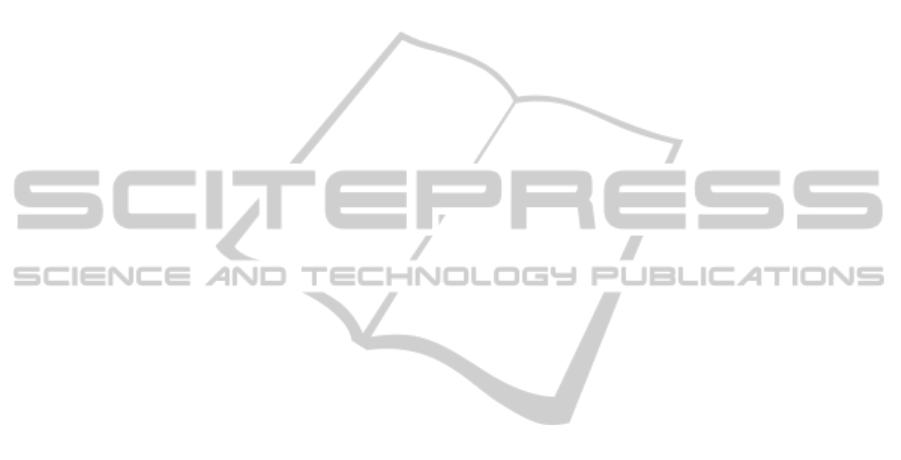
5 DISCUSSION AND
CONCLUSIONS
As an initial attempt, the analysis presented here is
rather basic and for the purpose of demonstrating the
use of Bayesian network. However, it sufficiently
shows the merits of Bayesian inference for the audits
on the correctness of the declarations.
Compared to the OLAP-based analysis, Bayesian
networks provide a lot more flexibilities in modeling
the relations between variables. Bayesian networks
allow us to update our beliefs about the conditional
probabilities of the declaration variables when new
evidence is received. This is especially useful for a
logistics company that is continuously operating and
accumulating data, as trends and changes in shipping
behavior can be monitored. Another merit of
Bayesian networks is their resemblance to the
transportation network in international trade, as the
behavior of transportation and trading routes can be
easily incorporated in the analysis.
When applying this analysis, one should note
that the inference cannot indicate whether a
declaration is incorrect, or vice versa. The analysis
result only gives an indication on the data reliability.
This can still be helpful for the auditor to direct his /
her attention to verify the most suspicious cases.
The quality of the analysis result is of course
sensitive to the choice of the threshold. Expert
knowledge and experience can be brought in for this
choice. Analytically, supervised method like
classification can be combined to complete the
“learning cycle” for this choice.
ACKNOWLEDGEMENTS
This work was supported by the EC FP7 project
CASSANDRA (Grant agreement no: 261795). We
are thankful to the reviewers’ constructive feedbacks.
REFERENCES
Caron, E. & Daniels, H. 2013, “Explanatory Business
Analytics in OLAP.” International Journal of
Business Intelligence Research (IJBIR), vol. 4, no. 3,
pp. 67–82.
Cendrowski, H, Petro, L, Martin, J & Wadecki, A 2007,
The handbook of fraud deterrence,
Choi, T. Y. & Hartley, J. L. 1996, “An exploration of
supplier selection practices across the supply chain.”
Journal of Operations Management, vol. 14, no. 4, pp.
333–343.
Christopher, M. & Lee, H. L. 2004, “Mitigating Supply
Chain Risk through Improved Confidence.”
International Journal of Physical Distribution &
Logistics Management, vol. 34, no. 5, pp. 388–396.
Feelders, A. & Daniels, HAM 2001, “A general model for
automated business diagnosis.” European Journal of
Operational Research, vol. 130, no. 3, pp. 623–637.
Hulstijn, J. & Overbeek, S. 2012, “Integrity of supply
chain visibility: Linking information to the physical
world.” Lecture Notes in Business Information
Processing, vol. 112, pp. 351–365.
Jambeiro Filho, J & Wainer, J 2007, “Using a Hierarchical
Bayesian Model to Handle High Cardinality Attributes
with Relevant Interactions in a Classification
Problem.,” in IJCAI,pp. 2504–2509.
Jensen, F. V. & Nielsen, TD 2007, Bayesian networks and
decision graphs, Springer Science+Business Media,
LLC, New York.
Klievink, B, van Stijn, E, Hesketh, D, Aldewereld, H, et al.
2012, “Enhancing Visibility in International Supply
Chains.” International Journal of Electronic
Government Research, vol. 8, no. 4, pp. 14–33.
Korb, K. B. & Nicholson, A. E. 2003, Bayesian artificial
intelligence, cRc Press.
Kumar, A. & Nagadevara, V. 2006, “Development of
hybrid classification methodology for mining skewed
data sets-a case study of indian customs data,” in
Computer Systems and Applications, 2006. IEEE
International Conference on.,pp. 584–591.
Liu, L., Daniels, H. & Hofman, W. 2013, “Detecting and
Explaining Business Exceptions for Risk Assessment.”
ICEIS 2013.
Tongzon, J. L. 2009, “Port choice and freight forwarders.”
Transportation Research Part E: Logistics and
Transportation Review, vol. 45, no. 1, pp. 186–195.
Wang, R. Y. & Strong, DM 1996, “Beyond accuracy:
What data quality means to data consumers.” J. of
Management Information Systems, vol. 12, no. 4, pp.
5–33.
Yan-hai, L. & Lin-yan, S 2005, “Study and applications of
data mining to the structure risk analysis of customs
declaration cargo,” in e-Business Engineering, 2005.
ICEBE 2005. IEEE International Conference on,pp.
761–764.
Yaqin, W. & Yuming, S. 2010, “Classification Model
Based on Association Rules in Customs Risk
Management Application,” in Intelligent System
Design and Engineering Application (ISDEA), 2010
International Conference on,pp. 436–439.
ICEIS2014-16thInternationalConferenceonEnterpriseInformationSystems
712