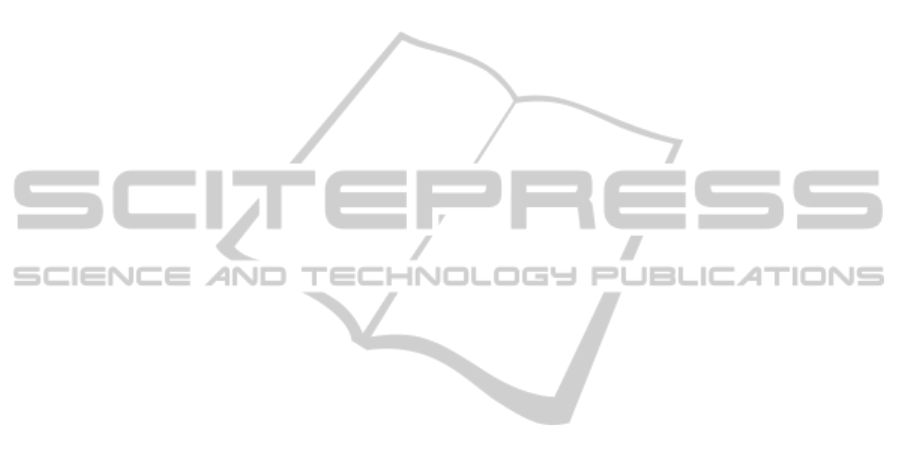
benefits for not only the focal vehicle who changes
lanes but also for the society as a whole, even if its
frequency is low.
Figure 4 (
155.0
) suggests a weak Prisoner’s
Dilemma (PD). This is confirmed by the following
facts. At P
c
= 0, NE is trapped because the defector’s
payoff is always greater than that of the cooperator.
EPO appears at P
c
= 1 because the social payoff
increases with increasing cooperation fraction,
although the effect is subtle. The same tendency
appears in Figure 7 (
211.0
), although the extent
of this dilemma seems more severe than that in
Figure 4. In Figure 7, the social payoff function does
not monotonically increase with the increase in the
cooperation fraction, as observed in Figure 4; rather,
it shows an N-character shape, in which a local peak
(much smaller than EPO at P
c
= 1) appears at a
lower cooperation fraction. This point is carefully
discussed in Sec. 3.2.
Figure 6 (
194.0
) differs slightly from the
simple PD because EPO is not observed at P
c
= 1,
although NE is trapped at P
c
= 0. At any rate, EPO is
largely inconsistent with NE since EPO, which is the
peak of social payoff, appears above P
c
= 0.5.
Therefore, we call this game structure a D-dominate
quasi-Prisoner’s Dilemma game.
Figure 8 (
244.0
) seems odd; it looks
analogous to a D-dominate quasi-PD Game (Fig. 6),
but it differs. EPO defined by the peak of social
payoff appears below P
c
= 0.5 and is relatively close
to NE found at P
c
= 0. Therefore, we call this a D-
dominate quasi-light PD game.
Figure 10 shows the effects of vehicle density on the
strength of dilemma,
, defined by Nakata et al.
(2011). Figure 10 shows that the density at severe
dilemma strength is consistent with the density
observed in the high-flux region, including the
metastable phase (Fig.1 (a)). This seems physically
plausible because, in this density region, a driver has
a strong incentive for changing lanes to exploit other
drivers and ensure his own benefit is maximized
(smaller travel time). However, when one driver
changes lanes, others might follow. Therefore, states
with high flux, say in the metastable phase, collapse
with the phase shifting to the jam phase.
4 CONCLUSIONS
For ordinal traffic flows, we have successfully
demonstrated that there are hidden social-dilemma
structures evoked by drivers’ decisions whether or
not they should change lanes. This was confirmed by
a series of numerical simulations using the revised
S-NFS cellular automaton model combined with a
lane-changing model that we developed and applied
with cyclic boundary conditions.
Interestingly, social dilemmas, as classified by
the Prisoner’s Dilemma game or its variants, were
only observed in situations of middle vehicle
density; these situations correspond to the region on
the fundamental diagram, including the metastable
phase, in which data are scattered. This seems
plausible because, when a driver is surrounded by
other vehicles, that driver has a serious incentive to
change lanes. However, if all drivers make the same
decision, social efficiency declines phenomenally
and huge traffic jams emerge. We also evaluated the
relation between dilemma strength and density of
vehicles.
ACKNOWLEDGEMENTS
This study was partially supported by a Grant-in-Aid
for Scientific Research by JSPS, awarded to Prof.
Tanimoto (#25560165), Pfizer Health Research
Foundation. We would like to express our gratitude
to these funding sources.
REFERENCES
Kerner, B. S, 2009; Introduction to Modern Traffic Flow
Theory and Control: The Long Road to Three-Phase
Traffic Theory, Springer.
Kokubo. S., Tanimoto. J., Hagishima. A., 2011; A new
Cellular Automata Model including a decelerating
damping effect to reproduce Kerner's three-phase
theory, Physica A 390(4), 561–568.
Kukida. S.; Tanimoto. J.; Hagishima. A., 2011; Analysis
of the influence of lane changing on traffic-flow
dynamics based on the cellular automaton model,
International Journal of Modern Physics C 22(3), 1–11.
Nakata, M., Yamauchi, A., Tanimoto, J., Hagishima, A.,
2010; Dilemma game structure hidden in traffic flow
at a bottleneck due to a 2 into 1 lane junction, Physica
A 389, 5353–5361.
Yamauchi, A., Tanimoto, J., Hagishima, A., Sagara, H.,
2009; Dilemma game structure observed in traffic
flow at a 2-to-1 lane junction, Physical Review E 79,
036104.
TrafficFlowSimulationDovetailedwithEvolutionaryGameTheory
519