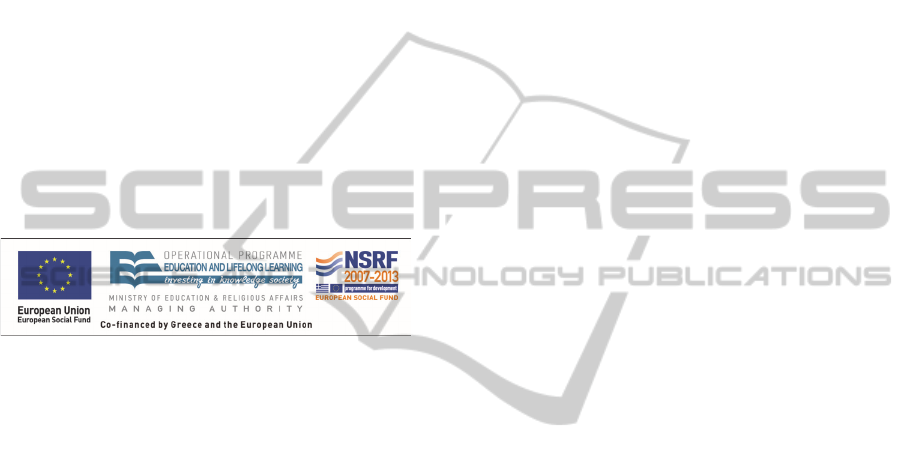
In our opinion the above results statistically
show that recommendation systems can leverage
much more from the appliance of various
methodologies which are working in tandem in a
heuristic fashion, as opposed to single-minded
approaches, however smart or well implemented,
when applied autonomously. In essence,
collaborative approaches work far better when
combined with content-based ones (i.e. k/w
extraction techniques).
For the future we are planning to improve each
of the presented methodologies in order to fine tune
them and achieve even better results. Furthermore,
we intent to extend our experimentation to a larger
user database and corpus statistically analyzing the
results that we shall obtain to a greater extend.
ACKNOWLEDGEMENTS
This research has been co-financed by the European
Union (European Social Fund – ESF) and Greek
national funds through the Operational Program
"Education and Lifelong Learning" of the National
Strategic Reference Framework (NSRF) - Research
Funding Program: Heracleitus II. Investing in
knowledge society through the European Social
Fund.
REFERENCES
Bouras, C., & Tsogkas, V., 2008. Improving text
summarization using noun retrieval techniques. In
Knowledge-Based Intelligent Information and
Engineering Systems (pp. 593-600).
Bouras, C., & Tsogkas, V., 2010. W-kmeans: clustering
news articles using WordNet. In Knowledge-Based
and Intelligent Information and Engineering Systems
(pp. 379-388).
Bouras, C., & Tsogkas, V., 2011. Clustering user
preferences using W-kmeans. In Signal-Image
Technology and Internet-Based Systems (SITIS), 2011
Seventh International Conference on (pp. 75-82).
IEEE.
Bouras, C., & Tsogkas, V. Enhancing news articles
clustering using word n-grams. 2013. 2nd Intenational
Conference on Data Management Technologies and
Applications, Reykjavvk, Iceland
Bianco, A., Mardente, G., Mellia, M., Munafo, M., &
Muscariello, L., 2005. Web user session
characterization via clustering techniques. In Global
Telecommunications Conference, 2005.
GLOBECOM'05. IEEE (Vol. 2, pp. 6-pp). IEEE.
Hand, D. J., Mannila, H., & Smyth, P., 2001. Principles of
data mining. MIT press.
Kim, B. M., Li, Q., Park, C. S., Kim, S. G., & Kim, J. Y.
(2006). A new approach for combining content-based
and collaborative filters. Journal of Intelligent
Information Systems, 27(1), 79-91.
Lops, P., Degemmis, M., & Semeraro, G., 2007.
Improving social filtering techniques through
WordNet-Based user profiles. In User Modeling 2007
(pp. 268-277).
Ntoutsi, E., Stefanidis, K., Nørvåg, K., & Kriegel, H. P.,
2012, Fast group recommendations by applying user
clustering. In Conceptual Modeling (pp. 126-140).
Moore, R., Lopes, J., 1999. Paper templates. In
TEMPLATE’06, 1st International Conference on
Template Production. SCITEPRESS.
Pazzani, M. J., & Billsus, D., 2007. Content-based
recommendation systems. In The adaptive web (pp.
325-341).
Smith, J., 1998. The book, The publishing company.
London, 2
nd
edition.
Tang, N., & Vemuri, V. R., 2005. User-interest-based
document filtering via semi-supervised clustering. In
Foundations of Intelligent Systems (pp. 573-582).
White, R. W., Chu, W., Hassan, A., He, X., Song, Y., &
Wang, H., 2013. Enhancing personalized search by
mining and modeling task behavior. In Proceedings of
the 22nd international conference on World Wide Web
(pp. 1411-1420). International World Wide Web
Conferences Steering Committee.
Yu, K., Schwaighofer, A., & Tresp, V. (2002, August).
Collaborative ensemble learning: Combining
collaborative and content-based information filtering
via hierarchical Bayes. In Proceedings of the
Nineteenth conference on Uncertainty in Artificial
Intelligence (pp. 616-623).
DATA2014-3rdInternationalConferenceonDataManagementTechnologiesandApplications
40