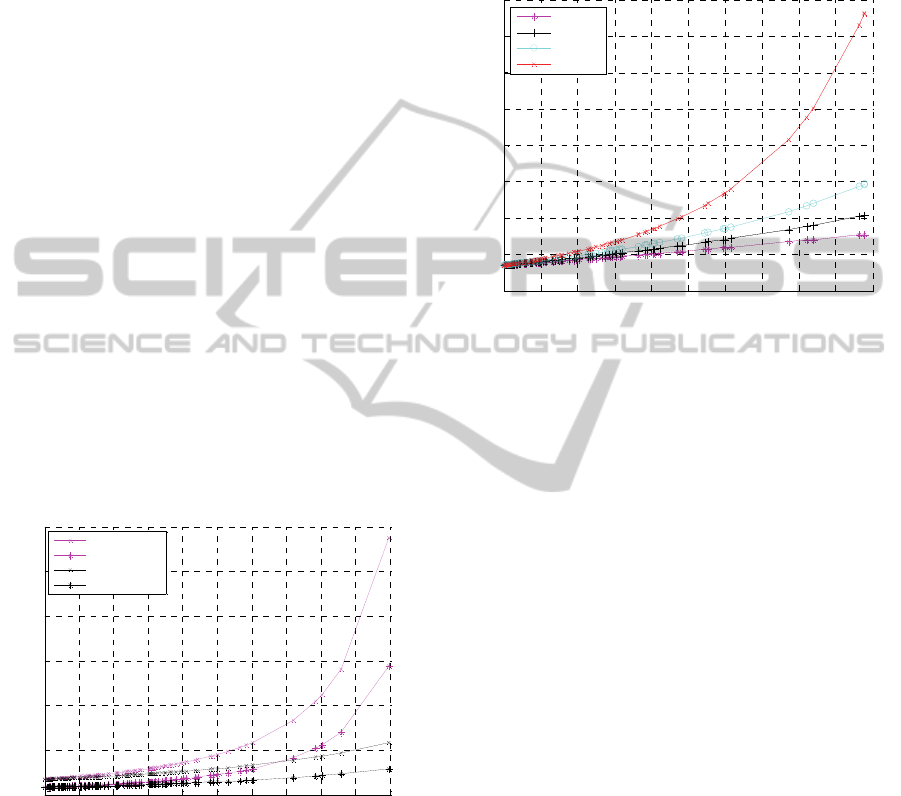
In Figure 6., we have considered the operation
for a fixed number of server and plotted the average
waiting time for exponential flow, we observe that
as the priority decreases, the waiting time increases
and the peak waiting time also increases at a much
faster rate. We have considered the 4-class,6-class,9-
class operation. We observe that the peak waiting
time drops ~70% between 9-class and 6-class and
~83% between 9-class and 4-class operation.
Through this data, we can theorize that as the
priority of the class increases, the waiting times
becomes constant for lower and higher traffic input;
hence the effect of increase of the traffic is highest
on the lowest of priority inputs and lowest on
highest of priority inputs. Thus, for the constant
traffic input, the increase of the volume of traffic has
a cascading effect on the lower priority classes, the
waiting time increase is the severest in the lowest of
priority classes, and thus the network has an upper
limit on the number of operations that can be
sustained economically. We also observe that when
the utilization of the server is the highest and the
traffic of the system approaches the peak value (~1
Erlang) the lowest of priority operations may have
such high waiting time that it may become un-
economical for the user. We must either reduce the
number of operations that can be supported or
increase the number of wavelengths that are allotted
to the MAN network.
Figure 7: Average Waiting Time (sec) versus Traffic
Intensity (rho) for 5 class for 2 and 4 server and 8 class for
2 and 4 server operation with exponential flow.
For Figure 7, we have considered the particular class
of operation, viz class 8 and class 5, for 2-server and
4-server operation. We observe that the drop in the
peak waiting time, observed across higher traffic is
approximately 49% when the number of servers is
increased, in class-8 case, whereas the effect over
the lower class (class-5) is (~50-60%). We also
observe that for lower amount of traffic (0-65%) of
peak traffic, class-8, 4-server operation performs
better than the class-5, 2-server operation. The above
operation highlight the importance of increasing the
number of servers, although their effect may vary as
the priority of the operation is increased.
Figure 8: Average Waiting Time (sec) versus Class 3
Waiting Time for 4, 5, 6, 9 class operation with
exponential flow.
The Figure 8 also proves that only a finite number of
operations can be supported on the network, here the
waiting times of class 4,5,6,9 are plotted against the
class 3 waiting traffic for a fixed number of servers.
The premise of such exercise is to find out the
effects on increase of traffic on lower and higher
traffic as well as their interdependence. We observe
that with the increase of waiting time of a lower
class traffic (class-3 in this case), there is a
cascading effect on the higher classes (for lowest of
priority operations) i.e. their waiting time increases
exponentially on the increase of traffic and thus, for
the least priority of classes, the waiting time may
become so high that the cost becomes unsustainable.
Thus, only a finite amount of classes can be
supported by the OFS network, for a fixed number
of servers.
We have observed in the above conclusion that
the difference in the peak waiting time decreases as
we increase the number of servers, so, we have to
arrive at an optimum parameter which balances the
economic consideration as well as the waiting time
of the server.
0 0.1 0.2 0.3 0.4 0.5 0.6 0.7 0.8 0.9 1
0
0.02
0.04
0.06
0.08
0.1
0.12
Rho
Average Waiting time
8-class,2 server
8-class,4 server
5-class,2 server
5-class,4 server
7 7.5 8 8.5 9 9.5 10 10.5 11 11.5 12
x 10
-3
0
0.01
0.02
0.03
0.04
0.05
0.06
0.07
0.08
Class 3 Waiting Time
Average Waiting time of other classes
4th Class
5th Class
6th Class
9th Class
MulticlassMultiserverServiceDifferentiationinOpticalFlowSwitchedNetworks
29