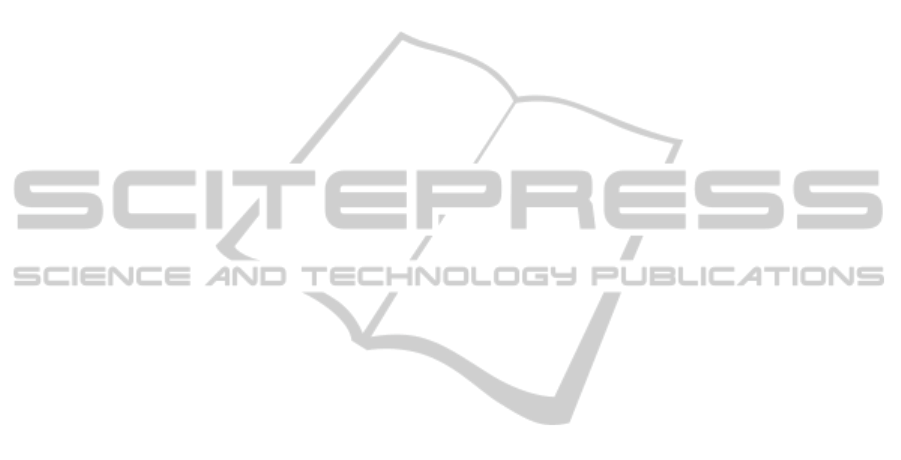
der to identify and visually highlight influencers (i.e.,
hub nodes), and influence (i.e., spread of multi-layer
peripheral nodes), represented by the opinions ex-
pressed by social media users on a given set of topics.
Results show that our approach produces aesthetically
pleasant graph layouts, by highlighting multi-layered
clusters of nodes surrounding hub nodes (the main
topics). These multi-layered peripheral node clusters
represent a visual aid to understand influence.
Our approach exploits the underlying concept of
power-law degree distribution with the metaphor of
k-shell decomposition, thus we able to visualize so-
cial networks in multi-layered, clustered peripheries
around hub-nodes, which not only preserves the graph
drawing aesthetic criteria, but also effectively rep-
resent multi-layered peripheral clusters around hub
nodes. We analysed multi-clusters, spread of multi-
layered peripheries, brand fidelity, content specificity,
and sentiment analysis through our proposed visual
framework.
Empirical testing and evaluation results show that
specificity, frequency, and retweets are mutually cor-
related, and have a significant impact on an author’s
influence and encourage us to further explore so-
cial network’s intrinsic characteristics. Although our
experiment can be repeated with data from entities
different from tourism, additional empirical work is
needed to extend testing to multiple datasets and do-
mains.
Future work will consider measures of influence
with additional parameters besides frequency of shar-
ing, content specificity and frequency of retweets. In
our current work, we are studying an achievable mea-
sure of influence through proposed visualization ap-
proach, that can be used to rank influential nodes in
social networks (Metra, 2014).
REFERENCES
Abello, J. and Queyroi, F. (2013). Fixed points of graph
peeling. In Proceedings of the 2013 IEEE/ACM Inter-
national Conference on Advances in Social Networks
Analysis and Mining, pages 256–263. ACM.
Alvarez-Hamelin, J. I., Dall’Asta, L., Barrat, A., and
Vespignani, A. (2006). Large scale networks finger-
printing and visualization using the k-core decompo-
sition. Advances in neural information processing sys-
tems, 18:41.
Andersen, R., Chung, F., and Lu, L. (2004). Drawing power
law graphs using local/global decomposition. Twelfth
Annual Symposium on Graph Drawing.
Andersen, R., Chung, F., and Lu, L. (2007). Drawing power
law graphs using a local global decomposition. Algo-
rithmica, 47(4):397.
Anger, I. and Kittl, C. (2011). Measuring influence on twit-
ter. In Proceedings of the 11th International Con-
ference on Knowledge Management and Knowledge
Technologies, page 31. ACM.
Anholt, S. (2006). Competitive identity: The new brand
management for nations, cities and regions. Palgrave
Macmillan.
Arbuckle, J. L. (2011). Ibm spss amos 20 users guide. Amos
Development Corporation, SPSS Inc.
Asur, S., Huberman, B. A., Szabo, G., and Wang, C. (2011).
Trends in social media: Persistence and decay. In
ICWSM.
Bagozzi, R. P. and Fornell, C. (1982). Theoretical concepts,
measurements, and meaning. A second generation of
multivariate analysis, 2(2):5–23.
Bakshy, E., Hofman, J. M., Mason, W. A., and Watts, D. J.
(2011). Everyone’s an influencer: quantifying influ-
ence on twitter. In Proceedings of the fourth ACM
international conference on Web search and data min-
ing, pages 65–74. ACM.
Barbagallo, D. (2010). A data quality based methodology to
improve sentiment analyses. PhD thesis, Politecnico
di Milano, Milan, Italy.
Barbagallo, D., Bruni, L., Francalanci, C., and Giacomazzi,
P. (2012). An empirical study on the relationship be-
tween twitter sentiment and influence in the tourism
domain. In Information and Communication Tech-
nologies in Tourism 2012, pages 506–516. Springer.
Benevenuto, F., Cha, M., Gummadi, K., and Haddadi, H.
(2010). Measuring user influence in twitter: The mil-
lion follower fallacy. In International AAAI Confer-
ence on Weblogs and Social (ICWSM10), pages pp.
10–17.
Bigonha, C., Cardoso, T. N., Moro, M. M., Gonc¸alves,
M. A., and Almeida, V. A. (2012). Sentiment-based
influence detection on twitter. Journal of the Brazil-
ian Computer Society, 18(3):169–183.
Blitzer, J., Dredze, M., and Pereira, F. (2007). Biographies,
bollywood, boom-boxes and blenders: Domain adap-
tation for sentiment classification. In ACL, volume 7,
pages 440–447.
Boutin, F., Thievre, J., and Hascoet, M. (2006). Focus-
based filtering + clustering technique for power-law
networks with small world phenomenon. SPIE-IS & T
Electronic Imaging, 6060.
Boyd, D., Golde, S., and Lotan, G. (2010). Tweet, tweet,
retweet: Conversational aspects of retweeting on twit-
ter. IEEE, pages pp. 1–10.
Bruni, L. (2010). A methodology framework to understand
and leverage the impact of content on social media
influence. PhD thesis, Politecnico di Milano, Milan,
Italy.
Bruni, L., Francalanci, C., Giacomazzi, P., Merlo, F., and
Poli, A. (2013). The relationship among volumes,
specificity, and influence of social media information.
In Proceedings of International Conference on Infor-
mation Systems.
Bullock, H. E., Harlow, L. L., and Mulaik, S. A. (1994).
Causation issues in structural equation modeling re-
DATA2014-3rdInternationalConferenceonDataManagementTechnologiesandApplications
328