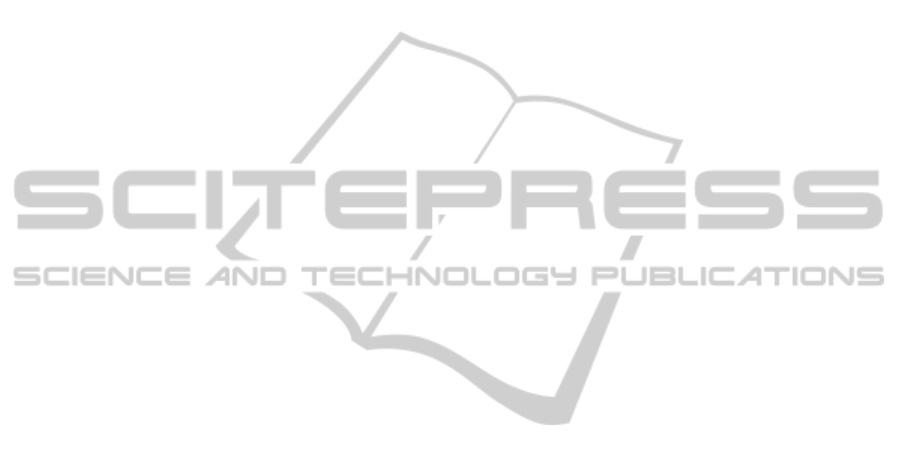
demonstrated using a well known object tracking
problem.
As the non adaptive GHF reportedly excels other
non adaptive sigma point filters like UKF and CDF,
the performance of the proposed adaptive GHF has
been compared with its non adaptive version.
In the absence of analytical proof of
convergence, each adaptive nonlinear filter,
including the proposed one, are to be thoroughly
evaluated with the help of extensive simulation
studies or real time experiments in several fields of
application before such filtering techniques may be
widely applied in practice with confidence.
However, the proposed filter may be
recommended for state and parameter estimation of
nonlinear systems because of its improved
estimation performance, good convergence, simple
adaptation rule, capacity to accommodate wide
uncertainty in the initial choice of measurement
noise covariance.
ACKNOWLEDGEMENTS
The first two authors acknowledge Council of
Scientific and Industrial Research, New Delhi, India
for financial support and express their gratitude to
the Centre for Knowledge Based System, Jadavpur
University, Kolkata, India for infrastructural
support.
REFERENCES
Simon, D., 2006. Optimal State Estimation: Kalman, H
Infinity, and Nonlinear Approaches, John Wilely &
Sons. New Jersey, 1
st
edition.
Lefebvre, T, Bruyninckx, H., Schutter, J., 2004. Kalman
filters for non-linear systems: a comparison of
performance. In International journal of Control,
77(7), 639-653.
Ito, K., & Xiong, K., 2000. Gaussian filters for nonlinear
filtering problems. In IEEE Transactions on Automatic
Control, 45(5), 910-927.
Arasaratnam, I., Haykin, S., Elliott, R. J., 2007. Discrete-
time nonlinear filtering algorithms using Gauss–
Hermite quadrature. In Proceedings of the IEEE,
95(5), 953-977.
Mehra, R. K., 1972. Approaches to adaptive filtering. In
IEEE Transactions on Automatic Control, 17(5), 693-
698.
Maybeck, P. S., 1982. Stochastic models, estimation, and
control (Vol. 2). Academic Press. New York, 1
st
edition.
Myers, K., Tapley, B. D., 1976. Adaptive sequential
estimation with unknown noise statistics. In IEEE
Transactions on Automatic Control, 21(4), 520-523.
Busse, F. D., How, J., Simpson, J., 2003. Demonstration
of adaptive extended Kalman filter for low earth orbit
formation estimation using CDGPS. In Journal of the
Insitute of. Navigation, 50(2), 79-93.
Das, M., Sadhu, S., Ghoshal, T. K., 2013. An Adaptive
Sigma Point Filter for Nonlinear Filtering Problems.
In International Journal of Electrical, Electronics and
Computer Engineering, 2(2), 13-19.
Karlgaard, C. D., Schaub, H., 2011. Adaptive nonlinear
Huber-based navigation for rendezvous in elliptical
orbit. In Journal of Guidance, Control, and Dynamics,
34(2), 388-402.
Hajiyev, C., Soken, H. E., 2014. Robust adaptive
unscented Kalman filter for attitude estimation of pico
satellites. In International Journal of Adaptive Control
and Signal Processing, 28(2), 107-120.
Dey, A., Sadhu. S., Ghoshal, T. K., 2014. Adaptive Gauss
Hermite Filter for Parameter Varying Nonlinear
Systems. Accepted for presentation in International
Conference on Signal Processing and Communication.
Sadhu, S., Srinivasan, M., Bhaumik, S., Ghoshal, T. K.
2007. Central difference formulation of risk-sensitive
filter. IEEE Signal Processing Letters,14(6), 421-424.
Norgaard, M., Poulsen, N. K., Ravn, O., 2000. New
developments in state estimation for nonlinear
systems. In Automatica, 36(11), 1627-1638.
Athans, M., Wishner, R. P., Bertolini, A., 1968.
Suboptimal state estimation for continuous-time
nonlinear systems from discrete noisy measurements.
In IEEE Transactions on Automatic Control, 13(5),
504-514.
Ristic, B., Farina, A., Benvenuti, D., Arulampalam, M. S.,
2003. Performance bounds and comparison of
nonlinear filters for tracking a ballistic object on re-
entry. In IEE Proceedings Radar, Sonar and
Navigation, 150(2), 65-70.
AdaptiveGaussHermiteFilterforParameterandStateEstimationofNonlinearSystems
589