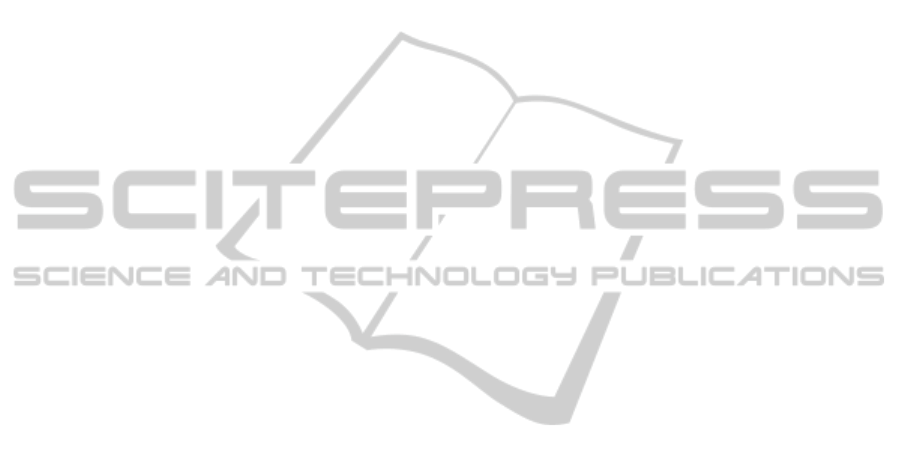
The above results show that the group
scheduling approach, based on applying the
criterion of relative direct cost and the criterion of
average orders utility, allows computing the
satisfactory schedule versions. However, one
cannot assert that any version is the best within a
given set of versions and, all the more, within a
whole possible set of versions. Moreover, when the
planning horizon in the “make-to-stock” strategy is
changed, the computed schedule versions change
substantially as well. Quality of scheduling
depends essentially on initial parameters: size of
the transport batch, the planning horizon and the
psychological coefficient.
Computations show that the order utility is
great for a small transport batch. When the batch
size increases, the order utility diminishes. For the
numeral example in Section 5, in the interval from
6 to 12 pieces there is sharp decrease of the order
utility, then utility increases again. Thus, in this
case the optimal size of the transport batch is equal
to 6 or 12.
When the planning horizon changes, the
computed versions of schedule also change
substantially. If the horizon increases, the system
automatically offers the versions with larger
groups of transport batches. Computations show
that at the horizon that is named critical, the
number of output batches for parts of any type
begin to increase sharply. This horizon value may
be considered as maximum possible for
scheduling.
Scheduling is a regular process that repeats
with certain, but not always constant cycle. For
this purpose it is convenient to use new MS Excel
sheets, where information from previous sheets
may be contained. By changing or inserting of
new data, the user can correct the previous plan or
design a new one. The proposed decision support
tool gives possibility for transition from previous
date to subsequent one without serious changes in
the scheduling methodology.
In real practice various additional constraints
may be necessary for scheduling. For example,
often it is needed to take into account the current
device wear and tear, limited storage possibilities,
general shipping terms, etc. In our opinion, it is not
reasonable to take into account all such constraints
in a single program. For each case it is necessary
to create a special program with joint efforts of the
user and the main developer. In the nearest future it
is planned to elaborate some solutions, which
correspond to listed problems.
REFERENCES
Barfod MB, Salling K.B. and Leleur S. (2011).
Composite decision support by combining cost-
benefit and multi-criteria decision analysis. Decision
Support Systems, 51(1), 167-175.
Buehlmann, U., Ragsdale, C.T. and Gfeller, B. (2000). A
spreadsheet-based decision support system for wood
panel manufacturing. Decision Support Systems,
28(3), 207-227.
Canon, L.-C. and Jeannot, E. (2011). MO-Greedy: an
extended beam-search approach for solving a multi-
criteria scheduling problem on heterogeneous
machines. IEEE International Symposium on
Parallel and Distributed Processing Workshops and
PhD Forum, 57-69.
Garcia-Sabater, J.P. , Maheut, J. and Garcia-Sabater,
J.J.(2009). A decision support system for aggregate
production planning based on MILP: A case study
from the automotive industry. In Proceedings of the
International Conference on Computers & Industrial
Engineering, 366-371, Troyes.
Fonseca, D. J., Li, L. and Chen, D.-C. (2005). A
decision support system for production planning in
just-in-time manufacturing. Asian Journal of
Information Technology, 4(6), 623-627.
Hasan, S. M., Sarker, R. and Essam, D. (2012). A
decision support system for solving job-shop
scheduling problems using genetic algorithms.
Flexible Services and Manufacturing Journal, 23(2),
137-155.
Heilala J., Montonen J., Järvinen P., Kivikunnas S.
(2010). Decision support using simulation for
customer-driven nanufacturing system design and
operations planning. In Decision Support Systems,
Advances in, 235-260, Croatia, INTECH.
Kalantari, M., Rabbani, M. and Ebadian,M. (2011). A
decision support system for order
acceptance/rejection in hybrid MRS/MTO
production systems. Applied Mathematical
Modelling, 35(3), 1363-1377.
Kargin, A.A. and Mironenko, D. C. (2009). Concept
and algorithm model of imitation and simulation for
sheet cutting at primary operation shops of MZTM
plant. Donetsk National University Herald, Series
A, Natural sciences, 1, 452-457 (in Russian).
Lee, W.J. and Lee, K.C. (1999). A meta decision support
system approach to coordinating
production/marketing decisions. Decision Support
Systems, 25(3), 239-250.
Mauergauz, Y. (2012). Objectives and constraints in
advanced planning problems with regard to scale of
production output and plan hierarchical level.
International Journal of Industrial and Systems
Engineering, 12(4), 369-393.
Mauergauz, Y. (2013). Cost-efficiency method for
production scheduling. In Proceedings of the World
Congress on Engineering 2013, 587-593, London.
Mahdavi, I., Shirazi, B. and Solimanpur, M. (2010).
Development of a simulation-based decision support
DecisionSupportToolforGroupJob-shopSchedulingProblems
405