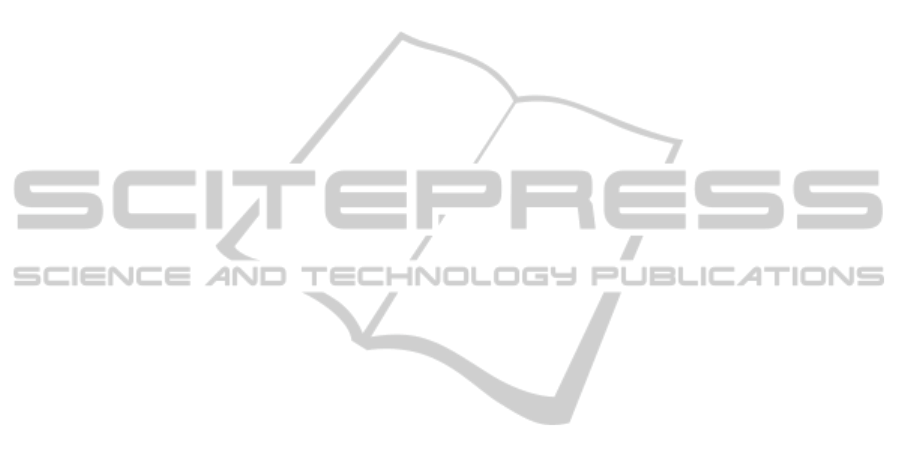
sult of
ROR
is given as 100.000 % with lowest
RPR
of 1. Not that, the average increase in performance
between the unimodal open/closed set identification
system and the multimodal open/closed set identifica-
tion system is 100 %.
5.2.3 Comparison Study
This section is dedicated to compare the performance
of the best open/closed set identification systems de-
scribed above, this can be done by showing the
ROC
curves for the open set identification using unimodal
and multimodal systems (see Fig. 7.(b)). As can be
seen by comparing these results, the accuracy of the
system using Green spectrum and the system based
on the fusion of all spectrum types (both based on the
fusion of the
GF
based sub-system and
CT
based sub-
system) is very much increased and can achieve ze-
roEER. Also, the Cumulative Match Curves (
CMC
)
comparing all best closed set identification systems
are plotted in Fig. 7.(c). From this figure, always,
Green spectrum and the system based on the fusion
of all spectrum types (both based on the fusion of the
GF
based sub-system and
CT
based sub-system) give
the best accuracy (
ROR
= 100 %). Through an anal-
ysis of the previous results, it can be observed that
in general the performance of the unimodal system is
significantly improved by using the fusion of several
spectrum types. In addition, experimentsalso demon-
strate that fusion of the two algorithms performs bet-
ter results.
6 CONCLUSION AND FURTHER
WORK
The objective of this work is to contribute to the
multimodal identification by the use of data fusion
technique. Two different sub-systems derived from
each spectrum were used in this study. Fusion of the
two proposed unimodal sub-systems is performed at
the matching score level to generate a fused match-
ing score which is used for recognizing a palm-
print image. Feature extraction process use both
GF
and
CT
methods. The experimental results, ob-
tained on a database of 400 persons, show a very
high open/closed set identification accuracy. They
also demonstrate that combining different spectrum
types or different algorithms does significantly re-
duce the accuracy of the system. In addition, our
tests show that the multimodal system provides better
open/closed set identification accuracy than the best
unimodal systems. For further improvement, our fu-
ture work will project to use other biometric modali-
ties (Face and Iris) as well as the use of other fusion
level like feature and decision levels. Also we will
focus on the performance evaluation in both phases
(verification and identification) by using a large size
database.
REFERENCES
A. Noore, R. S. and Vatsa, M. (2007). Robust memory-
efficient data level information fusion of multi-modal
biometric images. In Information Fusion. Vol. 8, No.
4, pp. 337-346.
Angel Serrano, Isaac Martyn de Diego, C. C. E. C.
(2010). Recent advances in face biometrics with gabor
wavelets: A review. In Pattern Recognition Letters.
Vol 31, pp. 372-381.
Anil Jain, Karthik Nandakumar, A. R. (2005). Score nor-
malization in multimodal biometric systems. In Pat-
tern Recognition. Vol. 38, pp. 2270-2285.
Cui, J.-R. (2012). Multispectral palmprint recognition us-
ing image based linear discriminant analysis. In In-
ternational Journal of Biometrics. Vol. 4, No. 2, pp.
106-115.
D. Zhang, V. Kanhangad, N. L. and Kumar, A. (2010). Ro-
bust palmprint verification using 2d and 3d features.
In Pattern Recognition. Vol 43, No. 1, pp. 358-368.
DavidZhang, Zhenhua Guo, G. L. L. Z. and Zuo, W. (2010).
An online system of multispectral palmprint verifica-
tion. In IEEE Transactions on Instrumentation and
Measurement. Vol. 59, No. 2,pp. 480-490.
Harun Uguz, Ahmet Arslan, I. T. (2007). A biomedical
system based on hidden markov model for diagnosis
of the heart valve diseases. In Pattern Recognition
Letters. Vol 28, pp. 395-404.
Jinrong Cui, Y. X. (2011). Three dimensional palmprint
recognition using linear discriminant analysis method.
In International Conference on Innovations in Bio-
inspired Computing and Applications. pp.107-111.
PolyU. The hong kong polytechnic university multispectral
palmprint database. www.comp.polyu.edu.hk/ bio-
metrics/MultispectralPalmprint/MSP.htm.
Singh, A. P. and Mishra, A. (2011). Image de-noising using
contoulets (a comparative study with wavelets). In Int.
J. Advanced Networking and Applications. Vol. 03, Is-
sue 03, pp. 1210-1214.
Xian-Qian Wu, Kuan-Quan Wang, D. Z. (2003). Palmprint
recognition using fishers linear discruminant. In Inter-
national Conference on Machine Learning and Cybe-
medcs.
RobustMultispectralPalmprintIdentificationSystembyJointlyUsingContourletDecomposition&GaborFilterResponse
197