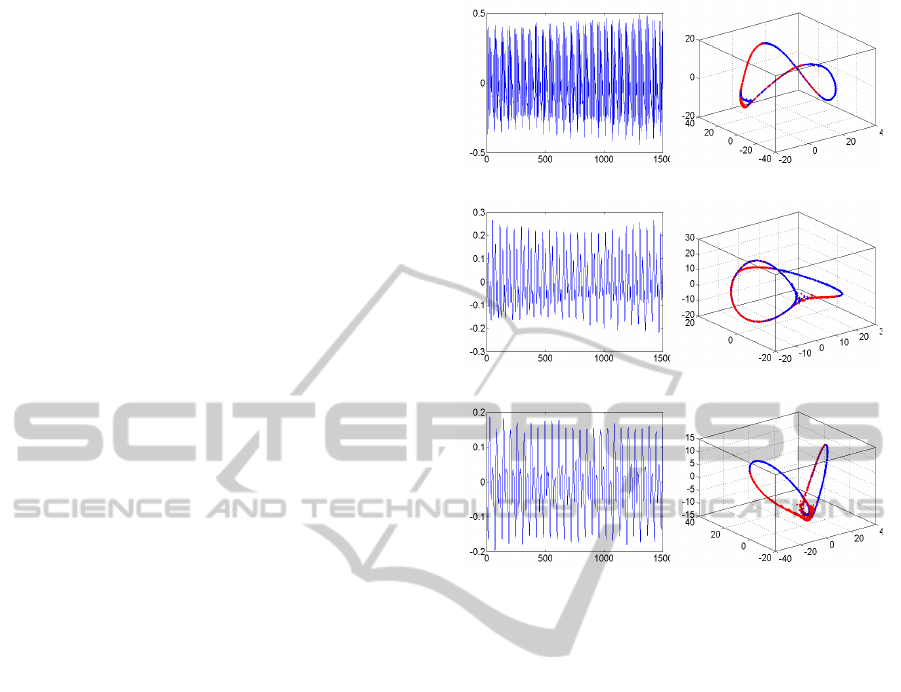
cello sounds, and their embeddings are composed of
two circles bounded. A close look at the figures
implies the number of circular shapes in the
embedding geometry is likely to be related to that of
dominant frequency components such as formants of
the waveforms. The waveforms are composed of
two dominant formants. The waveforms of violin
sounds, however, are more dynamic, i.e., have
couples of dominant frequency components,
compared with those of flutes, and are expected to
have more complicated geometric structures, as
shown in Fig. 4-(b). Indeed, the statistical
distribution of the patch sets extracted from the
different segments of the waveform varies according
to their spectral variations. For this reason, patch
sets, even though they are extracted from the same
waveform, may have quite different-looking
embedding. We get the similar results with the cello
sounds, which are displayed in Fig. 4-(c).
It is shown in Fig. 5 some examples of commute
time embedding of the patch sets extracted from the
segments of vowel sounds [a:], [o:] and [u;]. As
expected from the previous results, we observe the
embedding geometries similar to those of
instrumental sounds. The results given above
strongly support our earlier assertion that the
intrinsic geometries for the given waveform signals
can be generated using the graph Laplacian based
manifold embedding.
5 CONCLUSIONS
In this paper, we have explored the use of commute
time embedding for the purpose of transforming the
segments of some waveforms into their intrinsic
geometries. The embeddings corresponding to the
patch sets extracted from the dynamic regions of the
signals are scattered around some curves. We can
reduce such scatterings by smoothing the signals
from which patch sets are extracted, or increasing
the number of patches in the patch set. As long as
the segments of the waveforms are smooth enough
for the commute times between pairs of patches to
be densely distributed, it can be asserted that
commute time embedding generates their own
intrinsic geometries corresponding to the waveforms
on the embedding subspace. As a future research, we
would like to explore its application to pattern
classification or speech recognition in a geometric
way.
(a)
(b)
(c)
Figure 5: Commute time embedding results of the vowel
segments. (a) [a:], (b) [o:], (c) [u:].
REFERENCES
Belkin, M., Niyogi, P., 2003. Laplacian eigenmaps for
dimensionality reduction and data representation.
Neural Computation15(6), 1373-1396.
Brito, M., Chavez, E., Quiroz, A., Yukich, J., 1997.
Connectivity of the mutual k-nearest-neighbor graph in
clustering and outlier detection. Statistics and Probability
Letter.
Qiu, H., Hancock, E. R., 2007. Clustering and embedding
using commute times. IEEE Trans. PAMI, Vol. 29, No.
11, 1873-1890.
Roweis, S. T., Saul, L. K., 2000. Nonlinear dimensionality
reduction by locally linear embedding. Science
Vol.290, 2323-2326.
Taylor, K. M., 2011. The geometry of signal and image
patch-sets. PhD Thesis, University of Colorado,
Boulder, Dept. of Applied Mathematics.
Tenenbaum, J. B., deSilva, V., Langford, J. C., 2000. A
global geometric framework for nonlinear
dimensionality reduction. Science, Vol. 290, 2319-
2323.
von Luxburg, U., Radl, A., Hein, M., 2010. Getting lost in
space: Large sample analysis of the commute distance.
Neural Information Processing Systems.
ManifoldEmbeddingbasedVisualizationofSignals
189